An Effective Cuda Parallelization Of Projection In Iterative Tomography Reconstruction
PLOS ONE(2015)
摘要
Projection and back-projection are the most computationally intensive parts in Computed Tomography (CT) reconstruction, and are essential to acceleration of CT reconstruction algorithms. Compared to back-projection, parallelization efficiency in projection is highly limited by racing condition and thread unsynchronization. In this paper, a strategy of Fixed Sampling Number Projection (FSNP) is proposed to ensure the operation synchronization in the ray-driven projection with Graphical Processing Unit (GPU). Texture fetching is also used utilized to further accelerate the interpolations in both projection and back-projection. We validate the performance of this FSNP approach using both simulated and real conebeam CT data. Experimental results show that compare to the conventional approach, the proposed FSNP method together with texture fetching is 10 similar to 16 times faster than the conventional approach based on global memory, and thus leads to more efficient iterative algorithm in CT reconstruction.
更多查看译文
关键词
texture,kernel functions,computed axial tomography,image processing,interpolation,convolution,algorithms
AI 理解论文
溯源树
样例
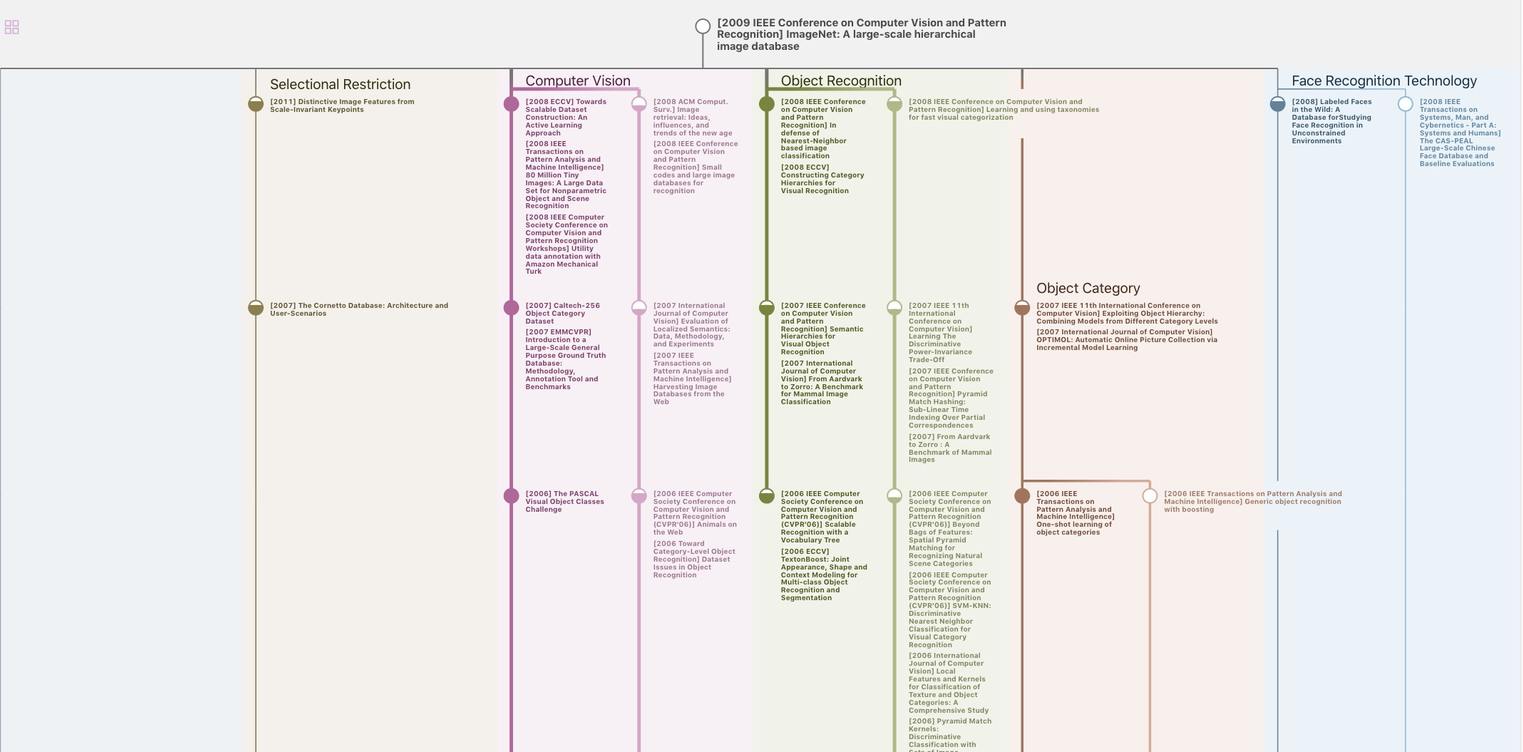
生成溯源树,研究论文发展脉络
Chat Paper
正在生成论文摘要