Strategy for minimizing between-study variation of large-scale phenotypic experiments using multivariate analysis.
ANALYTICAL CHEMISTRY(2012)
摘要
We have developed a multistep strategy that integrates data from several large-scale experiments that suffer from systematic between experiment variation. This strategy removes such variation that would otherwise mask differences of interest. It was applied to the evaluation of wood chemical analysis of 736 hybrid aspen trees: wild-type controls and transgenic trees potentially involved in wood formation. The trees were grown in four different greenhouse experiments imposing significant variation between experiments. Pyrolysis coupled to gas chromatography/mass spectrometry (Py-GC/MS) was used as a high throughput-screening platform for fingerprinting of wood chemotype. Our proposed strategy includes quality control, outlier detection, gene specific classification, and consensus analysis. The orthogonal projections to latent structures discriminant analysis (OPLS-DA) method was used to generate the consensus chemotype profiles for each transgenic line. These were thereafter compiled to generate a global dataset. Multivariate analysis and cluster analysis techniques revealed a drastic reduction in between-experiment variation that enabled a global analysis of all transgenic lines from the four independent experiments. Information from in-depth analysis of specific transgenic lines and independent peak identification validated our proposed strategy.
更多查看译文
关键词
analytical chemistry
AI 理解论文
溯源树
样例
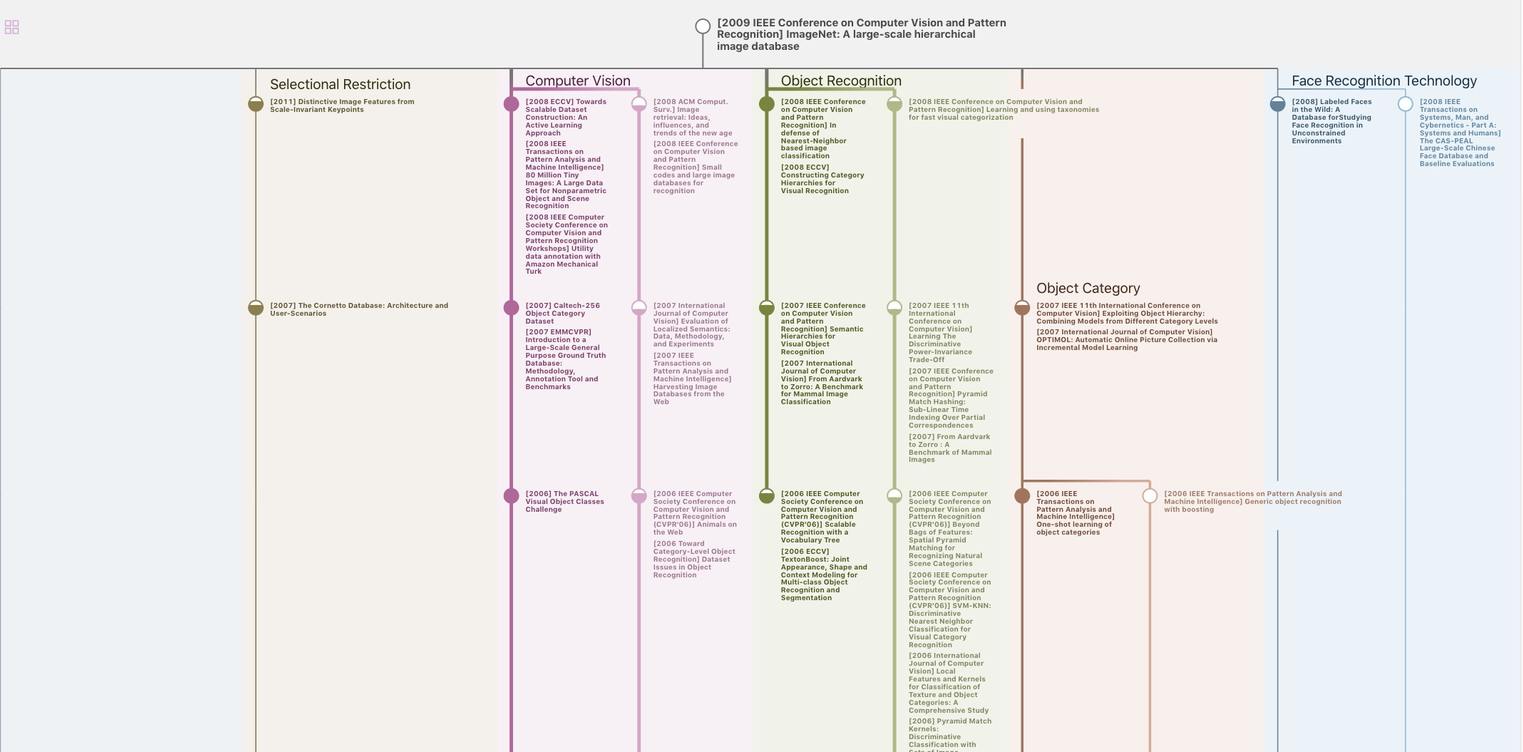
生成溯源树,研究论文发展脉络
Chat Paper
正在生成论文摘要