A Game-Theoretic Approach for Improving Generalization Ability of TSP Solvers
arXiv (Cornell University)(2021)
摘要
In this paper, we introduce a two-player zero-sum framework between a trainable \emph{Solver} and a \emph{Data Generator} to improve the generalization ability of deep learning-based solvers for Traveling Salesman Problem (TSP). Grounded in \textsl{Policy Space Response Oracle} (PSRO) methods, our two-player framework outputs a population of best-responding Solvers, over which we can mix and output a combined model that achieves the least exploitability against the Generator, and thereby the most generalizable performance on different TSP tasks. We conduct experiments on a variety of TSP instances with different types and sizes. Results suggest that our Solvers achieve the state-of-the-art performance even on tasks the Solver never meets, whilst the performance of other deep learning-based Solvers drops sharply due to over-fitting. To demonstrate the principle of our framework, we study the learning outcome of the proposed two-player game and demonstrate that the exploitability of the Solver population decreases during training, and it eventually approximates the Nash equilibrium along with the Generator.
更多查看译文
关键词
tsp
AI 理解论文
溯源树
样例
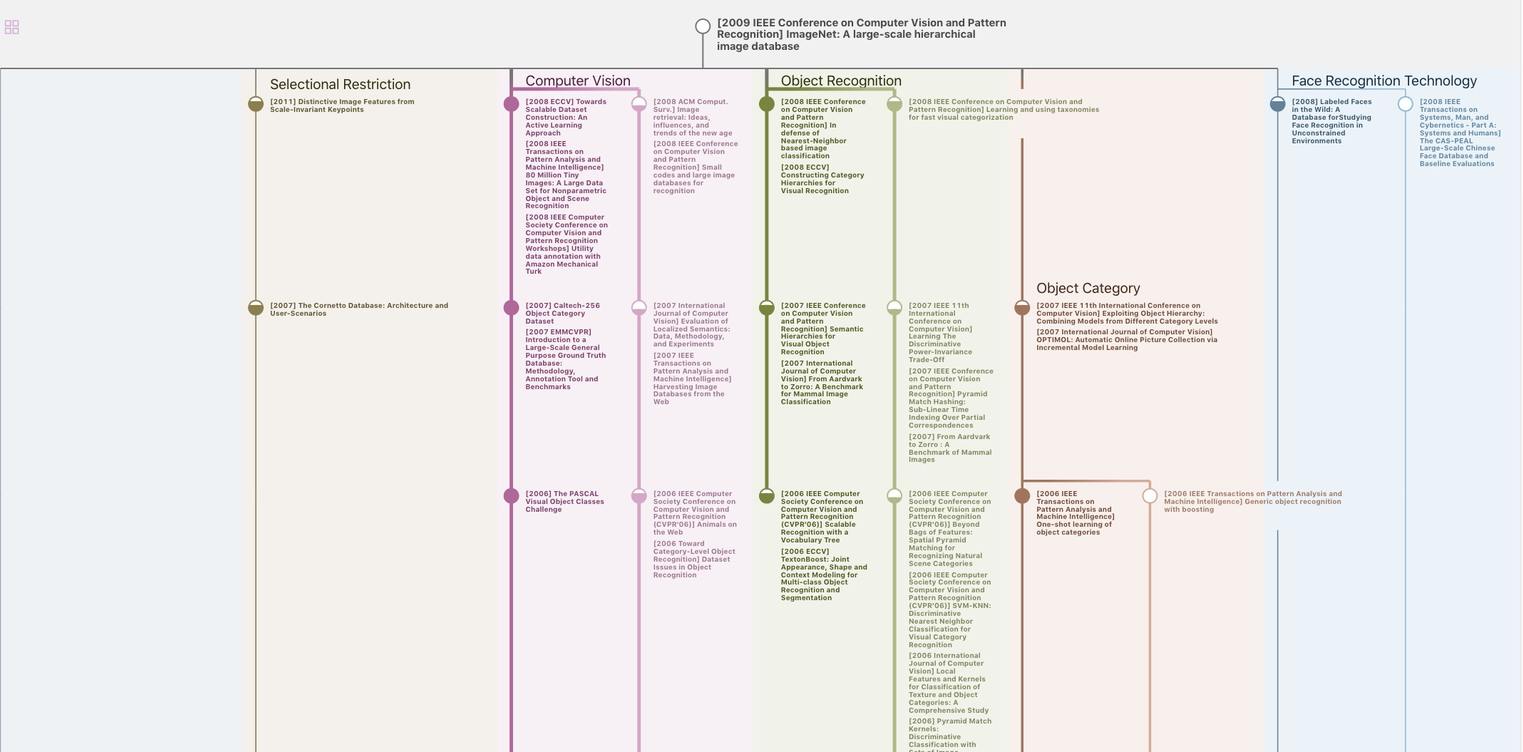
生成溯源树,研究论文发展脉络
Chat Paper
正在生成论文摘要