Noise peeling methods to improve boosting algorithms
Computational Statistics & Data Analysis(2016)
摘要
Boosting refers to a family of methods that combine sequences of individual classifiers into highly accurate ensemble models through weighted voting. AdaBoost, short for \"Adaptive Boosting\", is the most well-known boosting algorithm. AdaBoost has many strengths. Among them, there is sufficient empirical evidence pointing to its performance being generally superior to that of individual classifiers. In addition, even when combining a large number of weak learners, AdaBoost can be very robust to overfitting usually with lower generalization error than other competing ensemble methodologies, such as bagging and random forests. However, AdaBoost, as most hard margin classifiers, tends to be sensitive to outliers and noisy data, since it assigns observations that have been misclassified a higher weight in subsequent iterations. It has recently been proven that for any booster with a potential convex loss function, and any nonzero random classification noise rate, there is a data set, which can be efficiently learnable by the booster if there is no noise, but cannot be learned with accuracy better than 1/2 with random classification noise present. Several techniques to identify and potentially delete (peel) noisy samples in binary classification are proposed in order to improve the performance of AdaBoost. It is found that peeling methods generally perform better than AdaBoost and other noise resistant boosters, especially when high levels of noise are present in the data.
更多查看译文
关键词
AdaBoost,Ensembles,Generalization error,Noise detection,Noise filtering,Outliers
AI 理解论文
溯源树
样例
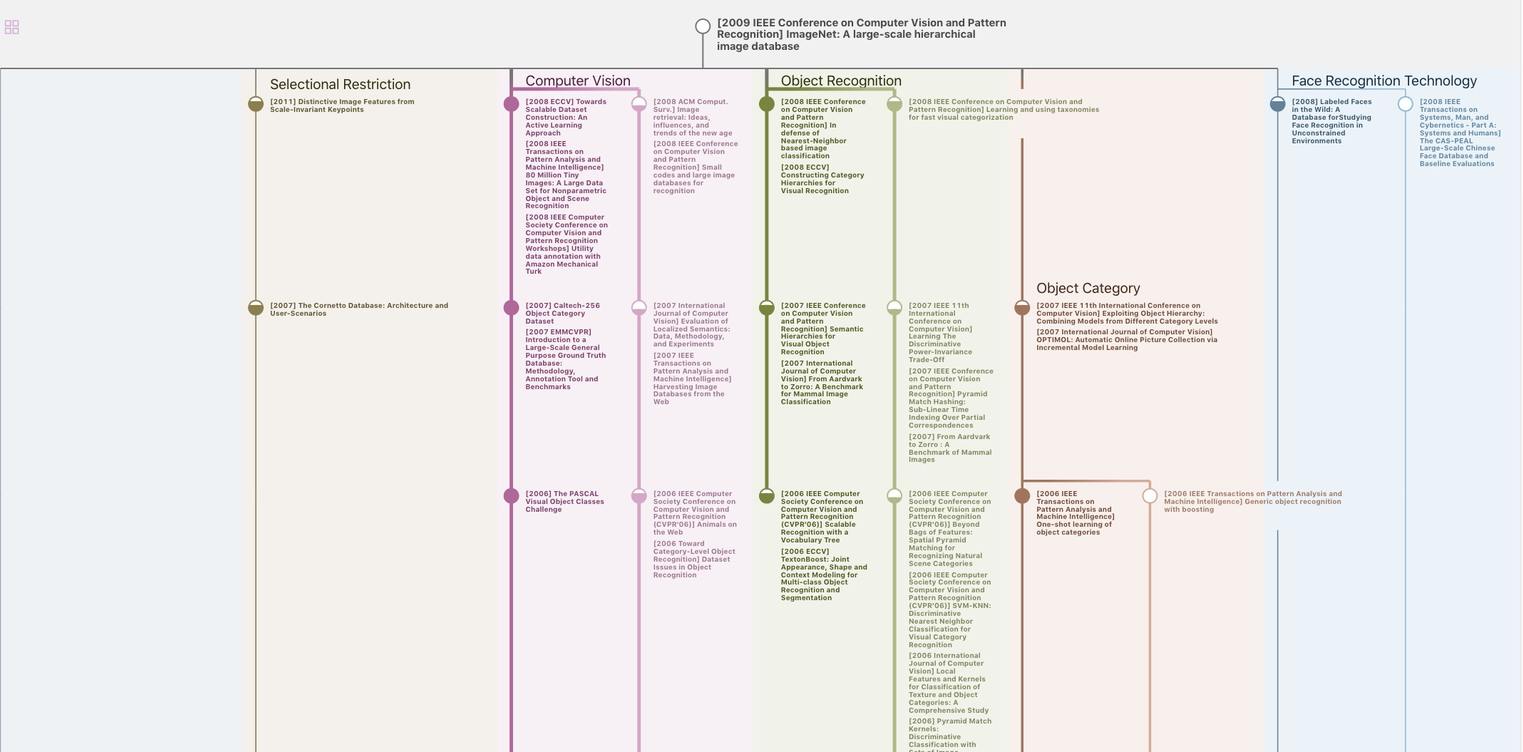
生成溯源树,研究论文发展脉络
Chat Paper
正在生成论文摘要