The role of margins in boosting and ensemble performance
Periodicals(2014)
摘要
AbstractBoosting refers to methods that create a sequence of classifiers that perform at least slightly better than random (weak learners) and combine them into a highly accurate ensemble model (strong learners) through weighted voting. There is sufficient empirical evidence to suggest that the performance of boosting methods is superior to that of individual classifiers. In the bias–variance decomposition framework, it has been demonstrated that boosting algorithms typically reduce bias for learning problems, and in some instances reduce the variance. In addition, even when combining a large number of weak learners, boosting algorithms can be very robust to overfitting, in most instances having lower generalization error than other competing ensemble methodologies, such as bagging and random forests. To explain the successful performance of boosting methods, Schapire et al. (Boosting the margin: a new explanation for the effectiveness of voting methods. Ann Stat 1998, 26:1651–1686) developed a bound based on the margins of the training data, from which they concluded that larger margins should lead to lower generalization error. In this article, we examine the role of margins in boosting and ensemble method performance. WIREs Comput Stat 2014, 6:124–131. doi: 10.1002/wics.1292This article is categorized under:1Statistical and Graphical Methods of Data Analysis > Bootstrap and Resampling2Statistical Learning and Exploratory Methods of the Data Sciences > Classification and Regression Trees (CART)
更多查看译文
关键词
generalization error, ensemble performance, bagging, random forests, large margin classifiers
AI 理解论文
溯源树
样例
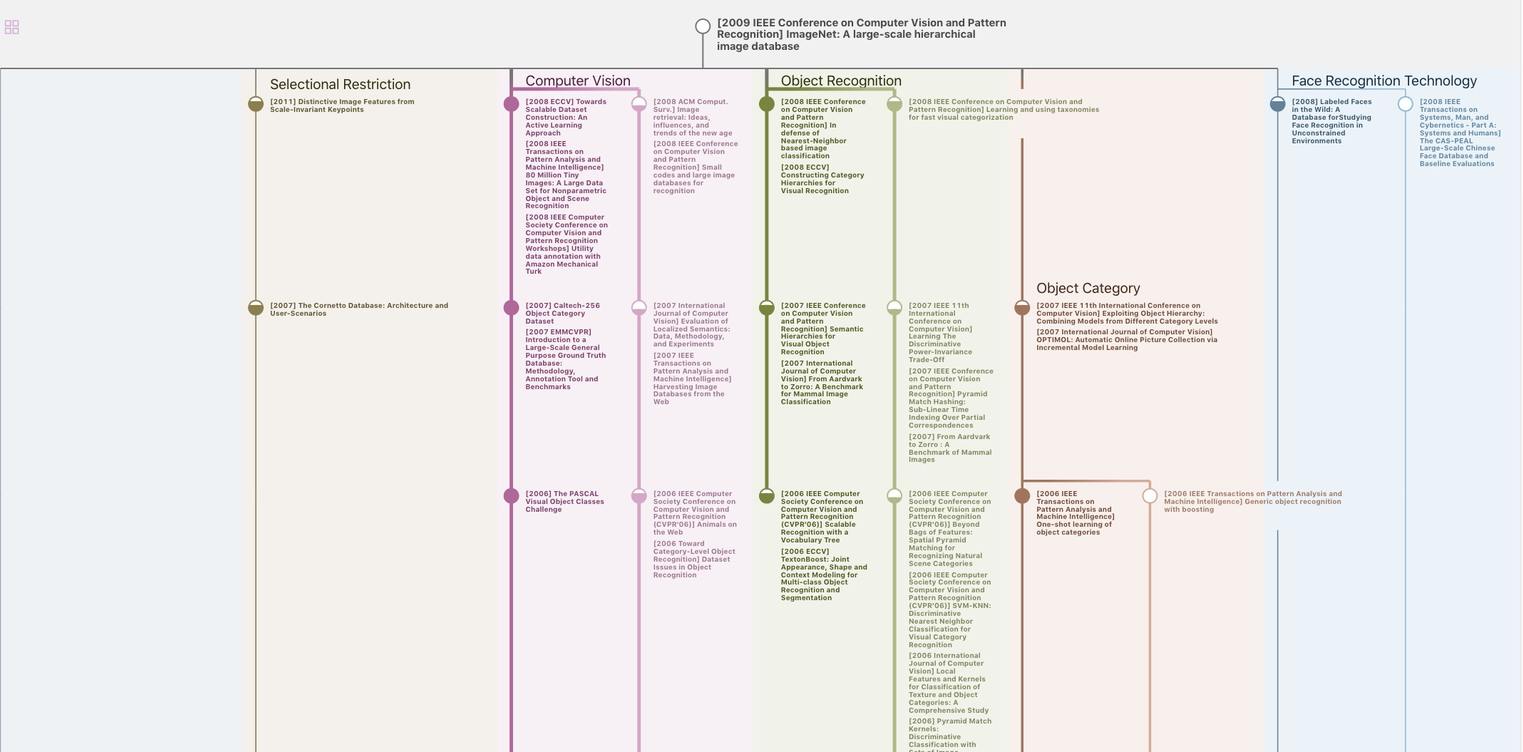
生成溯源树,研究论文发展脉络
Chat Paper
正在生成论文摘要