Automatic Classification of Laser-Induced Breakdown Spectroscopy (LIBS) Data of Protein Biomarker Solutions.
Applied spectroscopy(2014)
摘要
We perform multi-class classification of laser-induced breakdown spectroscopy data of four commercial samples of proteins diluted in phosphate-buffered saline solution at different concentrations: bovine serum albumin, osteopontin, leptin, and insulin-like growth factor II. We achieve this by using principal component analysis as a method for dimensionality reduction. In addition, we apply several different classification algorithms ( K-nearest neighbor, classification and regression trees, neural networks, support vector machines, adaptive local hyperplane, and linear discriminant classifiers) to perform multi-class classification. We achieve classification accuracies above 98% by using the linear classifier with 21–31 principal components. We obtain the best detection performance for neural networks, support vector machines, and adaptive local hyperplanes for a range of the number of principal components with no significant differences in performance except for that of the linear classifier. With the optimal number of principal components, a simplistic K-nearest classifier still provided acceptable results. Our proposed approach demonstrates that highly accurate automatic classification of complex protein samples from laser-induced breakdown spectroscopy data can be successfully achieved using principal component analysis with a sufficiently large number of extracted features, followed by a wrapper technique to determine the optimal number of principal components.
更多查看译文
关键词
Automatic identification of organic solutions,Laser-induced breakdown spectroscopy,Neural networks,Principal component analysis,Adaptive local hyperplane,K-nearest neighbors,Support vector machines
AI 理解论文
溯源树
样例
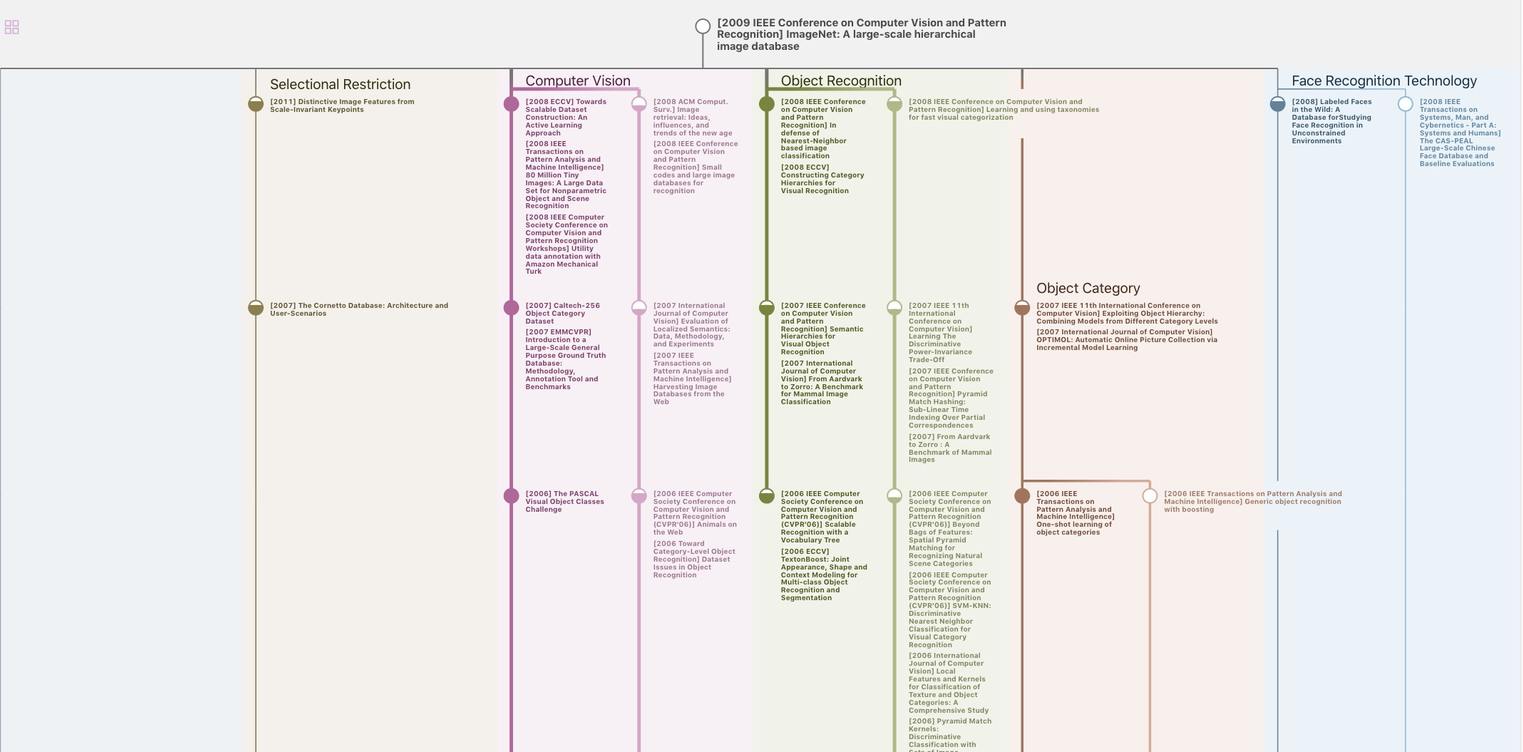
生成溯源树,研究论文发展脉络
Chat Paper
正在生成论文摘要