A unified probabilistic PLSR model for quantitative analysis of Surface-Enhanced Raman Spectrum (SERS)
international conference on communications(2014)
摘要
Gold Surface-enhanced Raman Scattering (Au SERS) nano-particles in combination with Raman spectroscopy have occurred as a newly sensitive, non-invasive molecular imaging technology. The multiplexing capability enables the technology to detect and separate multiple biomarkers with picomolar sensitivity. In this study, we demonstrate the ability of Raman spectroscopy to separate different fingerprints of Au SERS nanotags. Quantitative analysis of Raman spectrum data usually faces the challenge as high dimensional variables with a low sample number. The commonly applied partial least squares (PLS) regression algorithms, including PLS2 and SIMPLS, can not avoid overfitting to small data sets. In this paper, we present a unified probabilistic PLSR model, called PPLSR, stemmed from the concepts of probabilistic principal component analysis (PPCA) and probabilistic canonical correlation analysis (PCCA) to identify the spectral fingerprints from the measured mixing Raman signals. This model partitions the observed variables into the systematic part governed by a few latent variables and the unrelated noise part controlling the uncertainty of data sets. As a general methodology, this provides a solid foundation to develop Bayesian nonparametrics models and helps to build more robust models. Experimental results of Raman spectrum data using up to five different types of Au SERS nanotags with different combinations and mixing ratios are shown. Quantitative analysis using the proposed model and comparison methods are given with two cross-validation methods.
更多查看译文
关键词
Partial Little Square, Partial Little Square Regression, Raman Signal, Principal Component Regression, Residual Matrix
AI 理解论文
溯源树
样例
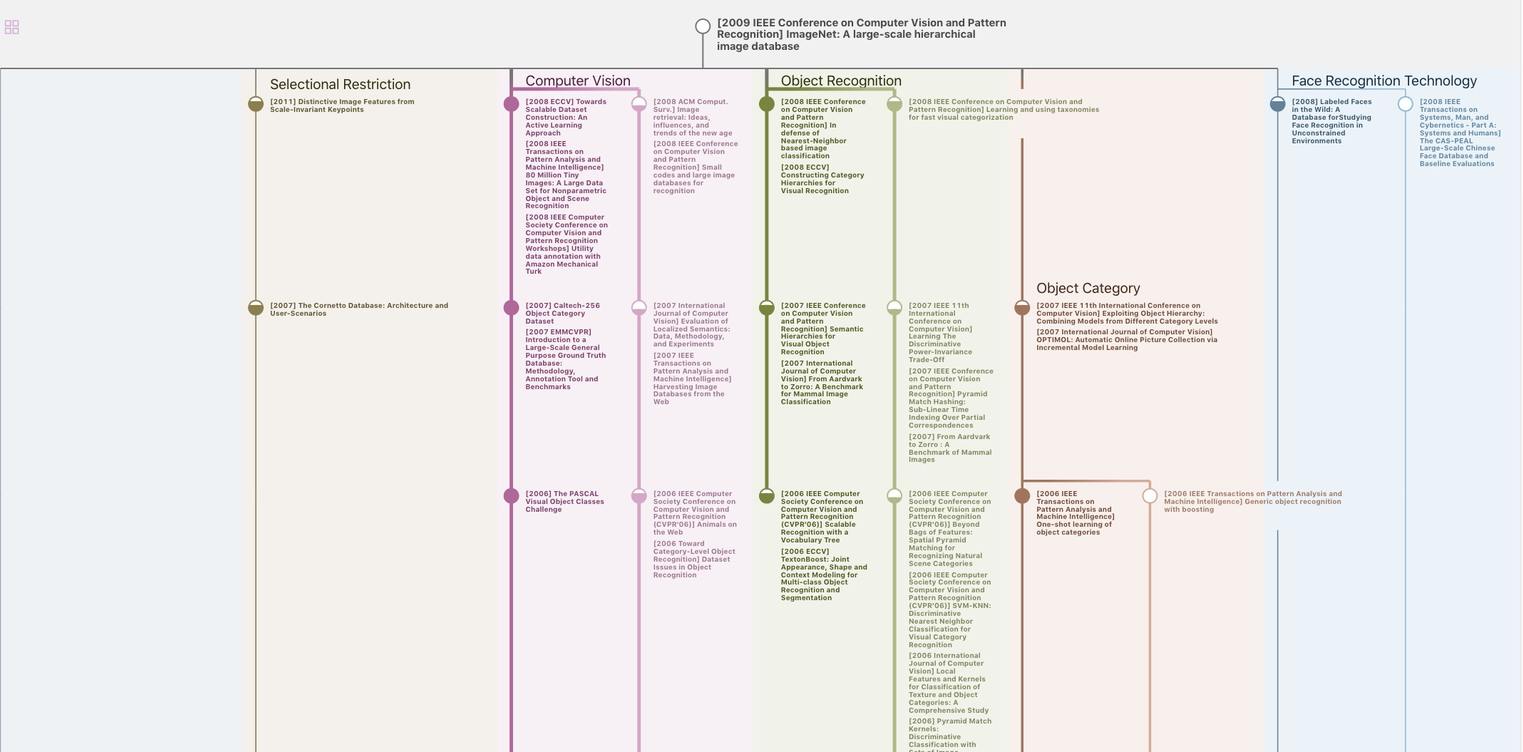
生成溯源树,研究论文发展脉络
Chat Paper
正在生成论文摘要