A Learning Scheme For Emg Based Interfaces: On Task Specificity In Motion Decoding Domain
NEURO-ROBOTICS: FROM BRAIN MACHINE INTERFACES TO REHABILITATION ROBOTICS(2014)
摘要
A complete learning scheme for EMG based interfaces is used to discriminate between different reach to graspmovements in 3D space. The proposed scheme is able to decode human kinematics, using the myoelectric activity captured from human upper arm and forearm muscles. Three different task features can be distinguished: subspace to move towards, object to be grasped and task to be executed (with the grasped object). The discrimination between the different reach to grasp movements is accomplished with a random forest classifier. The classification decision triggers task-specific motion decoding models that outperform "general" models, providing better estimation accuracy. The proposed learning scheme takes advantage of both a classifier and a regressor, that cooperate advantageously in order to split the space, confronting with task specificity, the nonlinear relationship between the EMG signals and the motion to be estimated. The proposed scheme can be used for a series of EMG-based interfaces, ranging from EMG based teleoperation of robot arm hand systems to muscle computer interfaces and EMG controlled neuroprosthetic devices.
更多查看译文
关键词
ElectroMyoGraphy (EMG),EMG based interfaces,Learning scheme,Task specificity
AI 理解论文
溯源树
样例
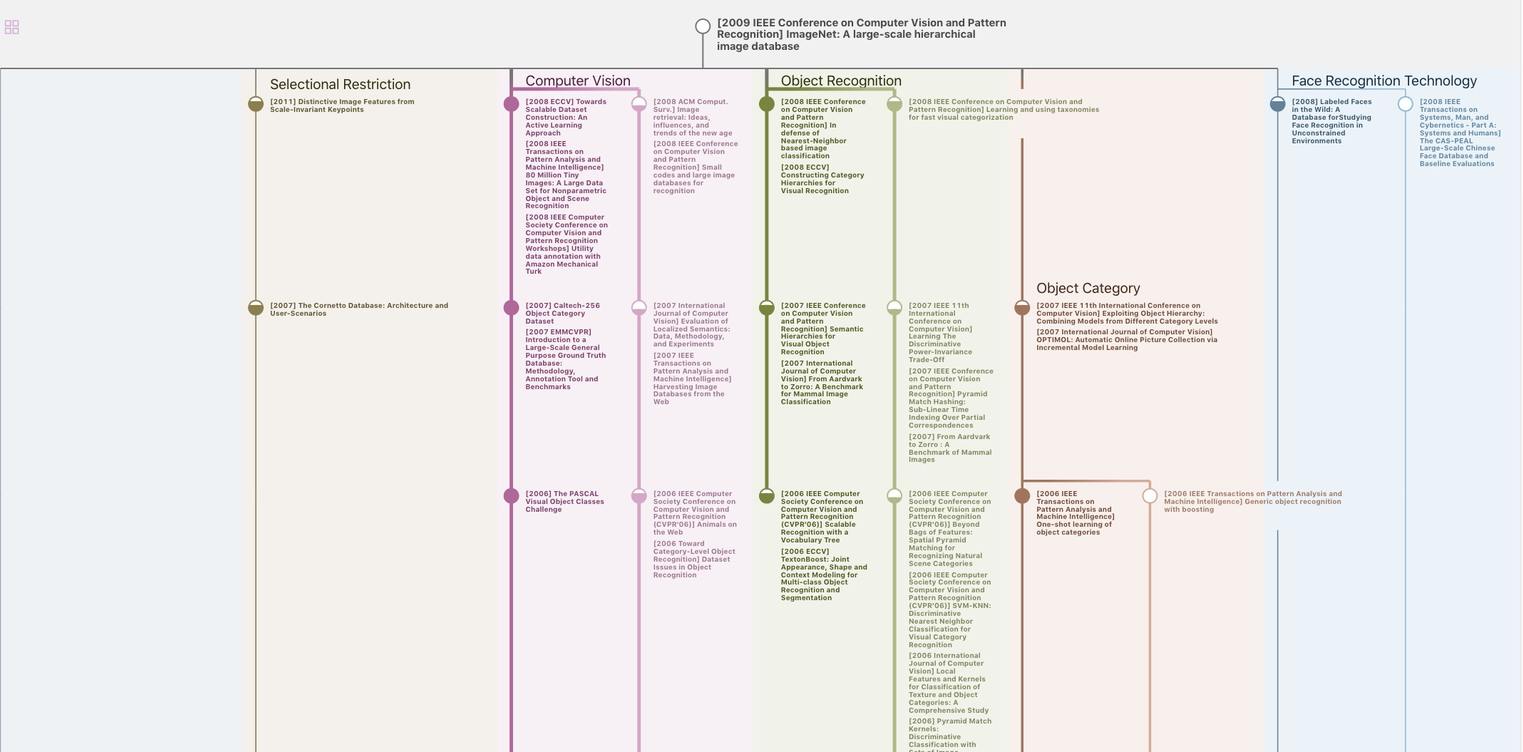
生成溯源树,研究论文发展脉络
Chat Paper
正在生成论文摘要