Predictive Subset VAR Modeling Using the Genetic Algorithm and Information Complexity
European Journal of Pure and Applied Mathematics(2010)
摘要
Can we use lagged values of major stock market indices to provide useful predictions as a standard vector autoregressive model? Underlying this application, of course, is the question of finding a vector autoregressive model which makes accurate and efficient forecasts. In this paper, we use the Genetic Algorithm with information complexity criteria as the fitness function to drive subset selection and parameter estimation. In the testing period when the target index lost more than 15%, the identified subset VAR model gained over 17%. The prediction error bands built around the forecasts are half as wide as those obtained by the saturated model. Using both simulation and application studies, we present evidence that even when the typical regres- sion assumptions seem to be met, the VAR model is misspecified. 2000 Mathematics Subject Classifications: 62HXX,62MXX,91BXX
更多查看译文
关键词
model selection,robustness
AI 理解论文
溯源树
样例
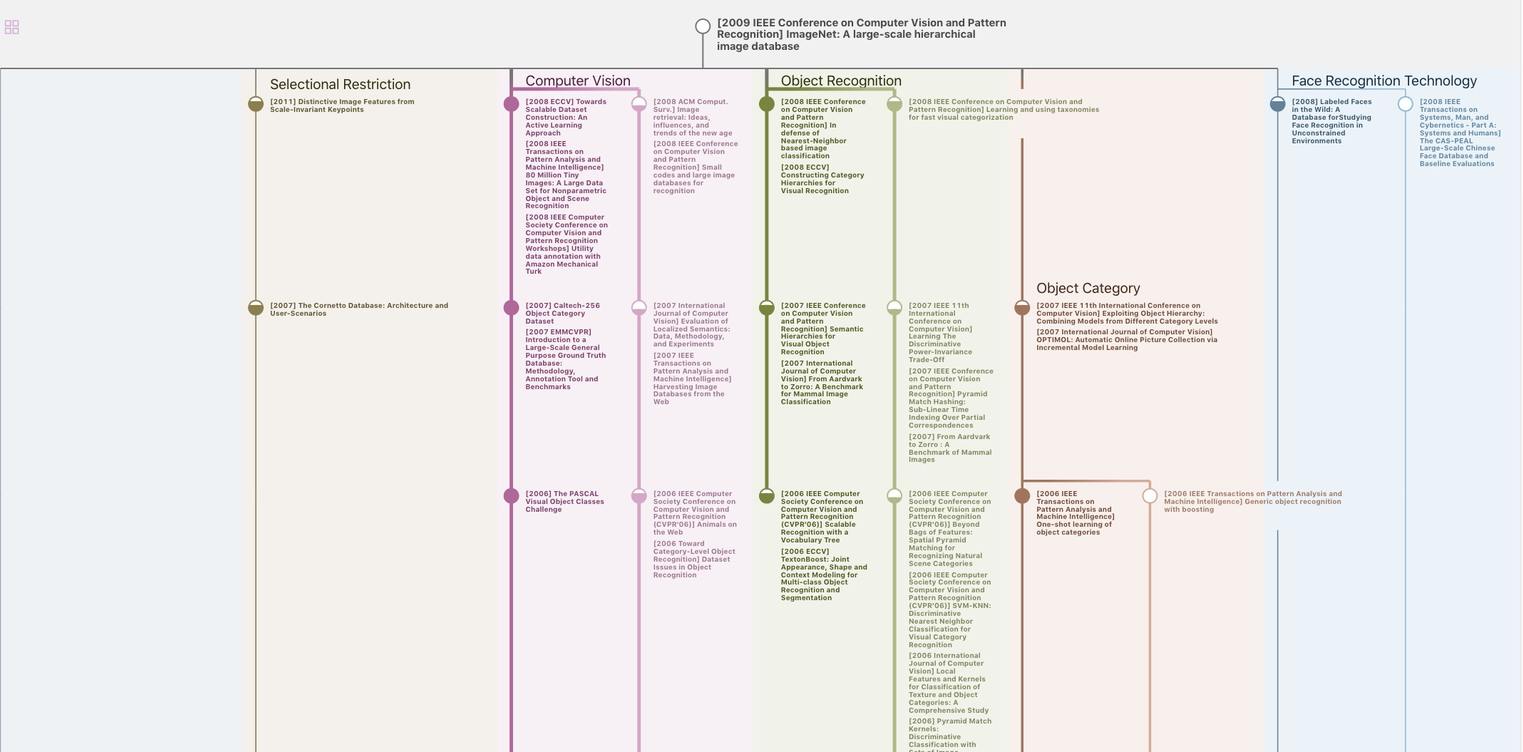
生成溯源树,研究论文发展脉络
Chat Paper
正在生成论文摘要