Difference representation learning using stacked restricted Boltzmann machines for change detection in SAR images
Soft Comput.(2014)
摘要
In this paper, we establish a deep neural network using stacked Restricted Boltzmann Machines (RBMs) to analyze the difference images and detect changes between multitemporal synthetic aperture radar (SAR) images. Given the two multitemporal images, a difference image which shows difference degrees between corresponding pixels is generated. Then, RBMs are stacked to form a deep hierarchical neural network to learn to analyze the difference image and recognize the changed pixels and unchanged pixels. The learning process includes unsupervised layer-wise feature learning and supervised fine-tuning of network parameters. Unsupervised learning aims to learn the representation of the difference image. Supervised fine-tuning aims to learn to classify the changed and unchanged pixels. The network can learn from datasets that have few labeled data. The labeled data can be selected from the results obtained by other methods because there is no prior information in image change detection. The system learns to detect the changes instead of recognizing the changes by fixed equations as in traditional change detection algorithms. We test the network with real synthetic aperture radar datasets and the labeled samples are extracted from the results obtained, respectively, by several methods, including a thresholding method, a level set method and two clustering methods. The results achieved by the trained network outperform that of other methods.
更多查看译文
关键词
Image change detection,Deep neural network,Representation learning,Synthetic aperture radar
AI 理解论文
溯源树
样例
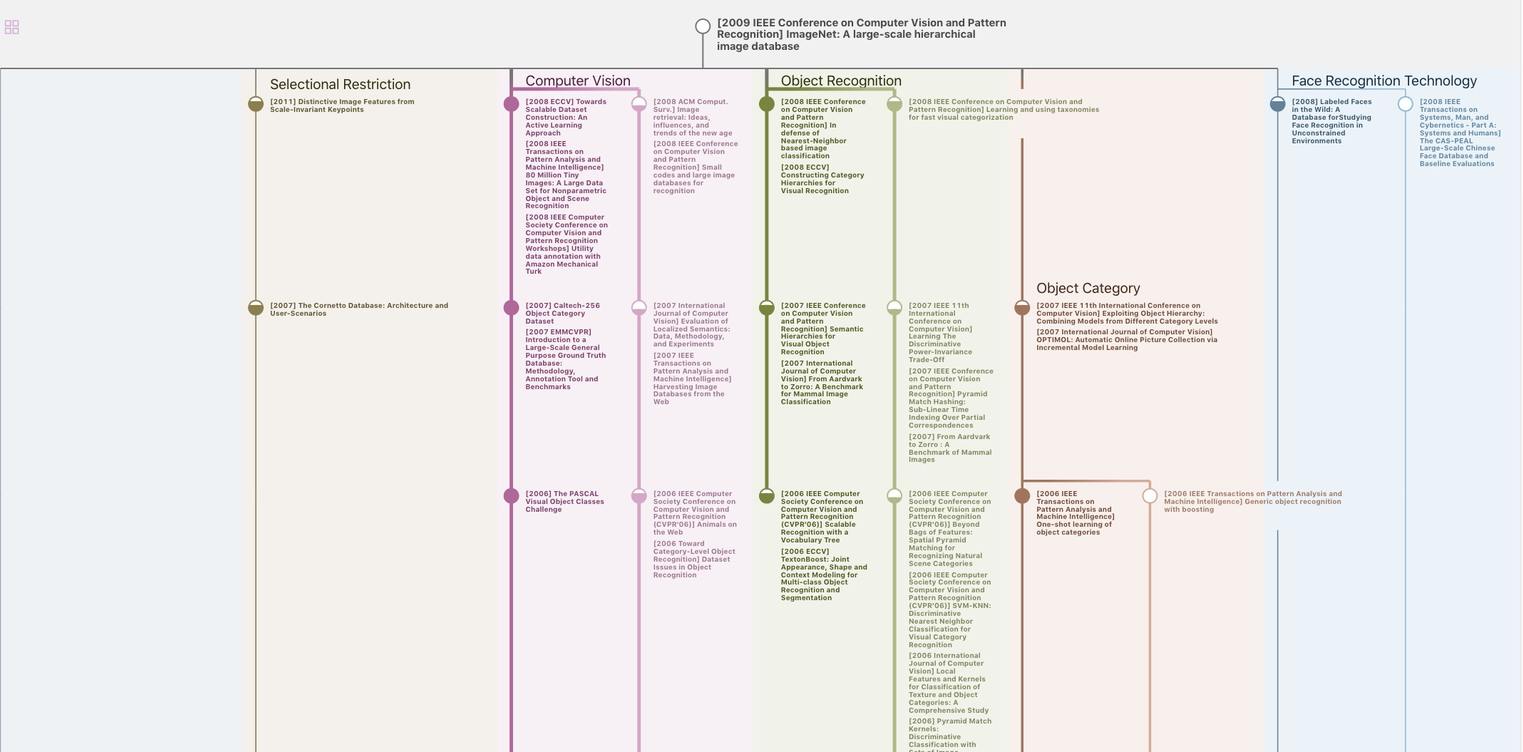
生成溯源树,研究论文发展脉络
Chat Paper
正在生成论文摘要