Hand movements classification for myoelectric control system using adaptive resonance theory
Australasian physical & engineering sciences in medicine / supported by the Australasian College of Physical Scientists in Medicine and the Australasian Association of Physical Sciences in Medicine(2015)
摘要
This research proposes an exploratory study of a simple, accurate, and computationally efficient movement classification technique for prosthetic hand application. Surface myoelectric signals were acquired from the four muscles, namely, flexor carpi ulnaris, extensor carpi radialis, biceps brachii, and triceps brachii, of four normal-limb subjects. The signals were segmented, and the features were extracted with a new combined time-domain feature extraction method. Fuzzy C-means clustering method and scatter plot were used to evaluate the performance of the proposed multi-feature versus Hudgins’ multi-feature. The movements were classified with a hybrid Adaptive Resonance Theory-based neural network. Comparative results indicate that the proposed hybrid classifier not only has good classification accuracy (89.09 %) but also a significantly improved computation time.
更多查看译文
关键词
Pattern recognition,EMG,Adaptive resonance theory,Neural network,Prosthetic hand
AI 理解论文
溯源树
样例
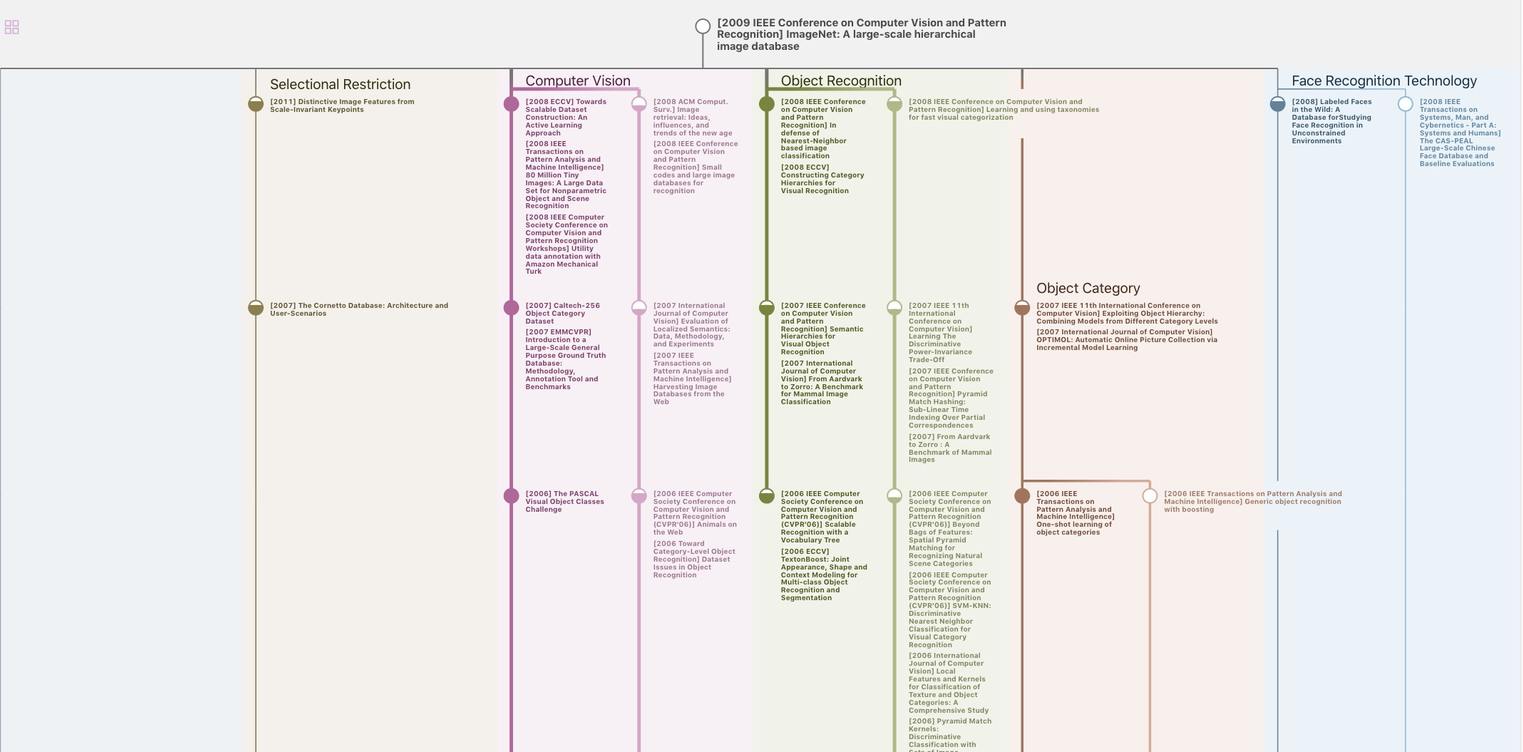
生成溯源树,研究论文发展脉络
Chat Paper
正在生成论文摘要