Going beyond a First Reader: A Machine Learning Methodology for Optimizing Cost and Performance in Breast Ultrasound Diagnosis
Ultrasound in Medicine & Biology(2015)
摘要
The goal of this study was to devise a machine learning methodology as a viable low-cost alternative to a second reader to help augment physicians' interpretations of breast ultrasound images in differentiating benign and malignant masses. Two independent feature sets consisting of visual features based on a radiologist's interpretation of images and computer-extracted features when used as first and second readers and combined by adaptive boosting (AdaBoost) and a pruning classifier resulted in a very high level of diagnostic performance (area under the receiver operating characteristic curve = 0.98) at a cost of pruning a fraction (20%) of the cases for further evaluation by independent methods. AdaBoost also improved the diagnostic performance of the individual human observers and increased the agreement between their analyses. Pairing AdaBoost with selective pruning is a principled methodology for achieving high diagnostic performance without the added cost of an additional reader for differentiating solid breast masses by ultrasound.
更多查看译文
关键词
Breast ultrasound,Breast cancer,Adaptive boosting,Computer-aided diagnosis,Artificial intelligence
AI 理解论文
溯源树
样例
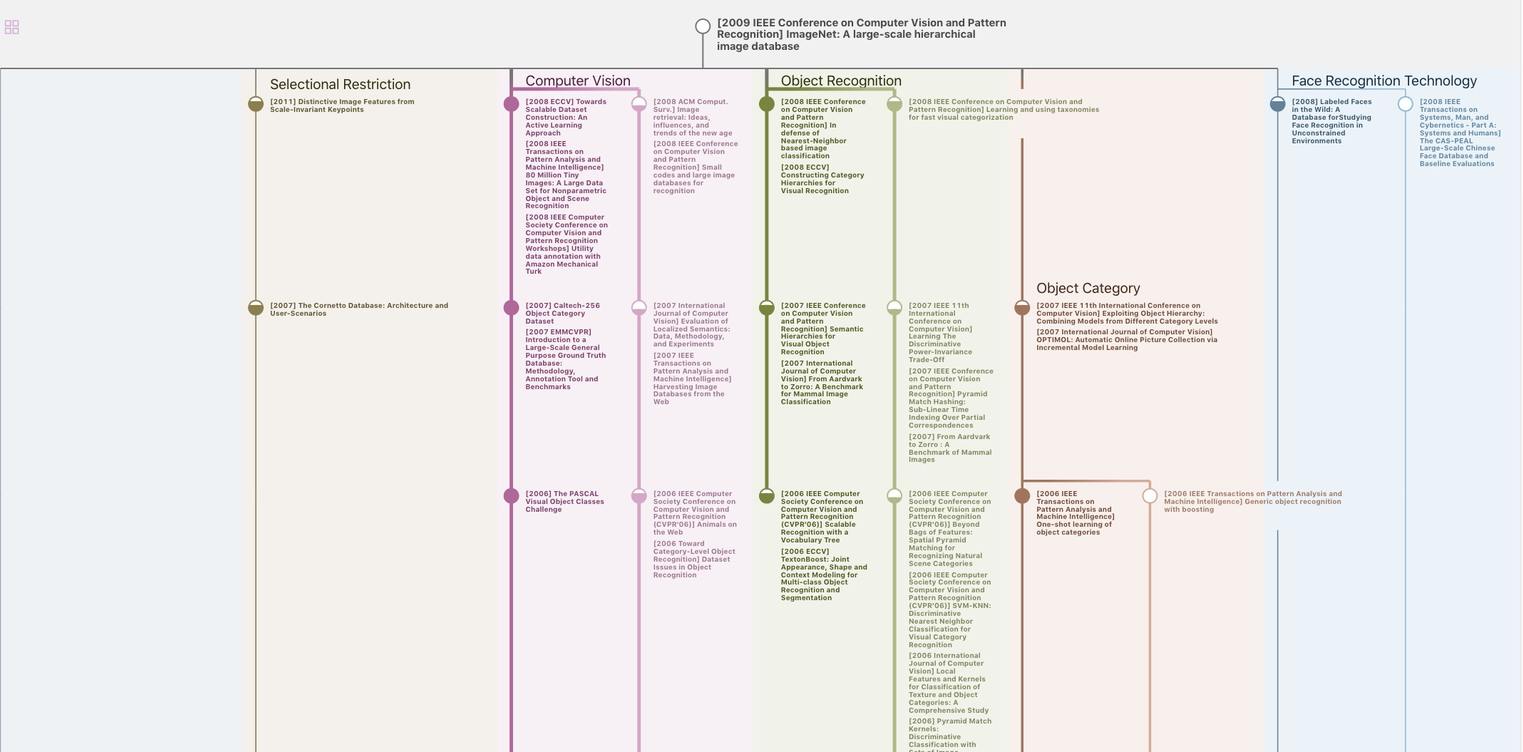
生成溯源树,研究论文发展脉络
Chat Paper
正在生成论文摘要