Transfer Learning in Hierarchical Feature Spaces
2015 10th International Conference on Intelligent Systems and Knowledge Engineering (ISKE)(2015)
摘要
Transfer learning provides an approach to solve target tasks more quickly and effectively by using previously acquired knowledge learned from source tasks. As one category of transfer learning approaches, feature-based transfer learning approaches aim to find a latent feature space shared between source and target domains. The issue is that the sole feature space can't exploit the relationship of source domain and target domain fully. To deal with this issue, this paper proposes a transfer learning method that uses deep learning to extract hierarchical feature spaces, so knowledge of source domain can be exploited and transferred in multiple feature spaces with different levels of abstraction. In the experiment, the effectiveness of transfer learning in multiple feature spaces is compared and this can help us find the optimal feature space for transfer learning.
更多查看译文
关键词
transfer learning,deep learning,feature extraction
AI 理解论文
溯源树
样例
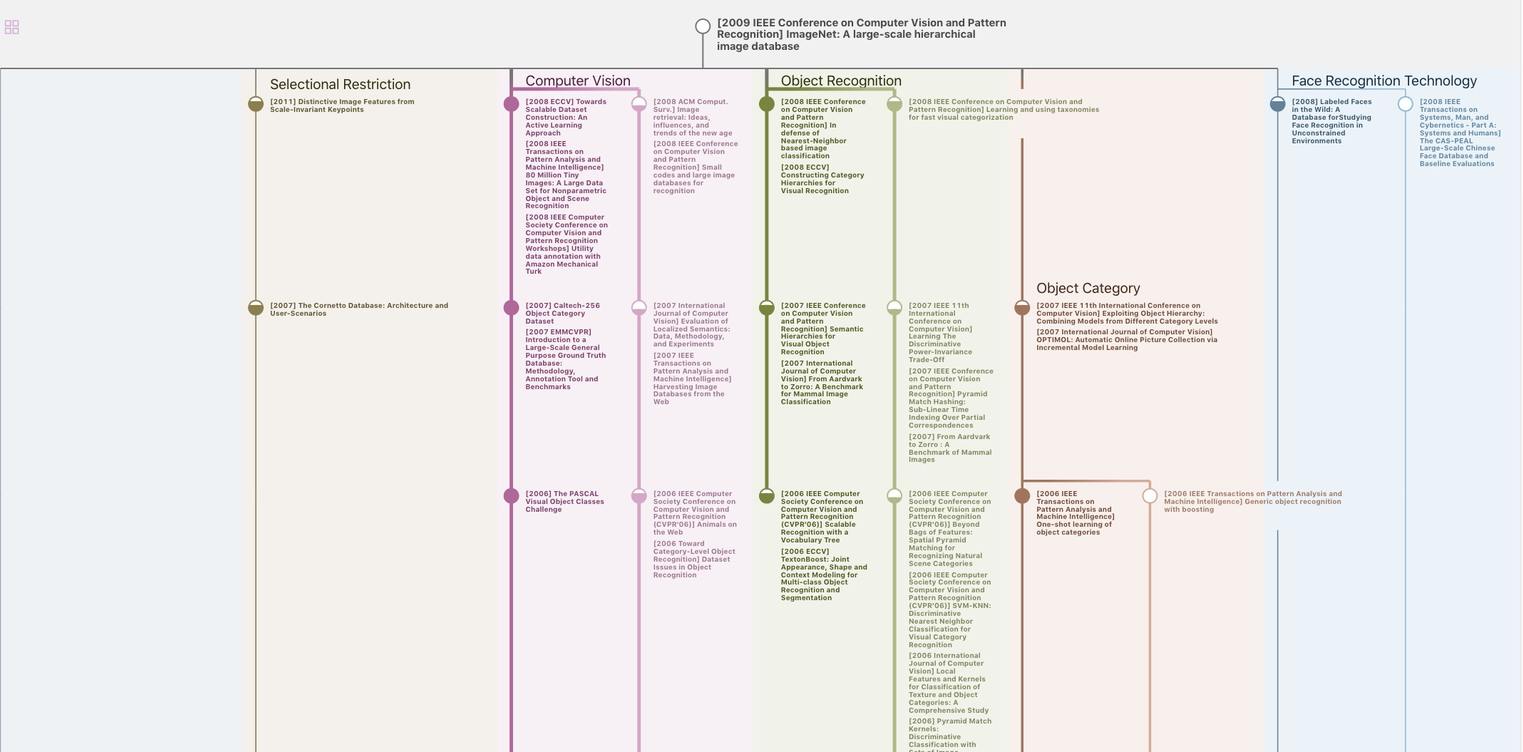
生成溯源树,研究论文发展脉络
Chat Paper
正在生成论文摘要