A Case Study of Early Performance Prediction and Intervention in a Computer Science Course
2020 ASEE Virtual Annual Conference Content Access Proceedings
摘要
This work presents the results of an intervention study performed in an upper-division undergraduate computer science (CS) course with 348 students, designed to offer additional resources to students that were identified as “at-risk” of low performance after completing graded assessments during the first two weeks of the semester. The course uses Python as the required programming language, however not every student that takes the class has prior experience with Python. Moreover, the overall programming experience is not uniform among the students, which can be partially explained due to the large diversity of majors in the class (44% CS, 19% CS + X, 19% Engineering and 18% other majors). This disparity in programming skills can greatly affect the overall student’s experience in the classroom and potentially their overall course performance. Other studies have shown how prerequisite grades are used to predict a student's performance in a course. For example, Liao et al [1] determined that prerequisite grades are the most predictive data for upper-division courses. However, for introductory programming courses, in-class short quiz questions are the most predictive data for student performance. In this study, we used data collected during the first two weeks of the semester to predict if a student was at risk of low performance. The available data consisted of two quizzes and two homework assignments covering linear algebra (course prerequisite) and basic Python programming. We used equivalent data from previous semesters to train a model using machine learning algorithms to predict students that were at risk of lower performance, here defined as a final grade less than 80% (roughly representing 30% of the class). Students that were identified as “at-risk” received an invitation to join a 6-week course, which was created to give students an additional opportunity to work on programming activities using Python. Out of the 60 students that received the invitation, 24 accepted and joined the short course that started on week 3 of the semester. The class met once a week for 80 minutes, and was held in an active learning classroom, where each group table had a large computer monitor and a white board. Students were split into groups of 5 and given a programming problem that required the entire group to collaborate. The tasks involved real world examples, designed in a structured way to allow students to complete the solution on their own, without a lot of guidance from the instructors. Focus groups were conducted along with a survey to capture student perceptions of the course. [1] Liao et al, “Exploring the Value of Different Data Sources for Predicting Student Performance in Multiple CS Courses”, SIGCSE '19.
更多查看译文
关键词
computer science course,early performance prediction,computer science,study
AI 理解论文
溯源树
样例
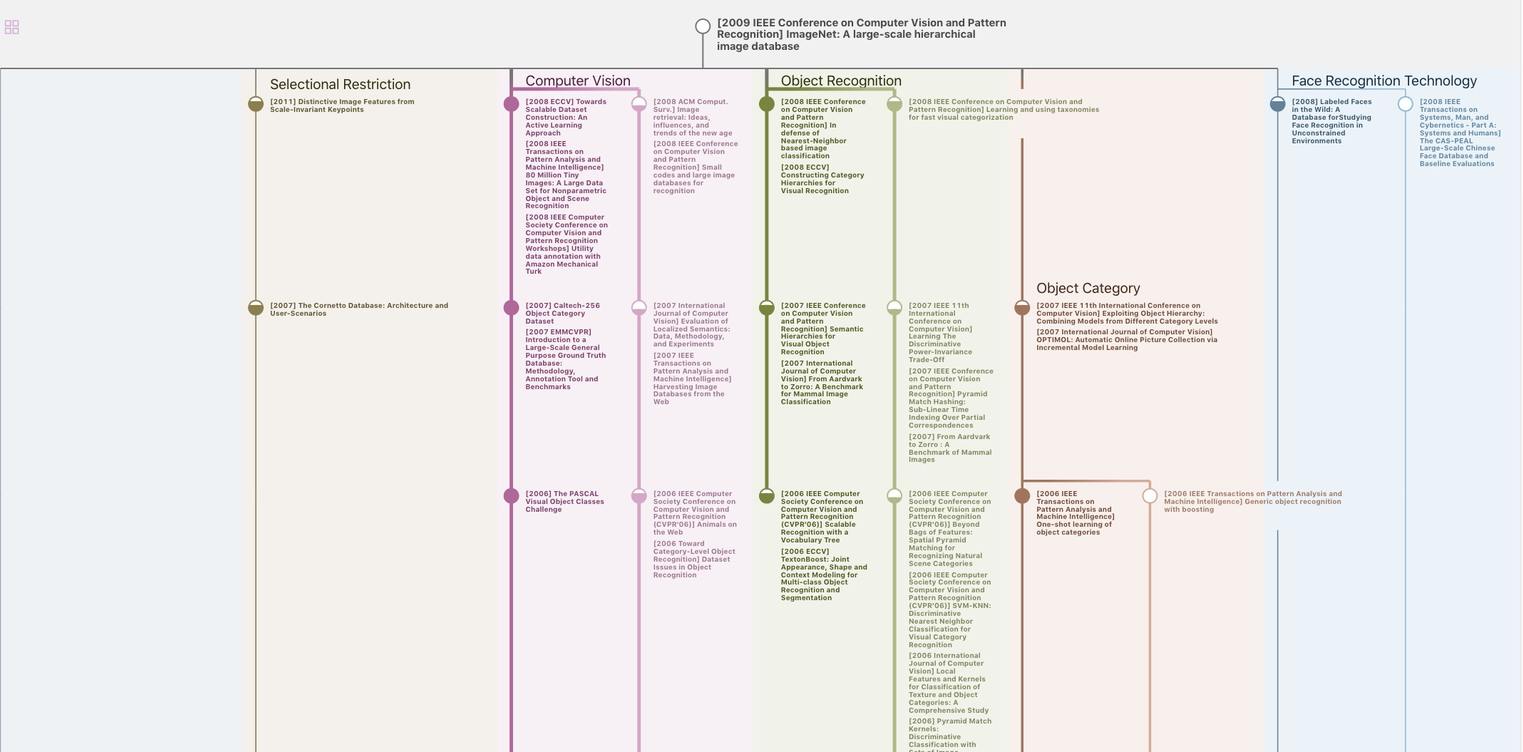
生成溯源树,研究论文发展脉络
Chat Paper
正在生成论文摘要