Community Detection In Large-Scale Networks: A Survey And Empirical Evaluation
WILEY INTERDISCIPLINARY REVIEWS-COMPUTATIONAL STATISTICS(2014)
摘要
Community detection is a common problem in graph data analytics that consists of finding groups of densely connected nodes with few connections to nodes outside of the group. In particular, identifying communities in large-scale networks is an important task in many scientific domains. In this review, we evaluated eight state-of-the-art and five traditional algorithms for overlapping and disjoint community detection on large-scale real-world networks with known ground-truth communities. These 13 algorithms were empirically compared using goodness metrics that measure the structural properties of the identified communities, as well as performance metrics that evaluate these communities against the ground-truth. Our results show that these two types of metrics are not equivalent. That is, an algorithm may perform well in terms of goodness metrics, but poorly in terms of performance metrics, or vice versa. (C) 2014 The Authors. WIREs Computational Statistics published byWiley Periodicals, Inc.
更多查看译文
关键词
clustering, community detection, empirical evaluation, graphs, ground-truth, networks
AI 理解论文
溯源树
样例
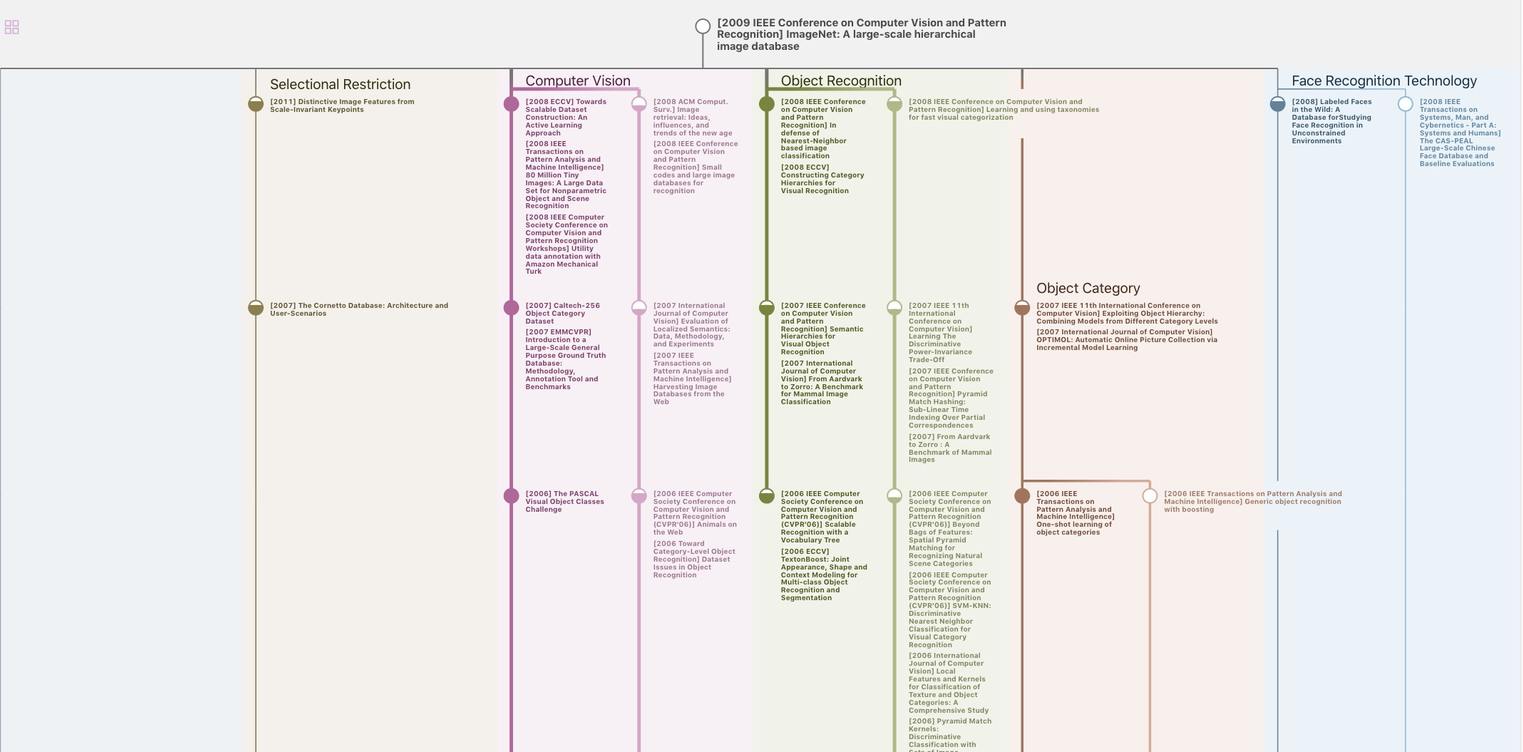
生成溯源树,研究论文发展脉络
Chat Paper
正在生成论文摘要