Control of Group Velocity Based on Nonlinear Kerr Effect in a Plasmonic Superlattice
Plasmonics(2015)SCI 4区
Department of Physics
Abstract
We propose a plasmonic superlattice with nonlinear Kerr medium in defect layer to realize slow light effect and demonstrate the group velocity control at telecom waveband (1550 nm) by peak intensity of input pulse. The tunable group velocity of surface plasmon polaritons is attributed to the change of dispersion of the superlattice caused by nonlinear Kerr effect. The method of controlling group velocity is analyzed by transfer matrix method based on characteristic impedance and confirmed by the finite-difference time-domain numerical simulation. Our method of control group velocity potentially applies in the tunable optics delay line.
MoreTranslated text
Key words
Slow surface plasmon polaritons (SPPs),Group velocity control,Nonlinear Kerr effect,Superlattice
PDF
View via Publisher
AI Read Science
AI Summary
AI Summary is the key point extracted automatically understanding the full text of the paper, including the background, methods, results, conclusions, icons and other key content, so that you can get the outline of the paper at a glance.
Example
Background
Key content
Introduction
Methods
Results
Related work
Fund
Key content
- Pretraining has recently greatly promoted the development of natural language processing (NLP)
- We show that M6 outperforms the baselines in multimodal downstream tasks, and the large M6 with 10 parameters can reach a better performance
- We propose a method called M6 that is able to process information of multiple modalities and perform both single-modal and cross-modal understanding and generation
- The model is scaled to large model with 10 billion parameters with sophisticated deployment, and the 10 -parameter M6-large is the largest pretrained model in Chinese
- Experimental results show that our proposed M6 outperforms the baseline in a number of downstream tasks concerning both single modality and multiple modalities We will continue the pretraining of extremely large models by increasing data to explore the limit of its performance
Try using models to generate summary,it takes about 60s
Must-Reading Tree
Example
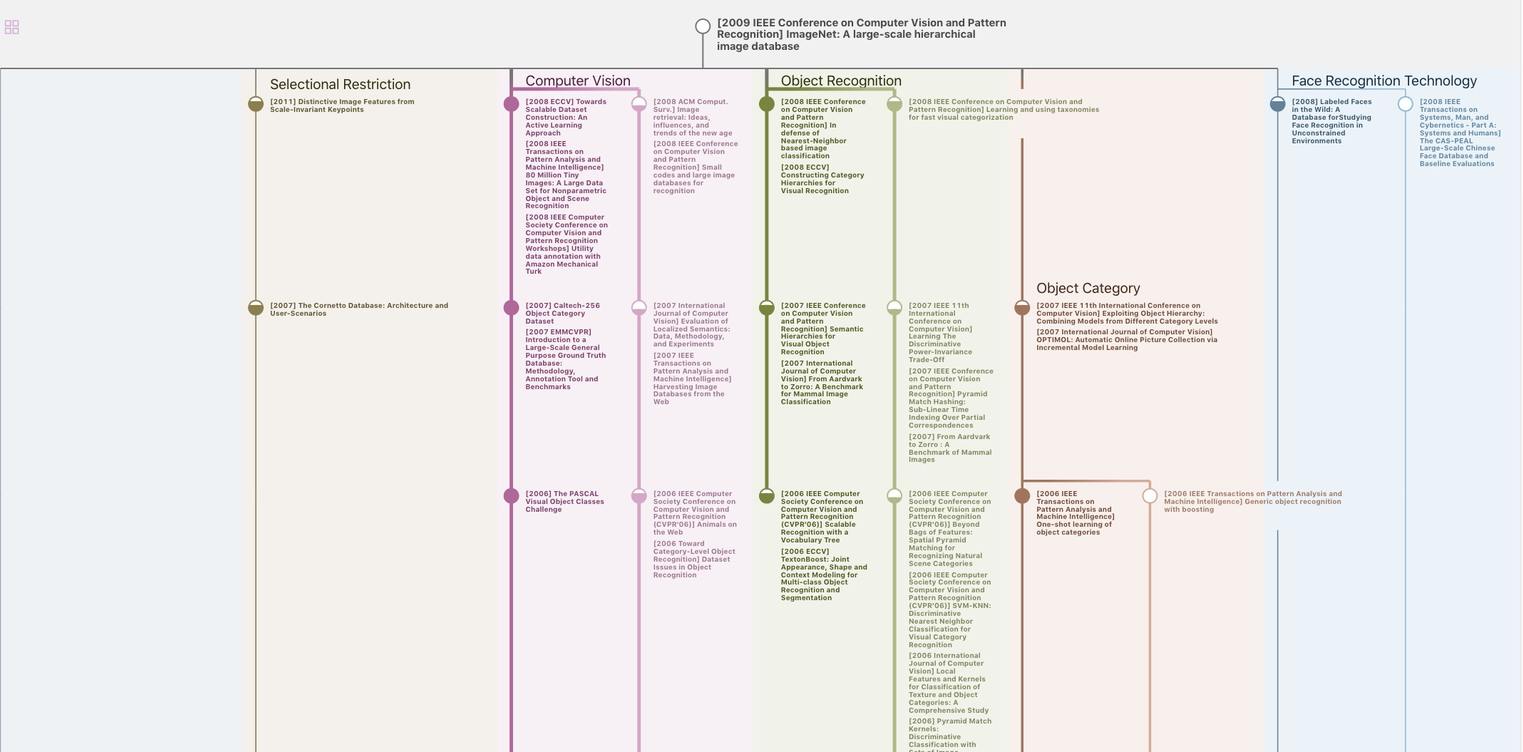
Generate MRT to find the research sequence of this paper
Related Papers
A Facile Strategy for All-Optical Controlling Platform by Using Plasmonic Perfect Absorbers
Plasmonics 2018
被引用4
Theoretical Study of Ultra-Wideband Slow Light in Dual-Stub-coupled Plasmonic Waveguide
Optics Communications 2016
被引用7
Ultra-broadband and Tunable Slow Surface Plasmon Polaritions in a Simple Graphene-Based Waveguide
Journal of Optics 2018
被引用1
OPTICS COMMUNICATIONS 2024
被引用1
Data Disclaimer
The page data are from open Internet sources, cooperative publishers and automatic analysis results through AI technology. We do not make any commitments and guarantees for the validity, accuracy, correctness, reliability, completeness and timeliness of the page data. If you have any questions, please contact us by email: report@aminer.cn
Chat Paper
GPU is busy, summary generation fails
Rerequest