Permanents, $$\alpha $$-permanents and Sinkhorn balancing
Computational Statistics(2014)
摘要
The method of Sinkhorn balancing that starts with a non-negative square matrix and iterates to produce a related doubly stochastic matrix has been used with some success to estimate the values of the permanent in some cases of physical interest. However, it is often claimed that Sinkhorn balancing is slow to converge and hence not useful for efficient computation. In this paper, we explain how some simple, low cost pre-processing allows one to guarantee that Sinkhorn balancing always converges linearly. We illustrate this approach by efficiently and accurately computing permanents and \(\alpha \)-permanents of some previously studied matrices.
更多查看译文
关键词
Matrix scaling, Doubly stochastic matrix, Sequential importance sampling
AI 理解论文
溯源树
样例
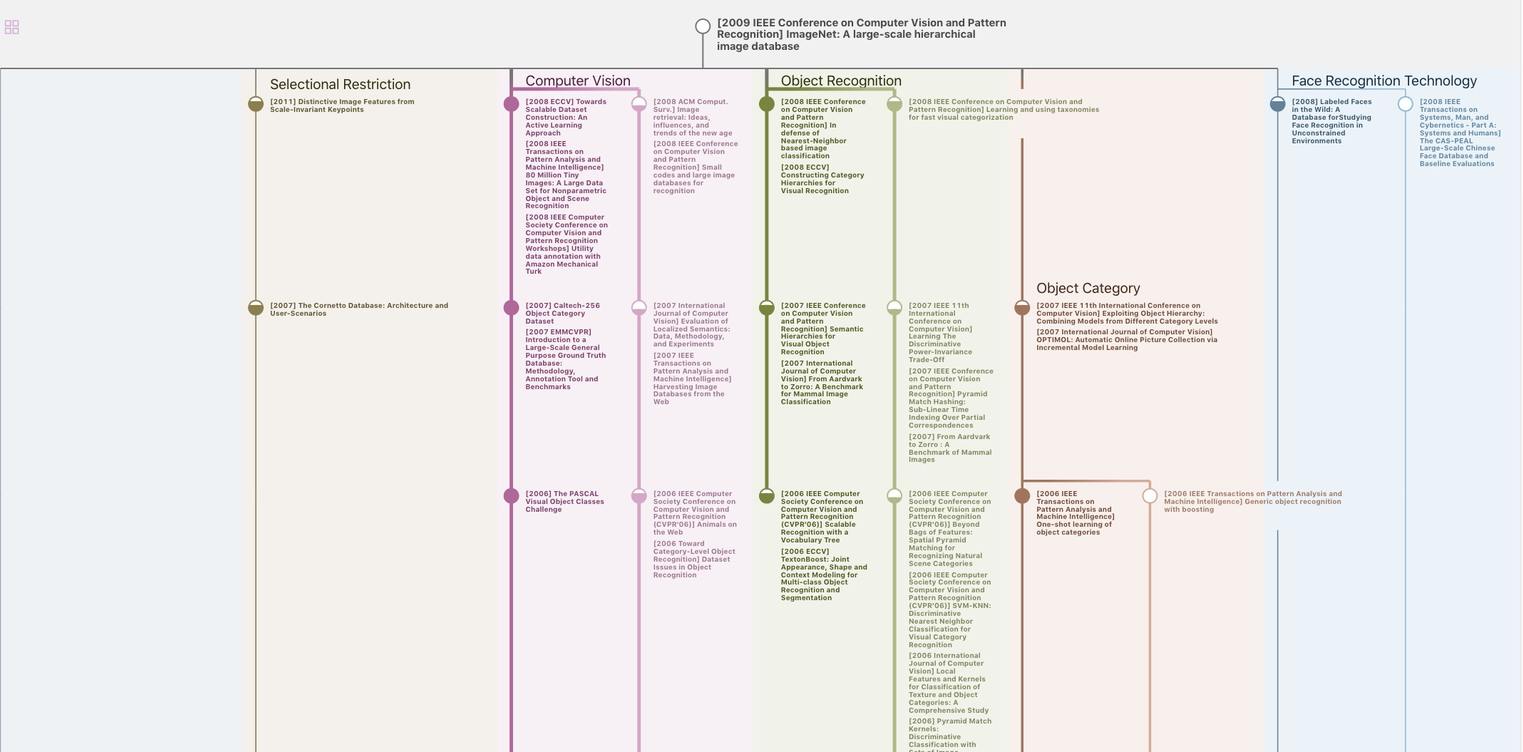
生成溯源树,研究论文发展脉络
Chat Paper
正在生成论文摘要