Genomic selection in sugar beet breeding populations
BMC genetics(2013)
摘要
Background Genomic selection exploits dense genome-wide marker data to predict breeding values. In this study we used a large sugar beet population of 924 lines representing different germplasm types present in breeding populations: unselected segregating families and diverse lines from more advanced stages of selection. All lines have been intensively phenotyped in multi-location field trials for six agronomically important traits and genotyped with 677 SNP markers. Results We used ridge regression best linear unbiased prediction in combination with fivefold cross-validation and obtained high prediction accuracies for all except one trait. In addition, we investigated whether a calibration developed based on a training population composed of diverse lines is suited to predict the phenotypic performance within families. Our results show that the prediction accuracy is lower than that obtained within the diverse set of lines, but comparable to that obtained by cross-validation within the respective families. Conclusions The results presented in this study suggest that a training population derived from intensively phenotyped and genotyped diverse lines from a breeding program does hold potential to build up robust calibration models for genomic selection. Taken together, our results indicate that genomic selection is a valuable tool and can thus complement the genomics toolbox in sugar beet breeding.
更多查看译文
关键词
Prediction Accuracy,Sugar Beet,Genomic Selection,Genomic Prediction,High Prediction Accuracy
AI 理解论文
溯源树
样例
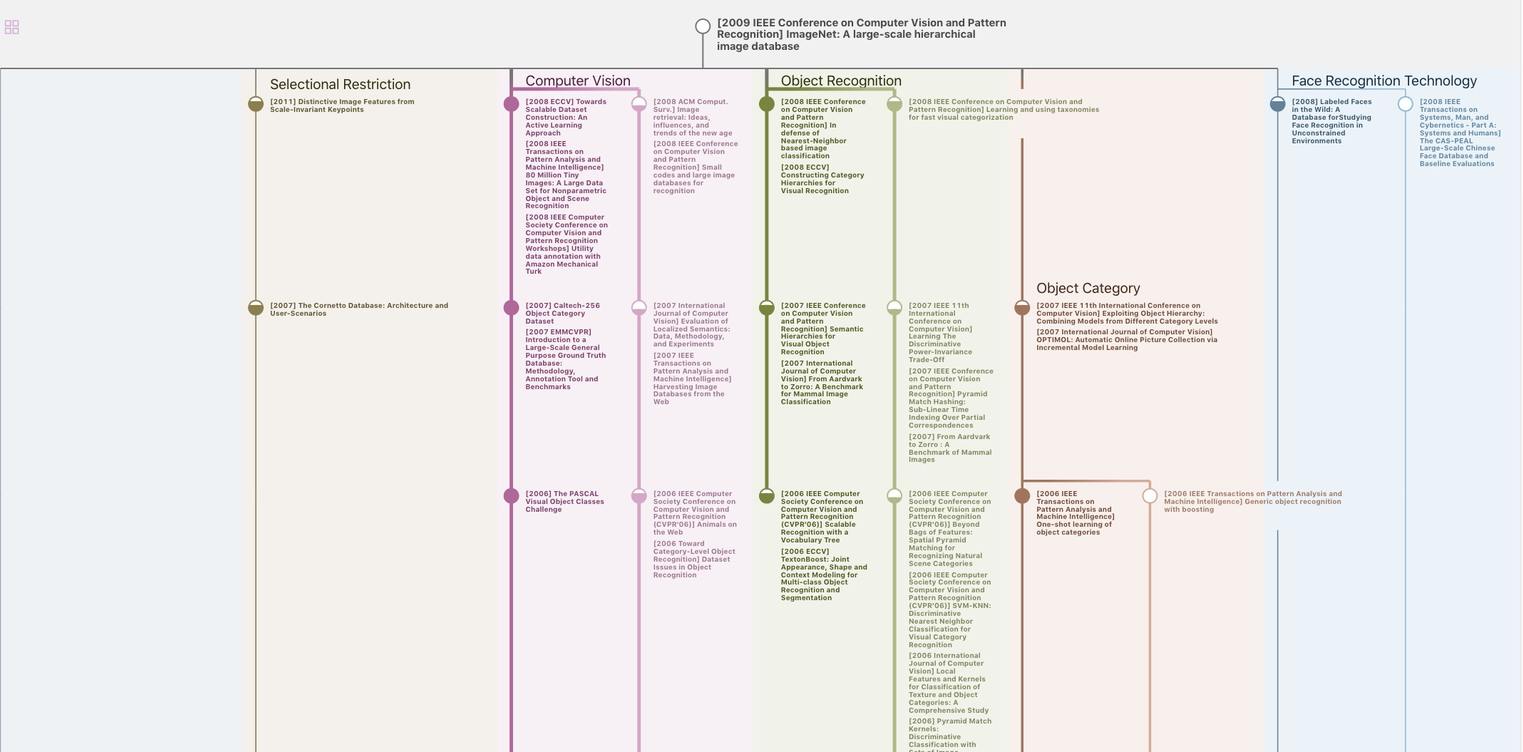
生成溯源树,研究论文发展脉络
Chat Paper
正在生成论文摘要