Advances in nowcasting influenza-like illness rates using search query logs
SCIENTIFIC REPORTS(2015)
摘要
User-generated content can assist epidemiological surveillance in the early detection and prevalence estimation of infectious diseases, such as influenza. Google Flu Trends embodies the first public platform for transforming search queries to indications about the current state of flu in various places all over the world. However, the original model significantly mispredicted influenza-like illness rates in the US during the 2012–13 flu season. In this work, we build on the previous modeling attempt, proposing substantial improvements. Firstly, we investigate the performance of a widely used linear regularized regression solver, known as the Elastic Net. Then, we expand on this model by incorporating the queries selected by the Elastic Net into a nonlinear regression framework, based on a composite Gaussian Process. Finally, we augment the query-only predictions with an autoregressive model, injecting prior knowledge about the disease. We assess predictive performance using five consecutive flu seasons spanning from 2008 to 2013 and qualitatively explain certain shortcomings of the previous approach. Our results indicate that a nonlinear query modeling approach delivers the lowest cumulative nowcasting error and also suggest that query information significantly improves autoregressive inferences, obtaining state-of-the-art performance.
更多查看译文
关键词
Applied mathematics, Computer science, Influenza virus, Epidemiology
AI 理解论文
溯源树
样例
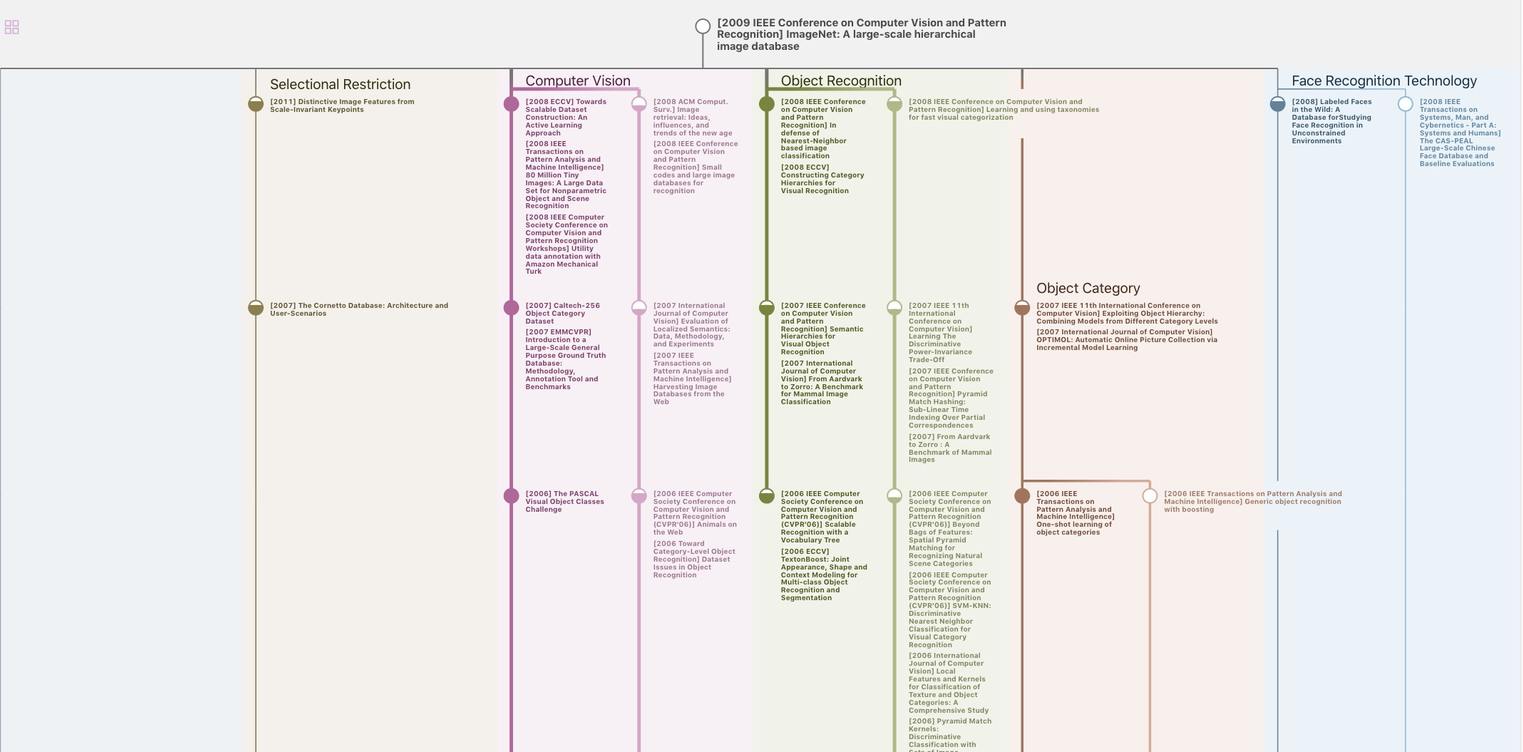
生成溯源树,研究论文发展脉络
Chat Paper
正在生成论文摘要