Integrating Model-Driven and Data-Driven Techniques for Analyzing Learning Behaviors in Open-Ended Learning Environments
IEEE transactions on learning technologies(2017)
摘要
Research in computer-based learning environments has long recognized the vital role of adaptivity in promoting effective, individualized learning among students. Adaptive scaffolding capabilities are particularly important in open-ended learning environments, which provide students with opportunities for solving authentic and complex problems, and the choice to adopt a variety of strategies and approaches to solving these problems. To help students overcome their difficulties and become effective learners and problem solvers, we have to develop methods that can track and interpret students' open-ended learning and problem-solving behaviors. The complexity of the problems and the open-ended nature of the solution processes pose considerable challenges to accurately interpret and evaluate student behaviors and performance as they work on the system. In this paper, we develop a framework that combines model-driven strategy detection with data-driven pattern discovery for analyzing students' learning activity data in open-ended environments. We present results from an in-depth case study of multiple activity patterns identified in data from the Betty's Brain learning environment. The results illustrate the benefits of combining model-and data-driven techniques to precisely characterize the learning behavior of students in an open-ended environment.
更多查看译文
关键词
Open-ended learning,environments,modeling learner,behaviors,hierarchical task modeling,activity sequence mining,coherence analysis,strategy modeling
AI 理解论文
溯源树
样例
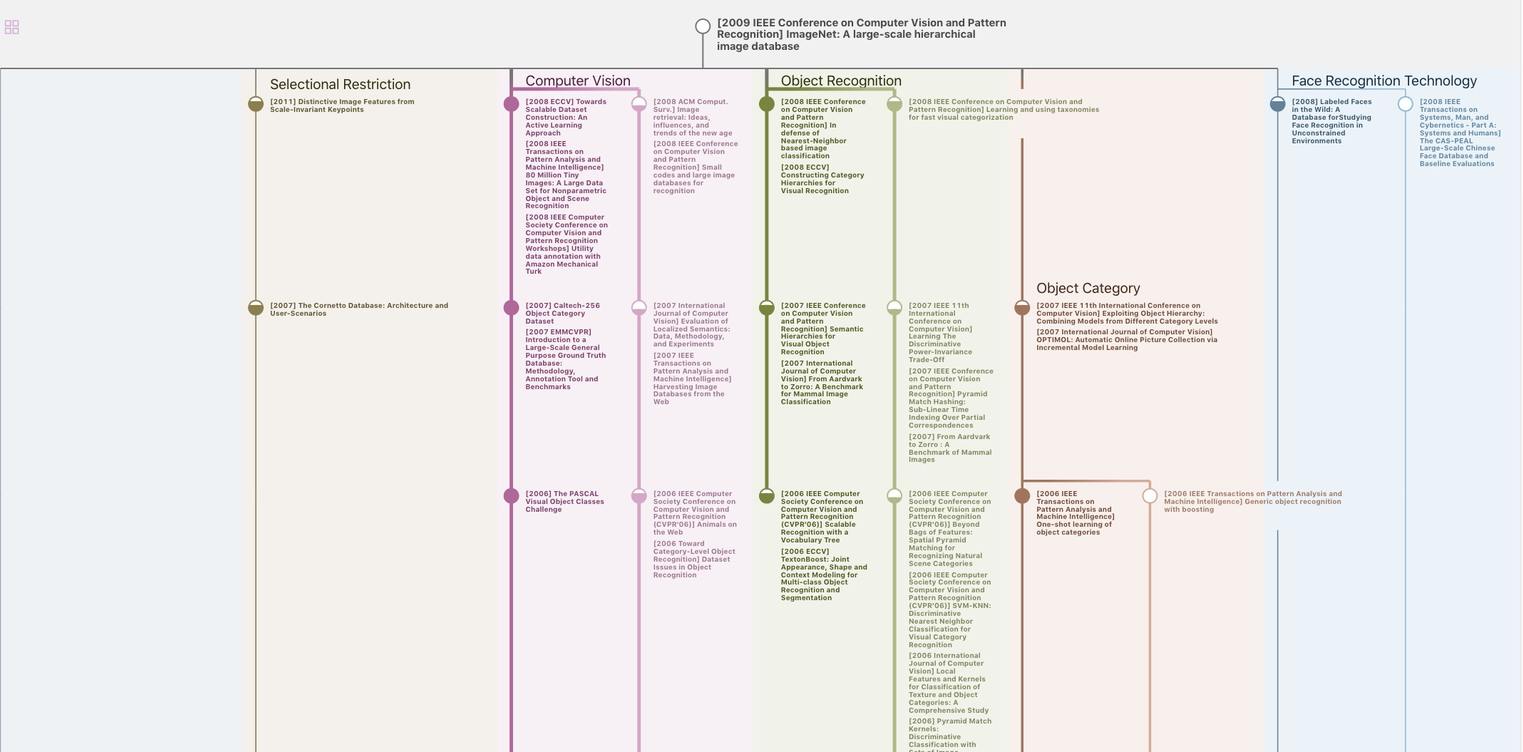
生成溯源树,研究论文发展脉络
Chat Paper
正在生成论文摘要