Bayesian model selection for pathological neuroimaging data applied to white matter lesion segmentation
IEEE Transactions on Medical Imaging(2015)
摘要
In neuroimaging studies, pathologies can present themselves as abnormal intensity patterns. Thus, solutions for detecting abnormal intensities are currently under investigation. As each patient is unique, an unbiased and biologically plausible model of pathological data would have to be able to adapt to the subject’s individual presentation. Such a model would provide the means for a better understanding of the underlying biological processes and improve one’s ability to define pathologically meaningful imaging biomarkers. With this aim in mind, this work proposes a hierarchical fully unsupervised model selection framework for neuroimaging data which enables the distinction between different types of abnormal image patterns without pathological a priori knowledge. Its application on simulated and clinical data demonstrated the ability to detect abnormal intensity clusters, resulting in a competitive to improved behavior in white matter lesion segmentation when compared to three other freelyavailable automated methods.
更多查看译文
关键词
Bayesian inference criterion (BIC), brain segmentation, Gaussian mixture model (GMM), magnetic resonance imaging (MRI), split-and-merge (SM) strategy, white matter lesion (WML)
AI 理解论文
溯源树
样例
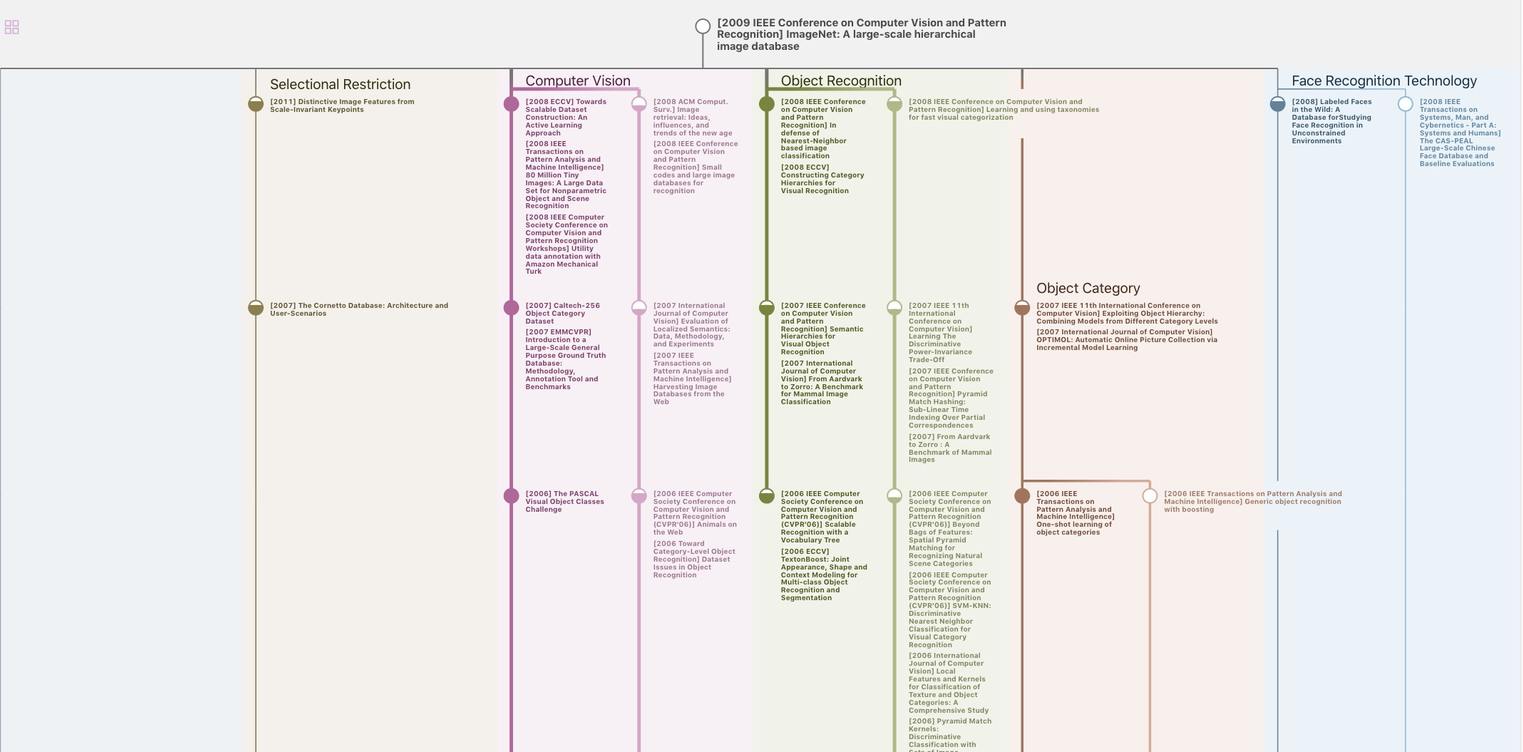
生成溯源树,研究论文发展脉络
Chat Paper
正在生成论文摘要