Veridicity annotation in the lexicon? A look at factive adjectives
meeting of the association for computational linguistics(2013)
摘要
In this note, we look at the factors that influence veridicity judgments with factive predicates. We show that more context factors play a role than is generally assumed. We propose to use crowd sourcing techniques to understand these factors better and briefly discuss the consequences for the association of lexical signatures with items in the lexicon. 1 Veridicity: what and why Recognizing the inferential properties of constructions and of lexical items is important for NLU (Natural Language Understanding) systems. In this paper we look at FACTUAL INFERENCES, inferences that allow the reader to conclude that an event has happened or will happen or that a state of affairs pertains or will pertain. We will refer to events and states together as SOAs. Factuality is in the world and outside of the text. In cases where the reader has no direct perceptual knowledge about the SOAs, she has to evaluate the factuality of a SOA referred to in a text based on her decoding of the author’s representation of the factuality of the SOA and on her knowledge about the world and about the author’s reliability. Authors have a plethora of means to signal whether they want to present SOAs as factual, as having happened or going to happen or as being more or less probable, possible, unlikely or not factual at all. We will call this presentation of a SOA the VERIDICITY of a SOA. We will call the reader’s interpretation of the author’s intention, the RIV (READER INFERRED VERIDICITY) and the reader judgment about the factuality of a SOA, RIF (READER INFERRED FACTUALITY). Annotation can, at its best, only provide us with RIVs as the author is typically not available for consultation. This leads to a methodological problem. A reader will in his interpretation of a sentence be sensitive, not only to the way an author signals her intentions but also to what he knows about the world. To circumvent this problem as much as possible, corpus annotation for veridicity is typically done by trained annotators with extensive guidelines (see e.g. (Sauri, 2008), (Sauri and Pustejovsky, 2012)) but corpus annotation by trained annotators is an expensive enterprise, hence looks at a limited number of cases. For instance, to anticipate on a case we will discuss later in the paper, lucky occurs only once in the FactBank ((Sauri and Pustejovsky, 2009). Given that annotation is done on running text, it is also difficult to avoid that the reader’s evaluation of the wider extralinguistic context might still play a role. We propose to supplement corpus annotation with crowd sourcing experiments. In these, sentences are presented to Mechanical Turk workers in limited contexts, very similar to the contexts in which linguists judge the effect of the contribution of a lexical item or a construction. But contrary to linguistic practice, we derive our examples from really occurring ones culled from the web and, more importantly, present them to many native speakers (typically 100) and in different variations to explore factors that can influence the interpretation. This kind of variation is very difficult to find in naturally occurring corpora of the type that are used for annotations (e.g. FactBank). This type of study comple-
更多查看译文
AI 理解论文
溯源树
样例
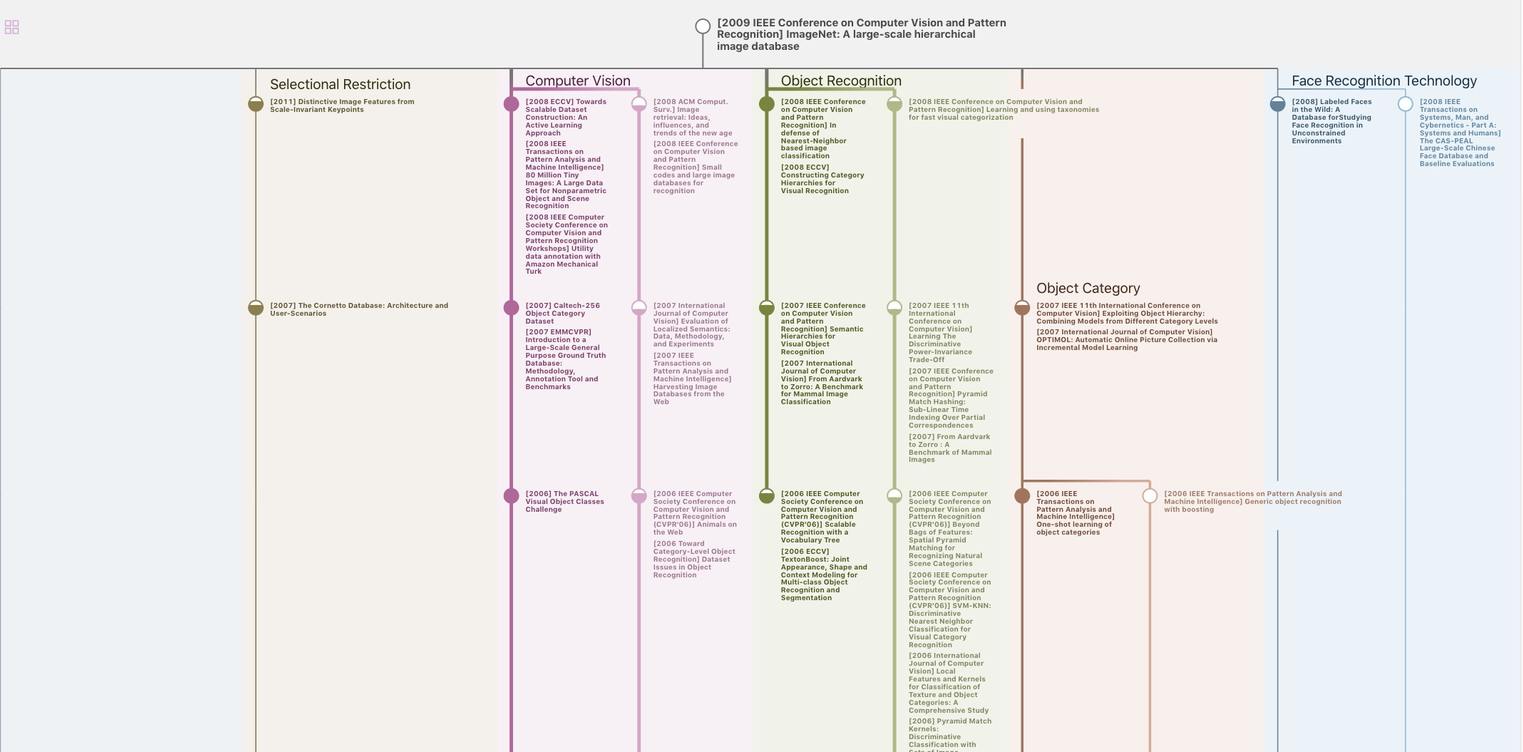
生成溯源树,研究论文发展脉络
Chat Paper
正在生成论文摘要