Application of Bayesian network structure learning to identify causal variant SNPs from resequencing data
BMC proceedings(2011)
摘要
Using single-nucleotide polymorphism (SNP) genotypes from the 1000 Genomes Project pilot3 data provided for Genetic Analysis Workshop 17 (GAW17), we applied Bayesian network structure learning (BNSL) to identify potential causal SNPs associated with the Affected phenotype. We focus on the setting in which target genes that harbor causal variants have already been chosen for resequencing; the goal was to detect true causal SNPs from among the measured variants in these genes. Examining all available SNPs in the known causal genes, BNSL produced a Bayesian network from which subsets of SNPs connected to the Affected outcome were identified and measured for statistical significance using the hypergeometric distribution. The exploratory phase of analysis for pooled replicates sometimes identified a set of involved SNPs that contained more true causal SNPs than expected by chance in the Asian population. Analyses of single replicates gave inconsistent results. No nominally significant results were found in analyses of African or European populations. Overall, the method was not able to identify sets of involved SNPs that included a higher proportion of true causal SNPs than expected by chance alone. We conclude that this method, as currently applied, is not effective for identifying causal SNPs that follow the simulation model for the GAW17 data set, which includes many rare causal SNPs.
更多查看译文
关键词
Bayesian Network,Hypergeometric Distribution,Exploratory Phase,Logistic Regression Result,Causal SNPs
AI 理解论文
溯源树
样例
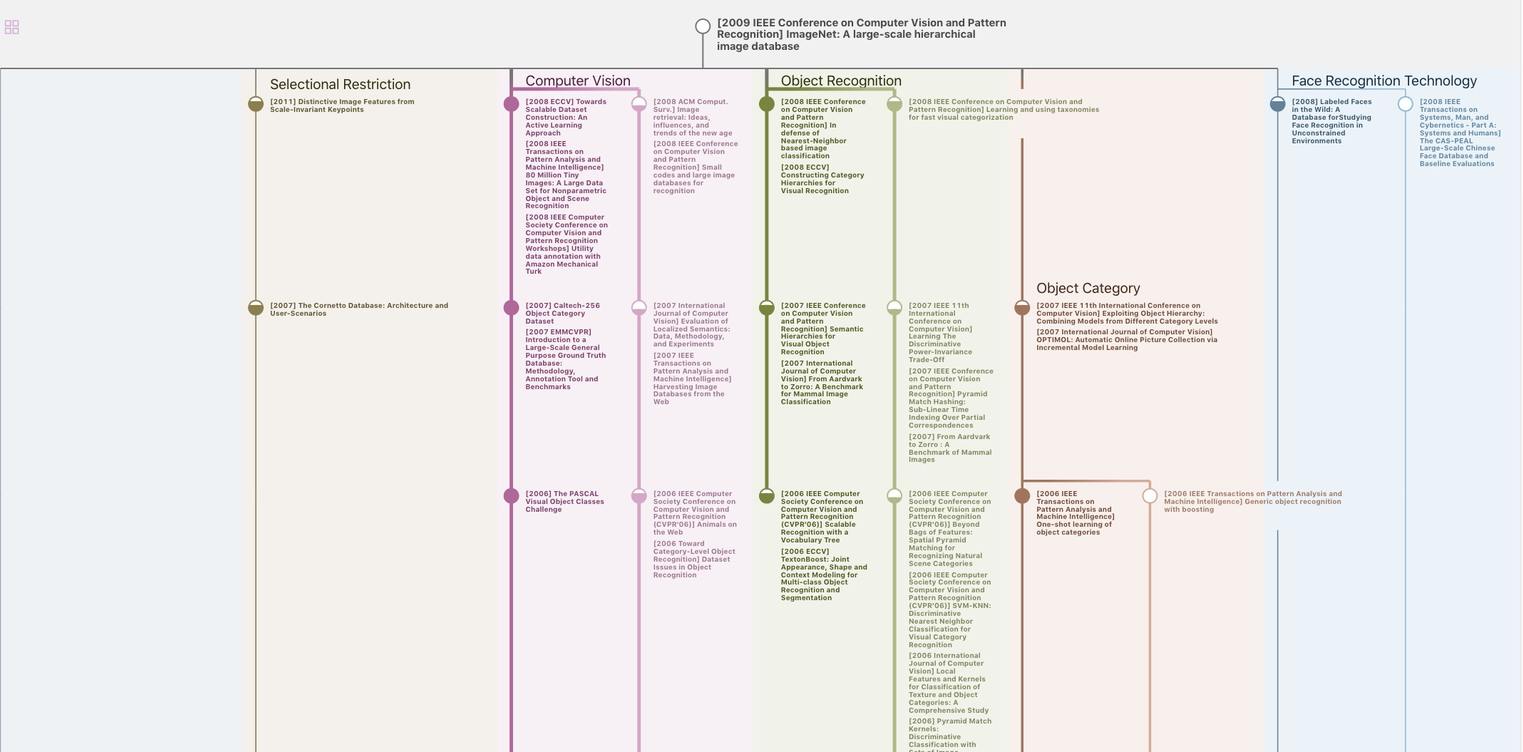
生成溯源树,研究论文发展脉络
Chat Paper
正在生成论文摘要