Driver Familiarity Modeling For Generating Navigation Directions
2013 16TH INTERNATIONAL IEEE CONFERENCE ON INTELLIGENT TRANSPORTATION SYSTEMS - (ITSC)(2013)
摘要
Current and future in-vehicle personal assistant systems stand to benefit from knowledge of the level of familiarity that the driver has with different parts of the current route. Such systems could use this information to adapt driving directions to be more succinct, understandable and personalized. However accumulating evidence of familiarity by direct experience alone could take many months and still be incomplete. Instead, we propose building predictive models of driver familiarity with road networks by generalizing intelligently from a small sample of data. Inspired by psychology studies that suggest a variety of cognitive models of route familiarity in humans, we present an ensemble of familiarity models using different machine learning methods on GPS time series data collected during normal driving by a volunteer pool of 22 drivers from San Francisco Bay area. We validate the models' predictions through an extensive questionnaire administered to the subjects about route familiarity and need for directions along select routes. Our results indicate that a significant component of driver familiarity can be predicted from unobtrusively collected driving data. We conclude with a proposed approach for integrating such models with a generator of driving directions.
更多查看译文
关键词
learning artificial intelligence,time series,data collection,global positioning system
AI 理解论文
溯源树
样例
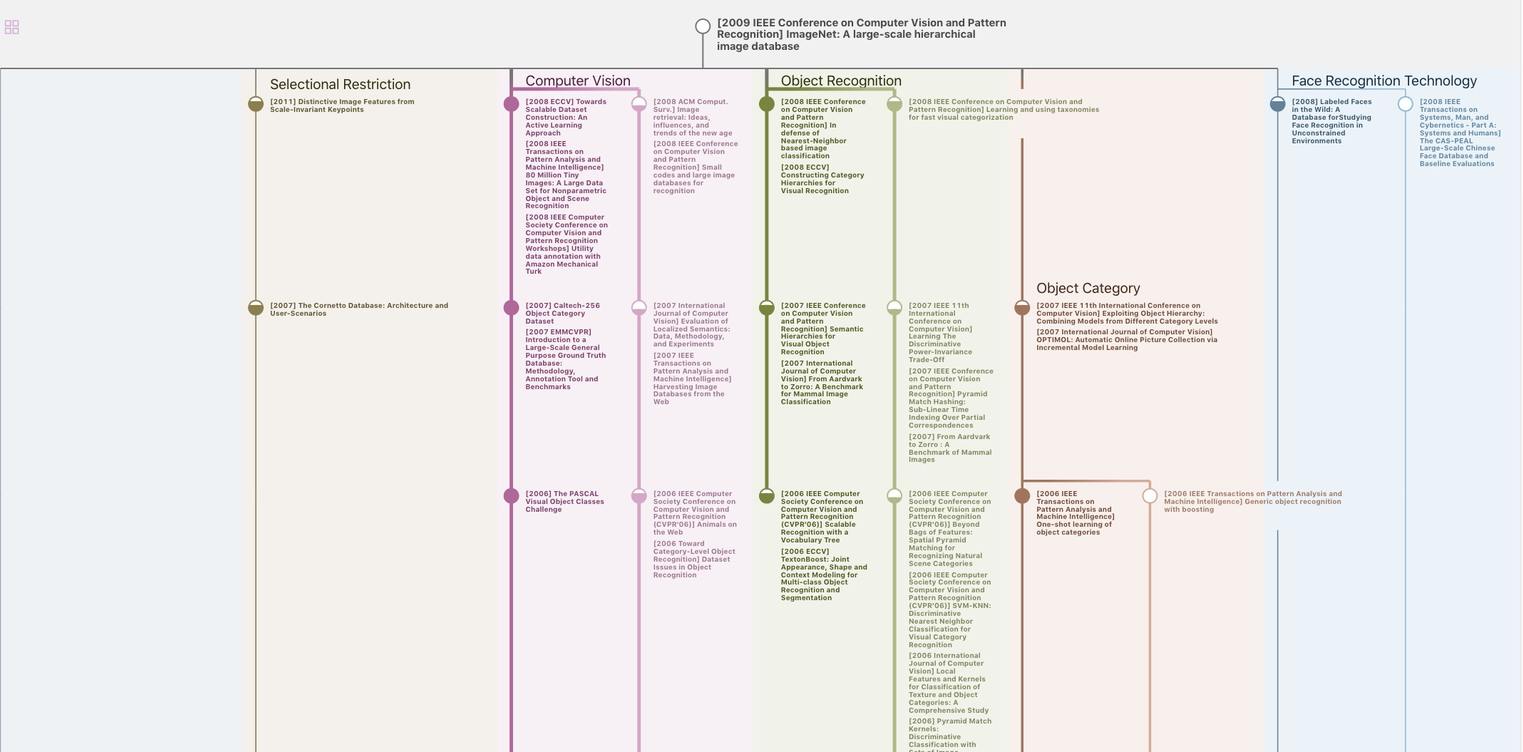
生成溯源树,研究论文发展脉络
Chat Paper
正在生成论文摘要