Predicting Diverse and Plausible State Foresight For Robotic Pushing Tasks
semanticscholar(2010)
摘要
Given an environment, humans are able to hallucinate diverse and plausible locations for an object to exist. Is it possible to let a robot learn such hallucination ability as well? If this can be carried out reliably, the robot could use this ability to generate the plausible state foresight just by observing the environment, and potentially leverage the state foresight for planning. In this paper, we study this problem of predicting diverse and plausible state foresight given an environment, which can be categorized as a conditional multimodal prediction task. Many existing approaches leverages Variational Auto-Encoder (VAE) [7] in various vision applications. However, we notice that even though these previous methods have shown they can generate multimodal results, none of them has shown that they can provide a good coverage of solution space for different conditional input, which is necessary for our application. Thus, we propose a novel two-stage model that first learns to unfold solution space of a canonical conditional environment input, and then learns to deform the solution space into an arbitrary environment. Our experiments show that our propose method outperform existing strong baselines in terms of mode coverage and plausibility. Finally, we demonstrate that our predicted state foresight can be used for planning robotic manipulation successfully.
更多查看译文
AI 理解论文
溯源树
样例
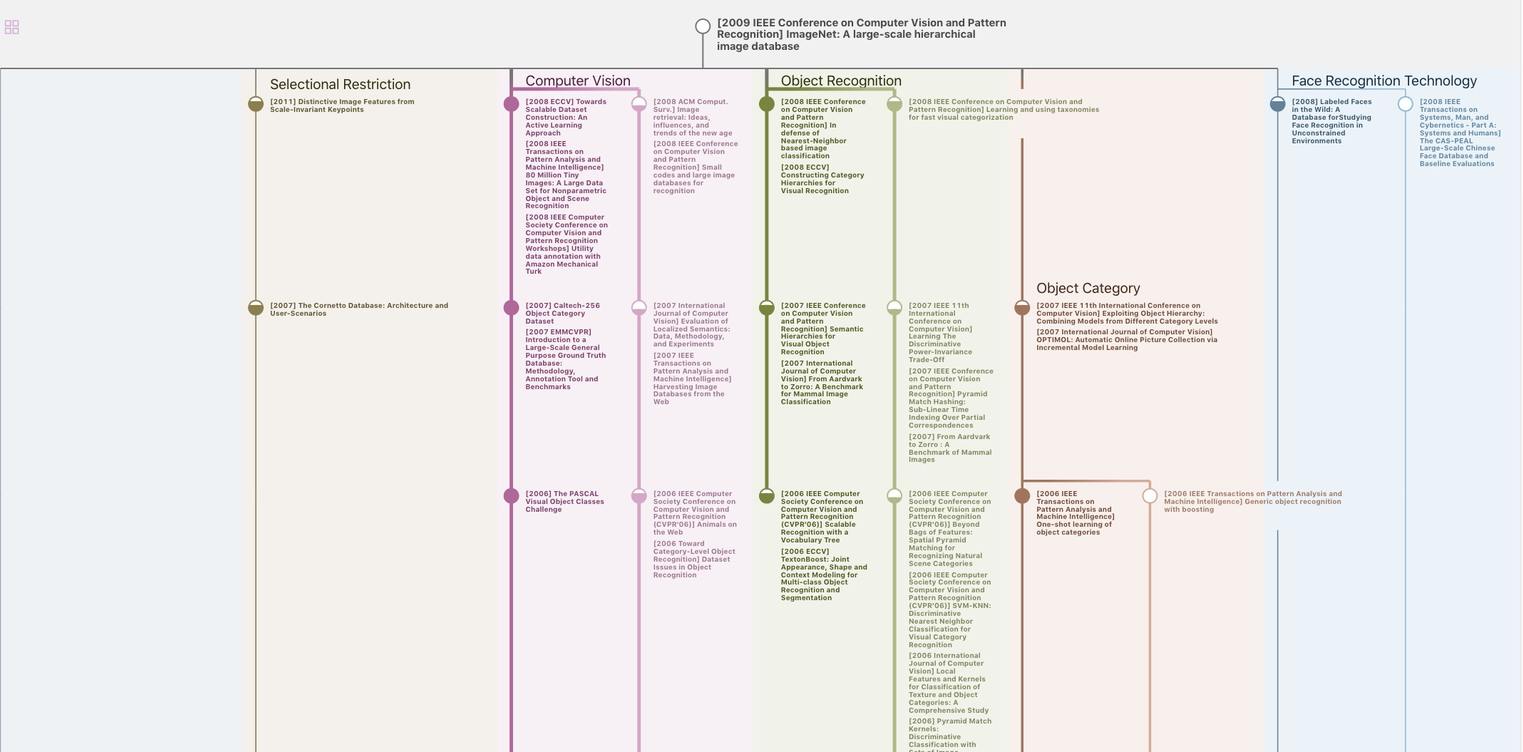
生成溯源树,研究论文发展脉络
Chat Paper
正在生成论文摘要