Kinetic Characterization Of 100 Glycoside Hydrolase Mutants Enables The Discovery Of Structural Features Correlated With Kinetic Constants
PLOS ONE(2016)
摘要
The use of computational modeling algorithms to guide the design of novel enzyme catalysts is a rapidly growing field. Force-field based methods have now been used to engineer both enzyme specificity and activity. However, the proportion of designed mutants with the intended function is often less than ten percent. One potential reason for this is that current force-field based approaches are trained on indirect measures of function rather than direct correlation to experimentally-determined functional effects of mutations. We hypothesize that this is partially due to the lack of data sets for which a large panel of enzyme variants has been produced, purified, and kinetically characterized. Here we report the k(cat) and K-M values of 100 purified mutants of a glycoside hydrolase enzyme. We demonstrate the utility of this data set by using machine learning to train a new algorithm that enables prediction of each kinetic parameter based on readily-modeled structural features. The generated dataset and analyses carried out in this study not only provide insight into how this enzyme functions, they also provide a clear path forward for the improvement of computational enzyme redesign algorithms.
更多查看译文
AI 理解论文
溯源树
样例
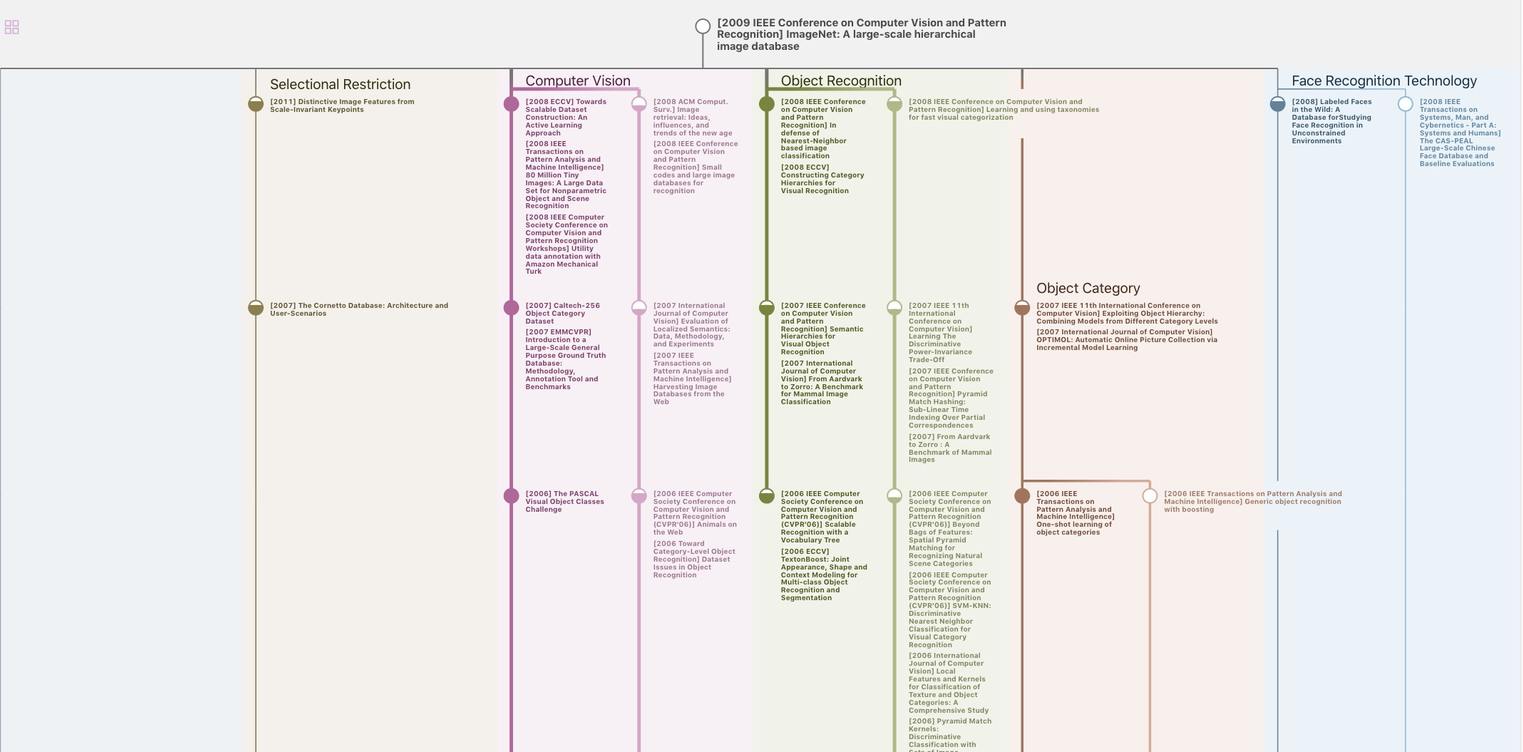
生成溯源树,研究论文发展脉络
Chat Paper
正在生成论文摘要