Enhanced single seed trait predictions in soybean (Glycine max) and robust calibration model transfer with near infrared reflectance spectroscopy.
JOURNAL OF AGRICULTURAL AND FOOD CHEMISTRY(2016)
摘要
Single seed near-infrared reflectance (NIR) spectroscopy predicts soybean (Glycine max) seed quality traits of moisture, oil, and protein. We tested the accuracy of transferring calibrations between different single seed NIR analyzers of the same design by collecting NIR spectra and analytical trait data for globally diverse soybean germplasm. X-ray microcomputed tomography (mu CT) was used to collect seed density and shape traits to enhance the number of soybean traits that can be predicted from single seed NIR Partial least-squares (PLS) regression gave accurate predictive models for oil, weight, volume, protein, and maximal cross-sectional area of the seed. PLS models for width, length, and density were not predictive. Although principal-component analysis (PCA) of the NIR spectra showed that black seed coat color had significant signal, excluding black seeds from the calibrations did not impact model accuracies. Calibrations for oil and protein developed in this study as well as earlier calibrations for a separate NIR analyzer of the same design were used to test the ability to transfer PLS regressions between platforms. PLS models built from data collected on one NIR analyzer had minimal differences in accuracy when applied to spectra collected from a sister device. Model transfer was more robust when spectra were trimmed from 910 to 1679 nm to 955-1635 run due to divergence of edge wavelengths between the two devices. The ability to transfer calibrations between similar single seed NIR spectrometers facilitates broader adoption of this high-throughput, nondestructive, seed phenotyping technology.:
更多查看译文
关键词
seed phenotyping,near-infrared spectroscopy,chemometrics,microcomputed tomography,density,oil,protein
AI 理解论文
溯源树
样例
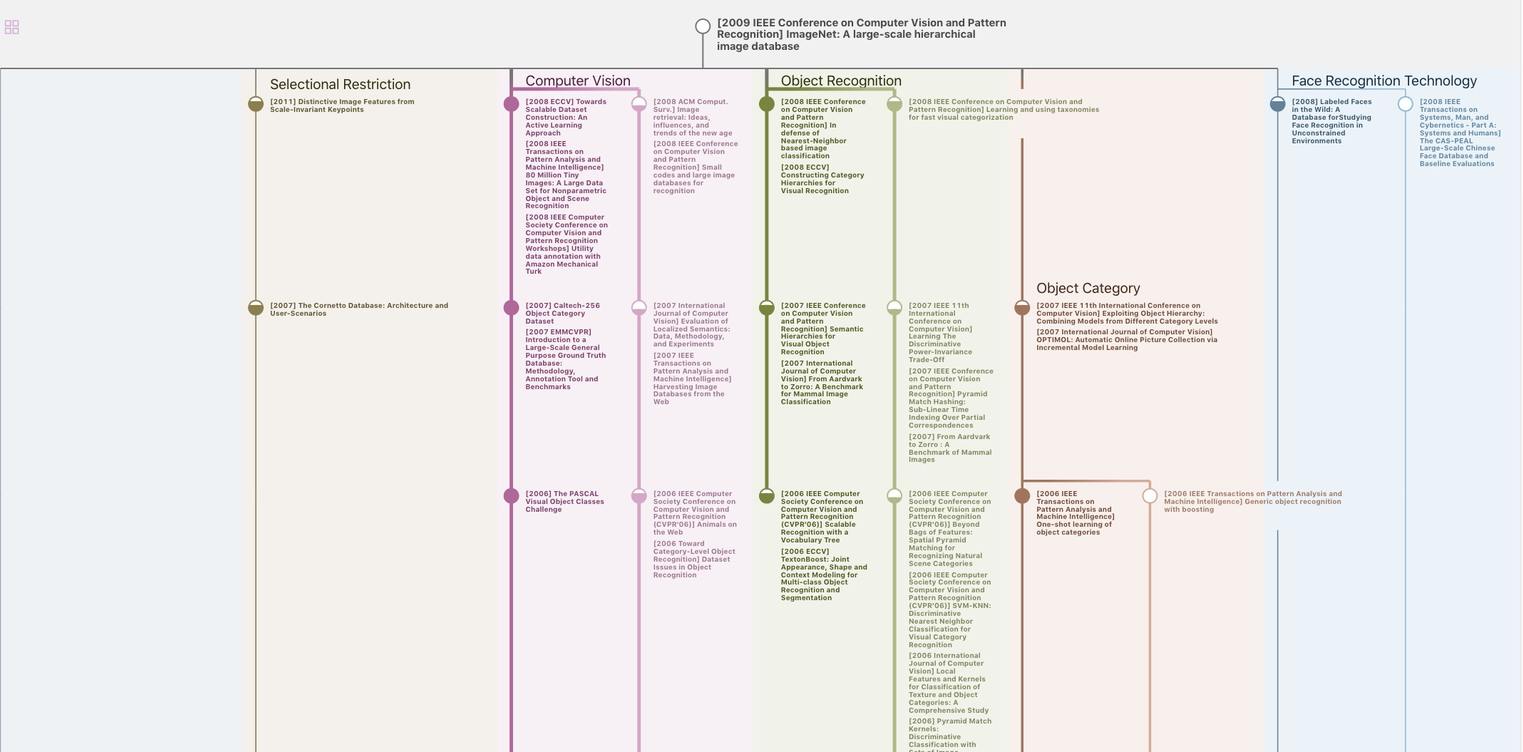
生成溯源树,研究论文发展脉络
Chat Paper
正在生成论文摘要