Decoding Motor Cortical Spike Trains For Brain Machine Interface Applications
PROCEEDINGS OF THE 25TH ANNUAL INTERNATIONAL CONFERENCE OF THE IEEE ENGINEERING IN MEDICINE AND BIOLOGY SOCIETY, VOLS 1-4: A NEW BEGINNING FOR HUMAN HEALTH(2003)
摘要
This paper addresses the issue of cortical control using motor neurons. We examined data from both open-loop off-line and close-loop online motor control tasks: Given multineuron recordings of action potentials, determine rats' paddle press intentions as they respond to the onset of light cues. We systematically evaluated two classes of machine learning classification techniques, the self-organizing maps (SOM), and the support vector machines (SVM). The SOM based algorithm was augmented by a Bayesian decision model for classification of control commands. To circumvent the difficulty of large number of data variables and relatively low number of data samples, principle component analysis (PCA) and partial least squares (PLS) were used as dimension reduction techniques associated with the classifiers. We demonstrated using recordings from 5 rats that the proposed algorithms provide much more accurate control decisions than those by chance. Our results provided validation of the feasibility of real-time brain control with feedback using predictive models between cortical firing patterns and control commands. Our real-time brain control does not require desired target information in decoding as some other algorithms did. In addition, careful comparisons and evaluations are provided for several different implementations of the decoding techniques.
更多查看译文
关键词
motor cortical control, brain-machine interface, spike train decoding, SOM, SVM
AI 理解论文
溯源树
样例
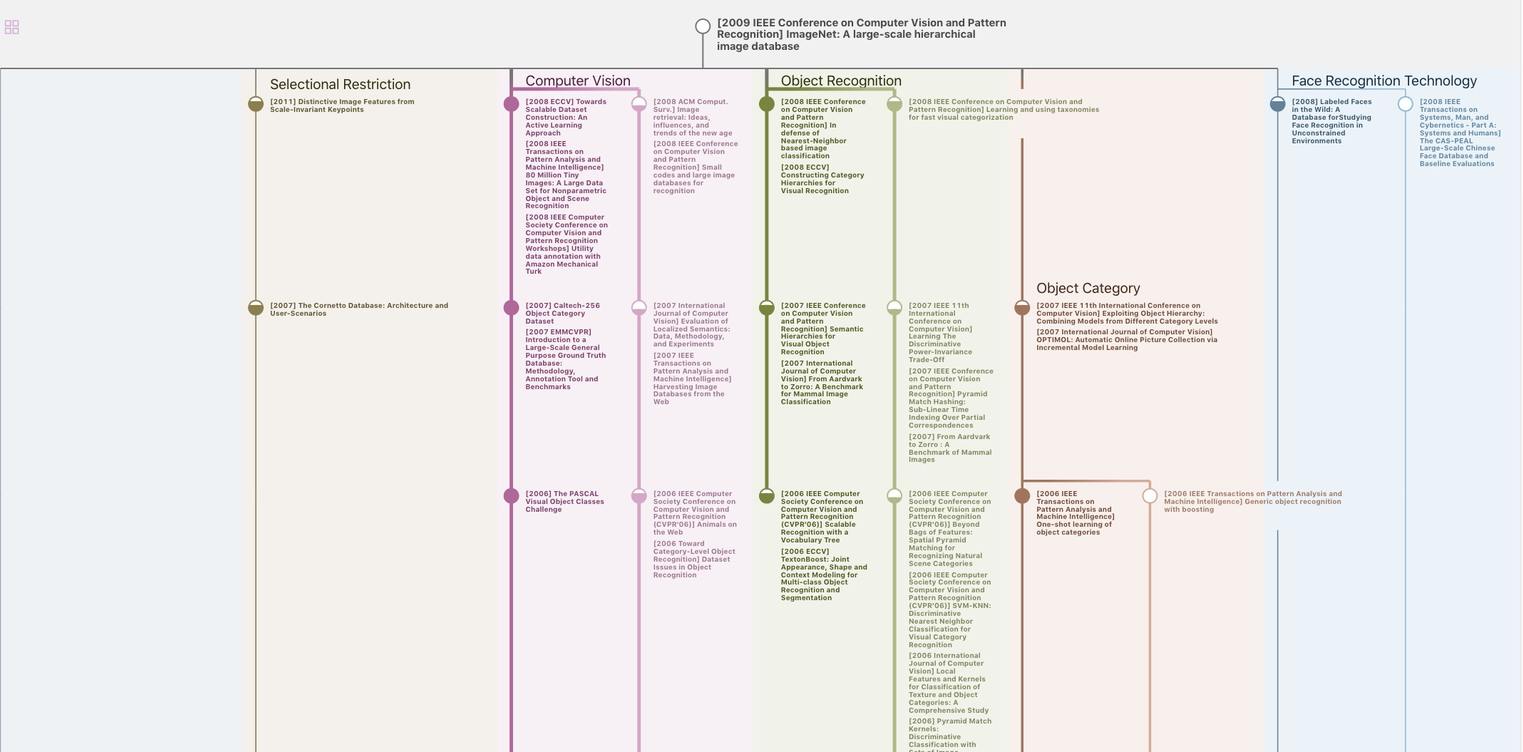
生成溯源树,研究论文发展脉络
Chat Paper
正在生成论文摘要