Classification of Small Lesions in Breast MRI: Evaluating The Role of Dynamically Extracted Texture Features Through Feature Selection.
JOURNAL OF MEDICAL AND BIOLOGICAL ENGINEERING(2013)
摘要
Dynamic texture quantification, i.e., extracting texture features from the lesion enhancement pattern in all available post-contrast images, has not been evaluated in terms of its ability to classify small lesions. This study investigates the classification performance achieved with texture features extracted from all five post-contrast images of lesions (mean lesion diameter of 1.1 cm) annotated in dynamic breast magnetic resonance imaging exams. Sixty lesions are characterized dynamically using Haralick texture features. The texture features are then used in a classification task with support vector regression and a fuzzy k-nearest neighbor classifier; free parameters of these classifiers are optimized using random sub-sampling cross-validation. Classifier performance is determined through receiver-operator characteristic (ROC) analysis, specifically through computation of the area under the ROC curve (AUC). Mutual information is used to evaluate the contribution of texture features extracted from different post-contrast stages to classifier performance. Significant improvements (p < 0.05) are observed for six of the thirteen texture features when the lesion enhancement pattern is quantified using the proposed approach of dynamic texture quantification. The highest AUC value observed (0.82) is achieved with texture features responsible for capturing aspects of lesion heterogeneity. Mutual information analysis reveals that texture features extracted from the third and fourth post-contrast images contributed most to the observed improvement in classifier performance. These results show that the performance of automated character classification with small lesions can be significantly improved through dynamic texture quantification of the lesion enhancement pattern.
更多查看译文
关键词
Dynamic breast magnetic resonance imaging (MRI),Texture analysis,Gray-level co-occurence matrices,Mutual information,Support vector regression
AI 理解论文
溯源树
样例
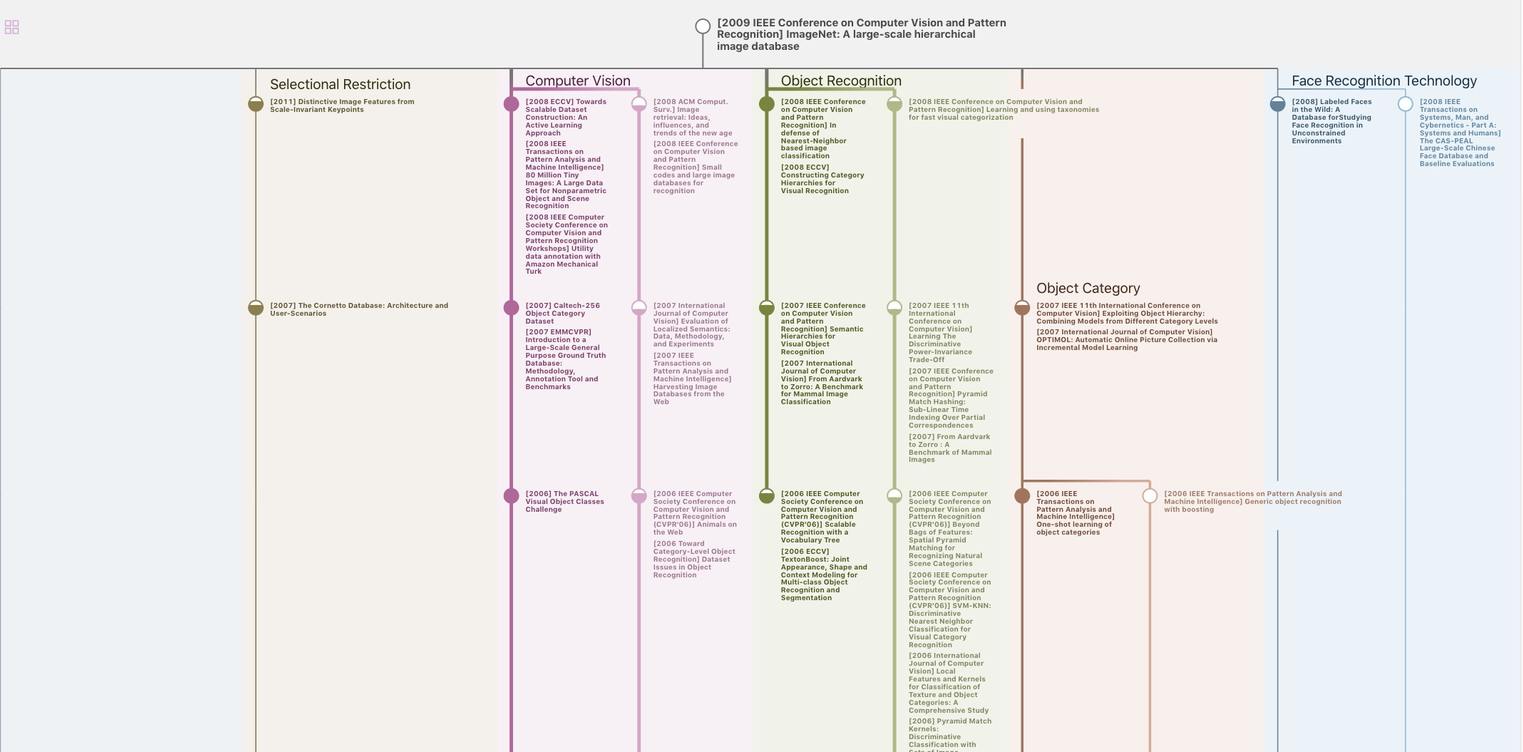
生成溯源树,研究论文发展脉络
Chat Paper
正在生成论文摘要