Exploiting Multiview Properties In Semi-Supervised Video Classification
2012 SIXTH INTERNATIONAL SYMPOSIUM ON TELECOMMUNICATIONS (IST)(2012)
摘要
In large databases, availability of labeled training data is mostly prohibitive in classification. Semi-supervised algorithms are employed to tackle the lack of labeled training data problem. Video databases are the epitome for such a scenario; that is why semi-supervised learning has found its niche in it. Graph-based methods are a promising platform for semi-supervised video classification. Based on the multiview characteristic of video data, different features have been proposed (such as SIFT, STIP and MFCC) which can be utilized to build a graph. In this paper, we have proposed a new classification method which fuses the results of manifold regularization over different graphs. Our method acts like a co-training method with respect to its iterative nature which tries to find the labels of unlabeled data during each iteration, but unlike co-training methods it takes into account the unlabeled data in classification procedure. The fusion is done after manifold regularization with a ranking method which makes the algorithm to be competitive with supervised methods. Our experimental results run on the CCV database show the efficiency of the proposed method.
更多查看译文
关键词
Semi-supervised learning, manifold, regularization, co-training, video classification, multiview features
AI 理解论文
溯源树
样例
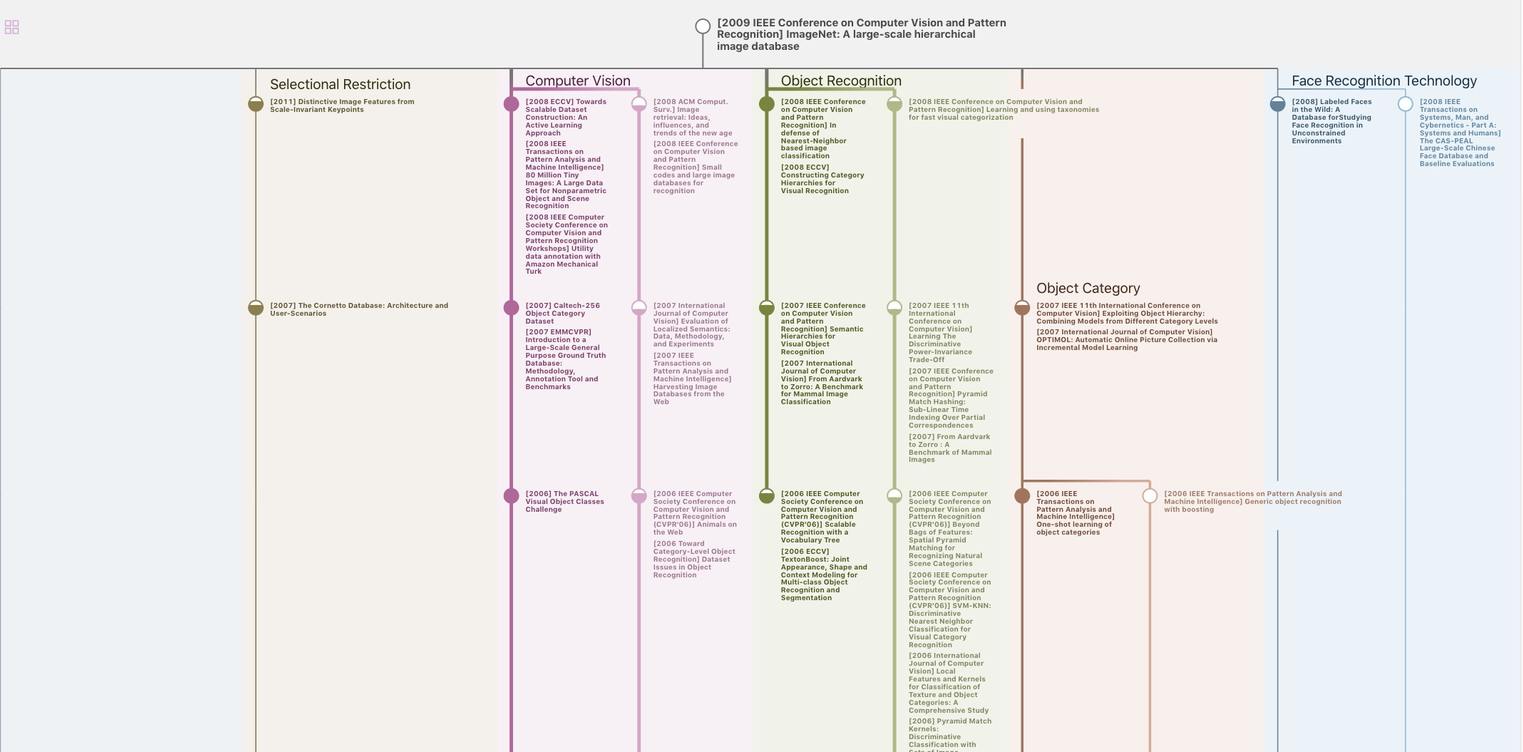
生成溯源树,研究论文发展脉络
Chat Paper
正在生成论文摘要