Computation Offloading Via Multi-Agent Deep Reinforcement Learning in Aerial Hierarchical Edge Computing Systems
IEEE Transactions on Network Science and Engineering(2024)
摘要
The exponential growth of Internet of Things (IoT) devices and emerging applications have significantly increased the requirements for ubiquitous connectivity and efficient computing paradigms. Traditional terrestrial edge computing architectures cannot provide massive IoT connectivity worldwide. In this paper, we propose an aerial hierarchical mobile edge computing system composed of high-altitude platforms (HAPs) and unmanned aerial vehicles (UAVs). In particular, we consider non-divisible tasks and formulate a task offloading problem to minimize the long-term processing cost of tasks while satisfying the queueing mechanism in the offloading procedure and processing procedure of tasks. We propose a multi-agent deep reinforcement learning (DRL) based computation offloading algorithm in which each device can make its offloading decision according to local observations. Due to the limited computing resources of UAVs, high task loads of UAVs will increase the ratio of abandoning offloaded tasks. To increase the success ratio of completing tasks, the convolutional LSTM (ConvLSTM) network is utilized to estimate the future task loads of UAVs. In addition, a prioritized experience replay (PER) method is proposed to increase the convergence speed and improve the training stability. The experimental results demonstrate that the proposed computation offloading algorithm outperforms other benchmark methods.
更多查看译文
关键词
Aerial computing,mobile edge computing,deep reinforcement learning,computation offloading
AI 理解论文
溯源树
样例
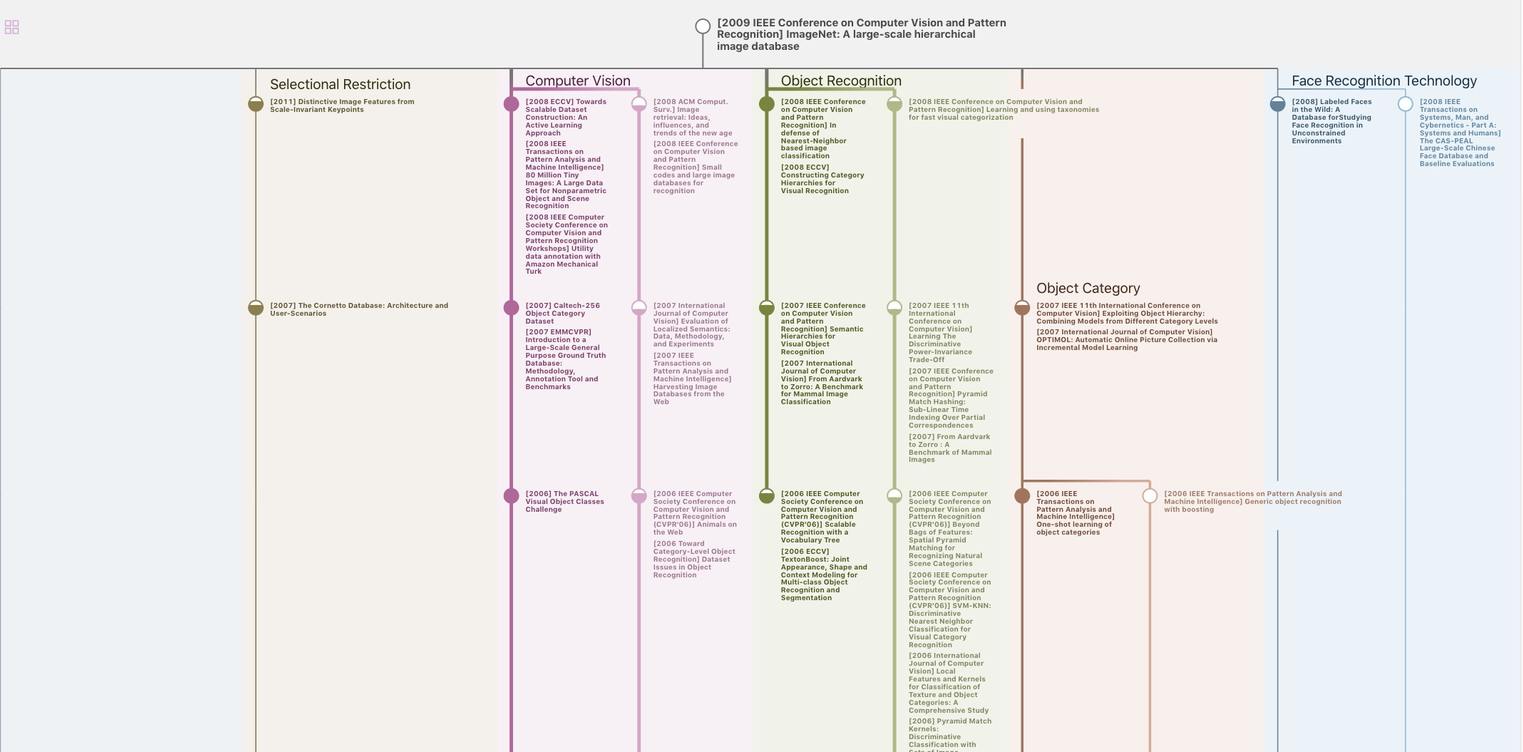
生成溯源树,研究论文发展脉络
Chat Paper
正在生成论文摘要