A geospatial model of ambient sound pressure levels in the contiguous United States.
JOURNAL OF THE ACOUSTICAL SOCIETY OF AMERICA(2014)
摘要
This paper presents a model that predicts measured sound pressure levels using geospatial features such as topography, climate, hydrology, and anthropogenic activity. The model utilizes RANDOM FOREST, a tree-based machine learning algorithm, which does not incorporate a priori knowledge of source characteristics or propagation mechanics. The response data encompasses 270 000 h of acoustical measurements from 190 sites located in National Parks across the contiguous United States. The explanatory variables were derived from national geospatial data layers and cross validation procedures were used to evaluate model performance and identify variables with predictive power. Using the model, the effects of individual explanatory variables on sound pressure level were isolated and quantified to reveal systematic trends across environmental gradients. Model performance varies by the acoustical metric of interest; the seasonal L-50 can be predicted with a median absolute deviation of approximately 3 dB. The primary application for this model is to generalize point measurements to maps expressing spatial variation in ambient sound levels. An example of this mapping capability is presented for Zion National Park and Cedar Breaks National Monument in southwestern Utah. (C) 2014 Acoustical Society of America.
更多查看译文
AI 理解论文
溯源树
样例
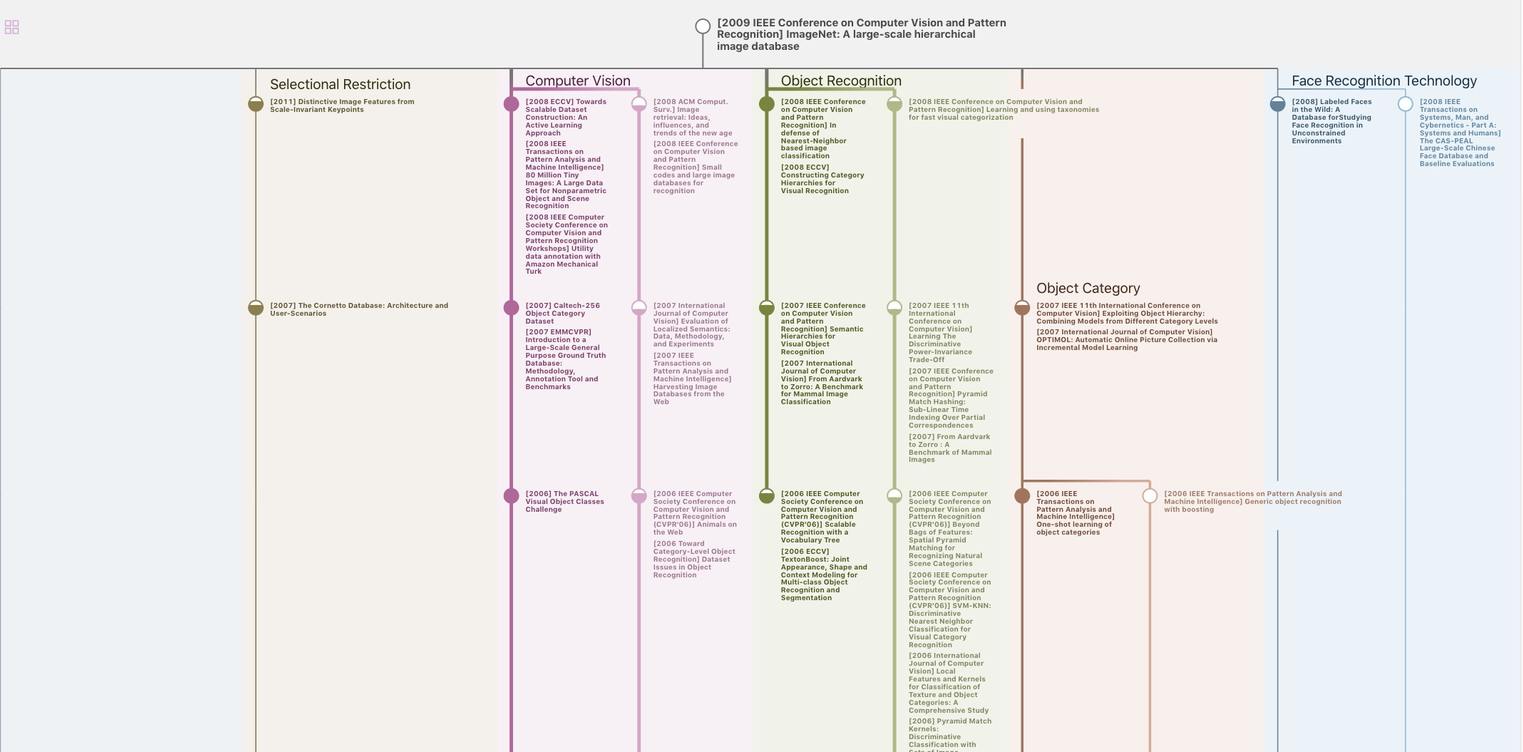
生成溯源树,研究论文发展脉络
Chat Paper
正在生成论文摘要