Iterative Reconstruction Of High-Dimensional Gaussian Graphical Models Based On A New Method To Estimate Partial Correlations Under Constraints
PLOS ONE(2013)
摘要
In the context of Gaussian Graphical Models (GGMs) with high-dimensional small sample data, we present a simple procedure, called PACOSE - standing for PArtial COrrelation SElection - to estimate partial correlations under the constraint that some of them are strictly zero. This method can also be extended to covariance selection. If the goal is to estimate a GGM, our new procedure can be applied to re-estimate the partial correlations after a first graph has been estimated in the hope to improve the estimation of non-zero coefficients. This iterated version of PACOSE is called iPACOSE. In a simulation study, we compare PACOSE to existing methods and show that the re-estimated partial correlation coefficients may be closer to the real values in important cases. Plus, we show on simulated and real data that iPACOSE shows very interesting properties with regards to sensitivity, positive predictive value and stability.
更多查看译文
关键词
medicine,chemistry,physics,biology,engineering
AI 理解论文
溯源树
样例
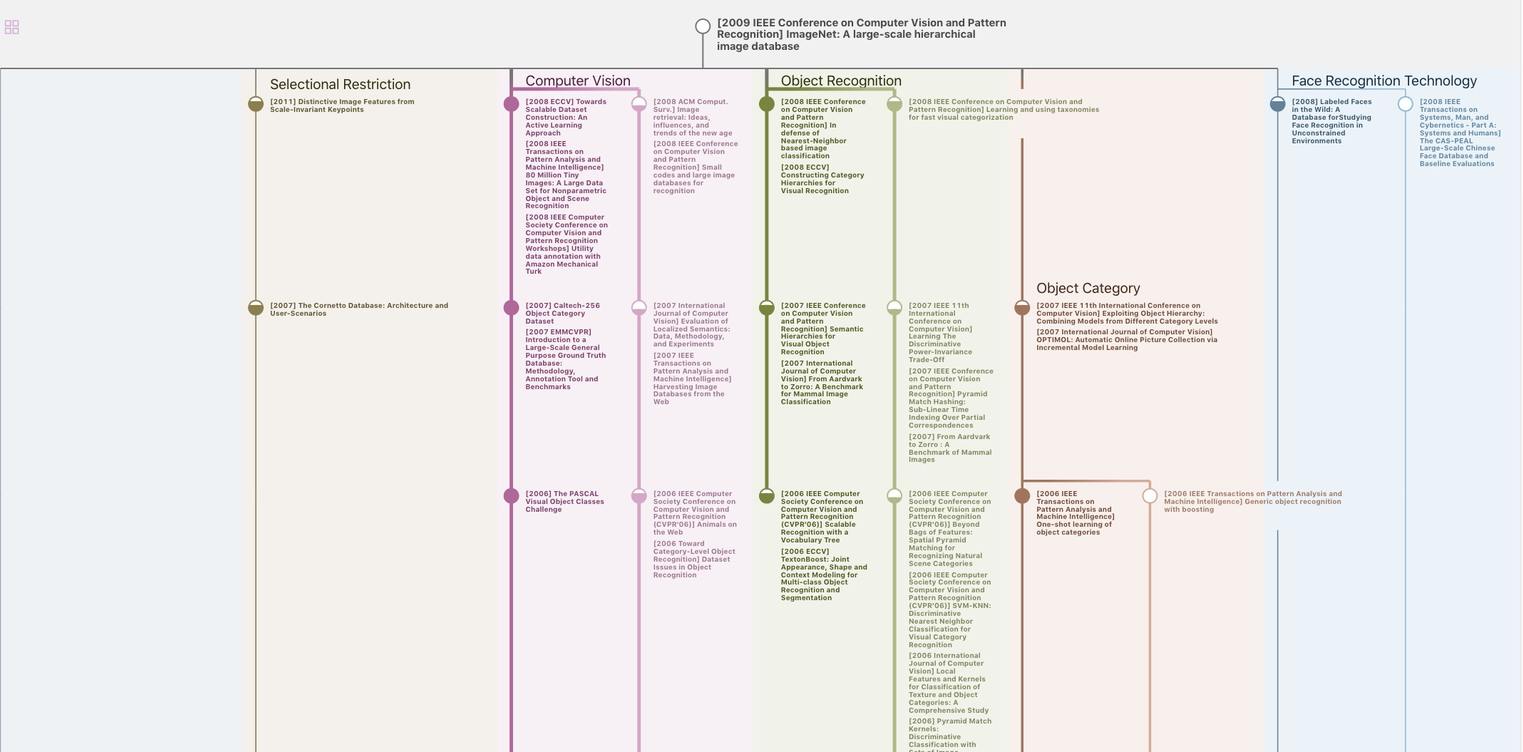
生成溯源树,研究论文发展脉络
Chat Paper
正在生成论文摘要