A Fully Traits-Based Approach To Modeling Global Vegetation Distribution
PROCEEDINGS OF THE NATIONAL ACADEMY OF SCIENCES OF THE UNITED STATES OF AMERICA(2014)
摘要
Dynamic Global Vegetation Models (DGVMs) are indispensable for our understanding of climate change impacts. The application of traits in DGVMs is increasingly refined. However, a comprehensive analysis of the direct impacts of trait variation on global vegetation distribution does not yet exist. Here, we present such analysis as proof of principle. We run regressions of trait observations for leaf mass per area, stem-specific density, and seed mass from a global database against multiple environmental drivers, making use of findings of global trait convergence. This analysis explained up to 52% of the global variation of traits. Global trait maps, generated by coupling the regression equations to gridded soil and climate maps, showed up to orders of magnitude variation in trait values. Subsequently, nine vegetation types were characterized by the trait combinations that they possess using Gaussian mixture density functions. The trait maps were input to these functions to determine global occurrence probabilities for each vegetation type. We prepared vegetation maps, assuming that the most probable (and thus, most suited) vegetation type at each location will be realized. This fully traits-based vegetation map predicted 42% of the observed vegetation distribution correctly. Our results indicate that a major proportion of the predictive ability of DGVMs with respect to vegetation distribution can be attained by three traits alone if traits like stem-specific density and seed mass are included. We envision that our traits-based approach, our observation-driven trait maps, and our vegetation maps may inspire a new generation of powerful traits-based DGVMs.
更多查看译文
关键词
functional variation, global vegetation map, probabilistic model, trait-environment relationships, vegetation attributes
AI 理解论文
溯源树
样例
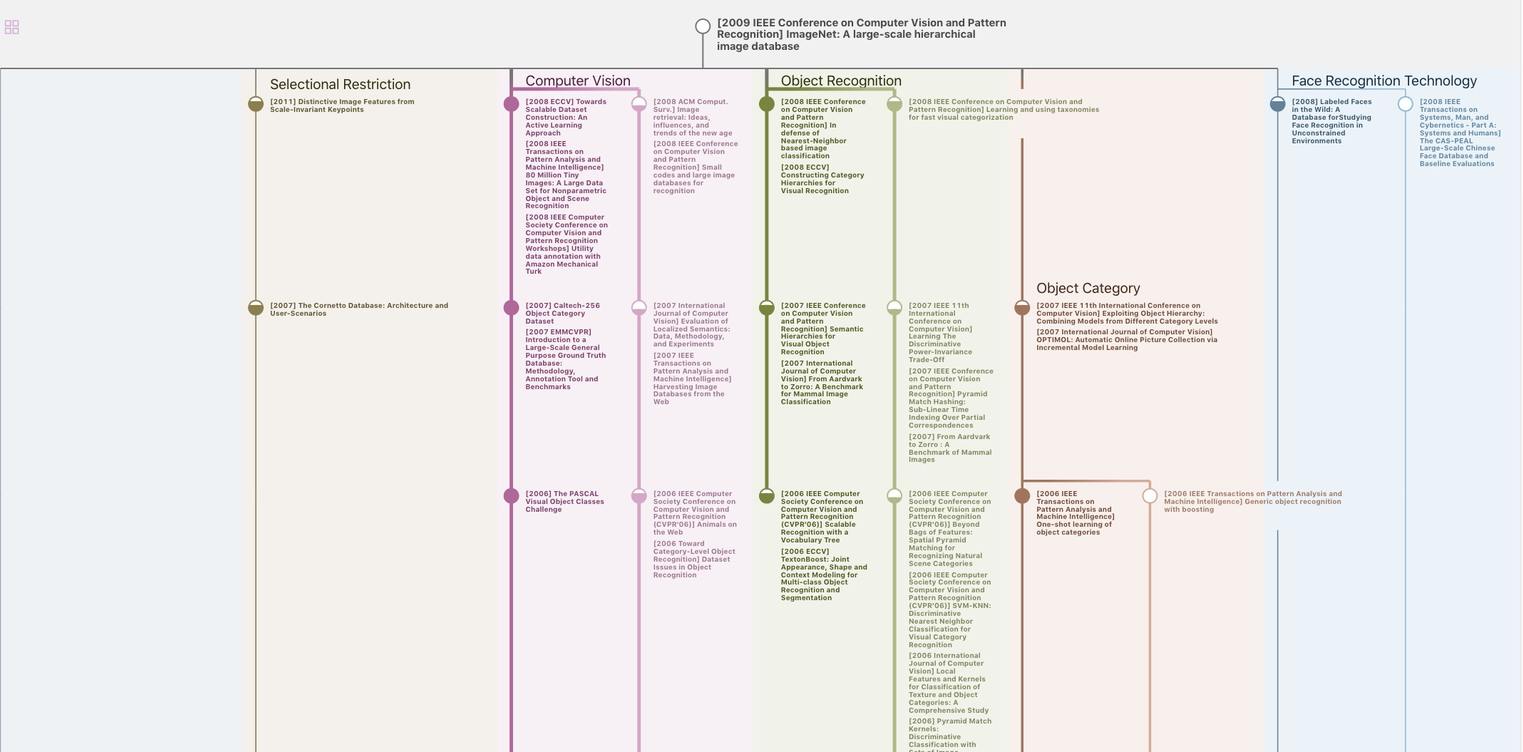
生成溯源树,研究论文发展脉络
Chat Paper
正在生成论文摘要