Assimilating radar for observations in a bird migration model
Topics in Catalysis(2004)
摘要
In this study we investigate the possiblities to predict north-south autumn bird migration over the Netherlands by combining predictions from a dynamic spatially distributed model (an extended version of Erni et al. (1)) and radar observations at several locations in Europe. The model is initialised with a virtual population of birds leav- ing Scandinavia. Time-variable model inputs are wind speed and direction, and constant inputs are the terrain and habitat quality over Europe. The main process underlying the complex patterns of migratory flight is the bird's energy balance. We link the bird-migration model to an Ensemble Kalman filter, a Reduced Rank Square Root filter and combinations of these - using the algorithms as described and implemented by Verlaan and Heemink (2). In our study we use real weather and terrain data, but artificial radar observations. We first investigate the be- haviour of our predictions, by varying density and location of our radar observation stations (with constant, white observation errors). Next, we extend our analysis to the more realistic situation where the radar measurement error depends on both bird densities (especially during migration peaks) and weather conditions (especially rainfall). The trade-offs between model error - measurement error - measurement density and predictive uncertainty are described for different weather conditions. Future work will concentrate on understanding the structure of model errors, and also on using data sources complementary to the radar observations such as visual counts of birds at resting places or ringing data. These observations suffer not from state-dependent observation errors, but are on the other hand less easily linked to the model scale. References
更多查看译文
关键词
ensemble kalman filter,measurement error,wind speed,energy balance,model error
AI 理解论文
溯源树
样例
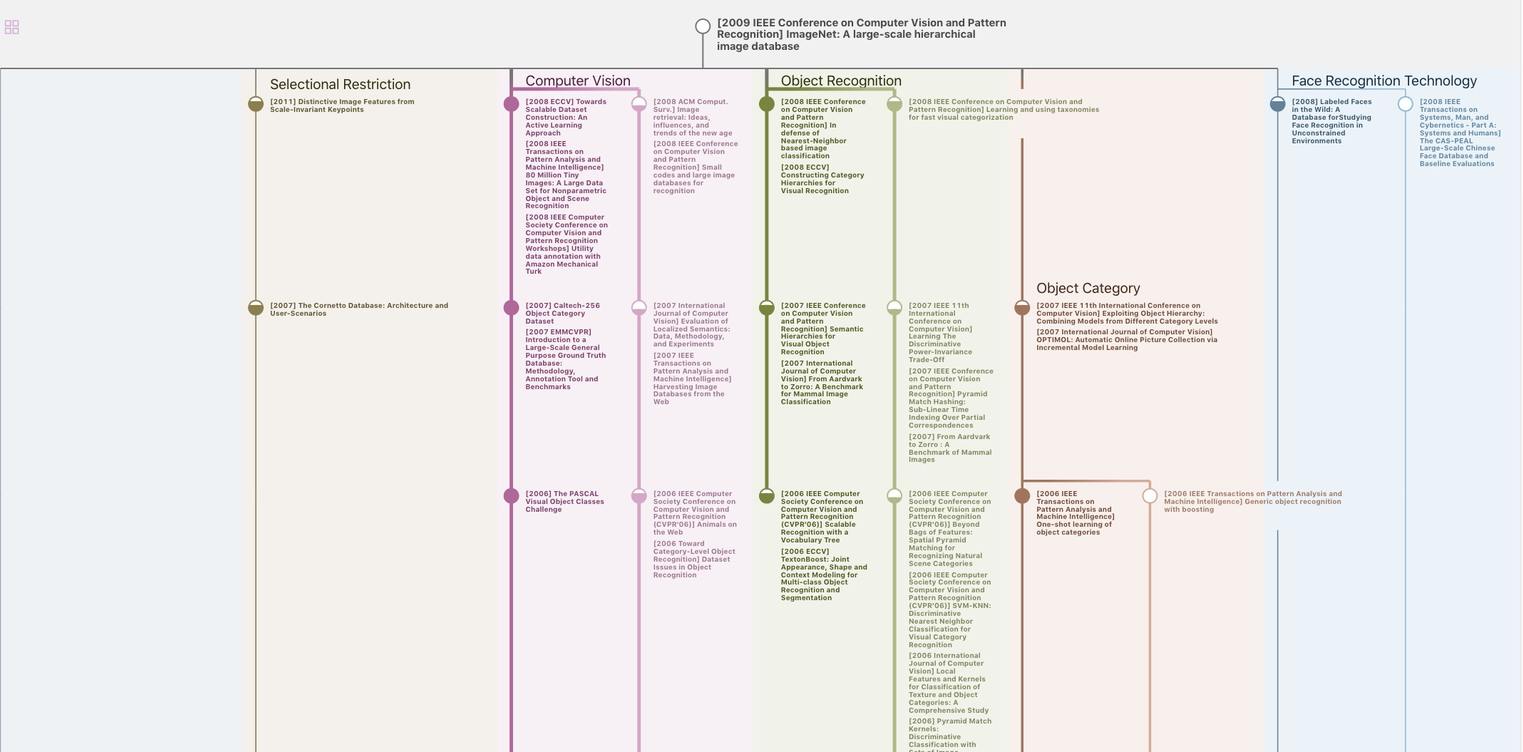
生成溯源树,研究论文发展脉络
Chat Paper
正在生成论文摘要