Feature Selection For Tree Species Identification In Very High Resolution Satellite Images
2011 IEEE INTERNATIONAL GEOSCIENCE AND REMOTE SENSING SYMPOSIUM (IGARSS)(2011)
摘要
The aim of this study was to provide an effective feature selection for tree species classifiers in mixed-species boreal forest, from a very high resolution optical satellite image. The 35 input features were the 5 input spectral bands (multispectral and panchromatic channels), 9 contextual features derived from the panchromatic channel and 21 segment-wise features computed at three segment sizes around the treetop locations. A variable ranking was first performed to evaluate the relevance of each feature. Then sequential forward selection was carried out using k-nearest neighbors (kNN) and Linear Discriminant Analysis classifiers. The results suggested that a reasonable feature set would contain 6 to 10 features, mostly from input bands and contextual features. On such a feature set, the best kNN classifier (k=5) returned classification accuracies of 76% for pine and spruce and 88% for decidous trees, with RMS errors between 1.4% and 3.5% and few mixing with the 4 non-tree classes.
更多查看译文
关键词
image segmentation,k nearest neighbor,forestry,feature extraction,accuracy,feature selection,boreal forest,vegetation,satellites
AI 理解论文
溯源树
样例
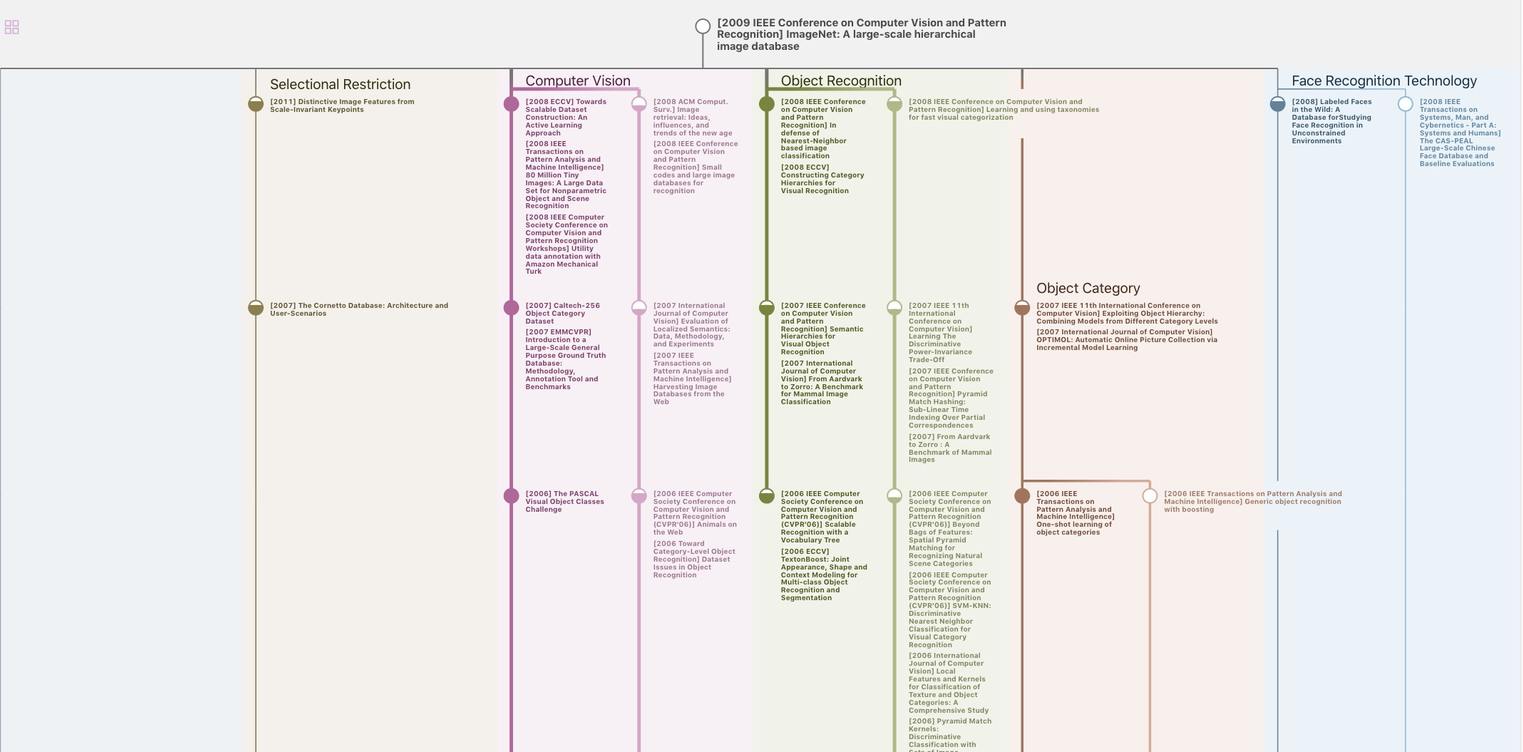
生成溯源树,研究论文发展脉络
Chat Paper
正在生成论文摘要