Abstract PR1: Integrating expression data improves mutational significance analysis of lung squamous carcinoma
CLINICAL CANCER RESEARCH(2012)
摘要
In contrast to lung adenocarcinoma in which a driving and potentially targetable genetic aberration can be identified in excess of 50% of tumors, only 10% can be found in squamous cell lung cancer, the second most common type of lung cancer, none of which are druggable by an approved targeted therapy. Our limited knowledge regarding targetable genetic alterations in squamous cell lung cancer is the result of a lack of detailed genomic study of this disease and the lack of experimental validation of identified putative therapeutic targets. We have analyzed exome sequencing data to identify novel driving mutations in 184 lung squamous cell carcinomas using previously successful methods (Integrated genomic analyses of ovarian carcinoma, Nature 2011), but these analyses are confounded by an unusually high mutation rate, 8-9/Mb on average, some reaching as many as 80/Mb. Many of the most significantly mutated genes in the analysis are unlikely related to the pathogenesis of the disease based on a lack of expression in lung and known role in cancer, suggesting that genes which are not expressed (or minimally expressed) escape negative selection and result in a higher mutation density as compared with expressed genes. Here, we present a method integrating expression and mutation data in order to more accurately determine the significance of a mutated gene based on the expression subclass in which it falls and the frequency at which it9s mutated relative to our expectation; unexpressed genes are likely much more tolerant of mutations and should therefore have a higher expected background mutation rate. Analyses of this kind have removed many of the genes putatively irrelevant in this cancer type and have identified many biologically plausible novel genes that to date are unlinked with lung squamous cell carcinoma. We believe this method refines an already powerful analysis for identifying significantly mutated genes in cancer with a particularly high potential for separating signal and noise in the most highly mutated cancer types. This abstract is also presented as Poster A17.
更多查看译文
AI 理解论文
溯源树
样例
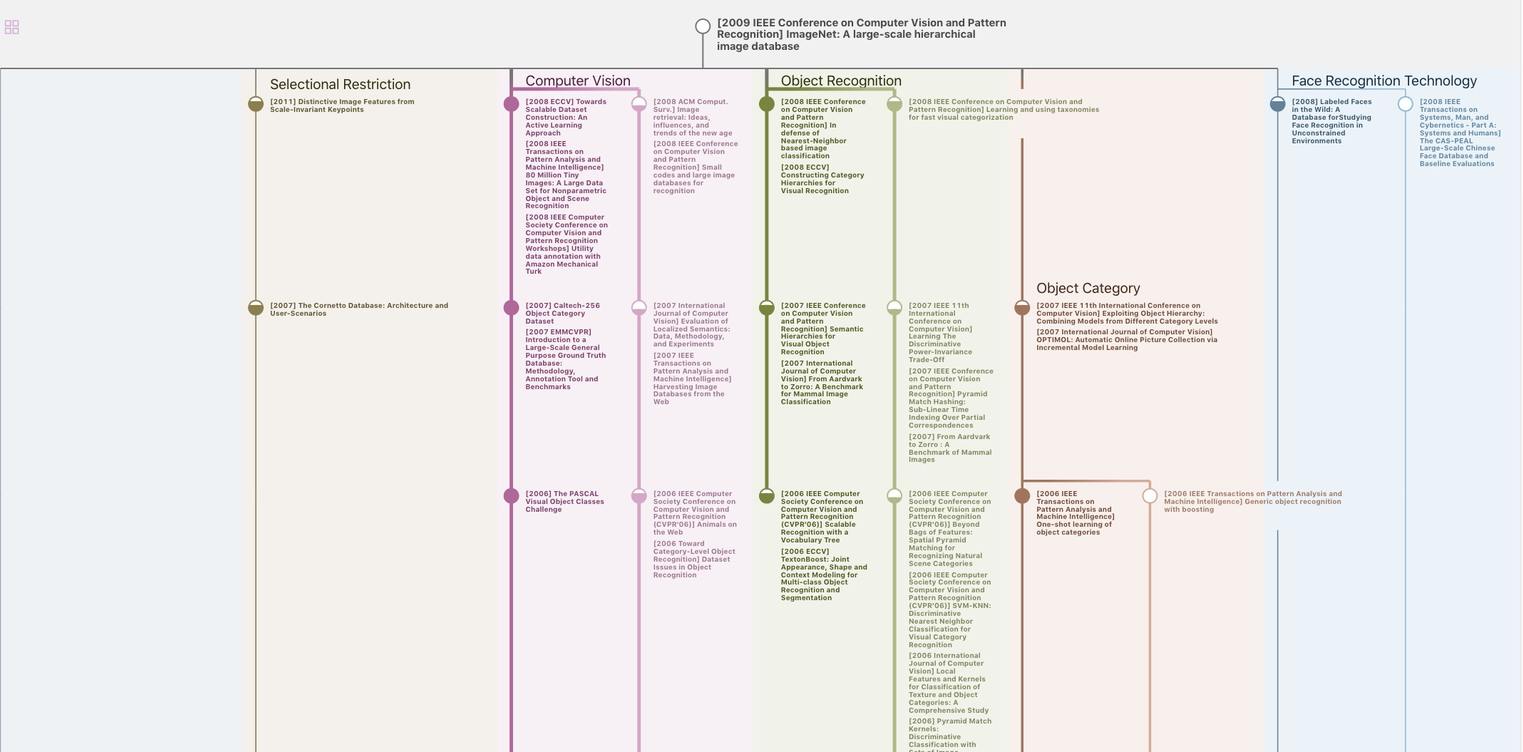
生成溯源树,研究论文发展脉络
Chat Paper
正在生成论文摘要