The Progressive Proposal Particle Filter: Better Approximations to the Optimal Importance Density
mag(2014)
摘要
The crucial step in designing a particle filter for a particular application is the choice of importance density. The optimal scheme is to use the conditional posterior density of the state, but this cannot be sampled or calculated analytically in most case. In practice, approximations of this density are used, particularly Gaussian densities based on linearisation or the unscented transform. For many highly nonlinear or non-Gaussian models, these approximations can be poor, leading to degeneracy of the particle approximation or even the filter "losing track" completely. In this paper, we develop a new mechanism for approximating the optimal importance density, which we call the progressive proposal method. This works by introducing the observation progressively and performing a series of state updates, each using a local Gaussian approximation to the optimal importance density. A number of refinements and extensions to the basic algorithm are also introduced. Simulations are used to demonstrate an improvement in performance over simpler particle filters on a number of applications.
更多查看译文
AI 理解论文
溯源树
样例
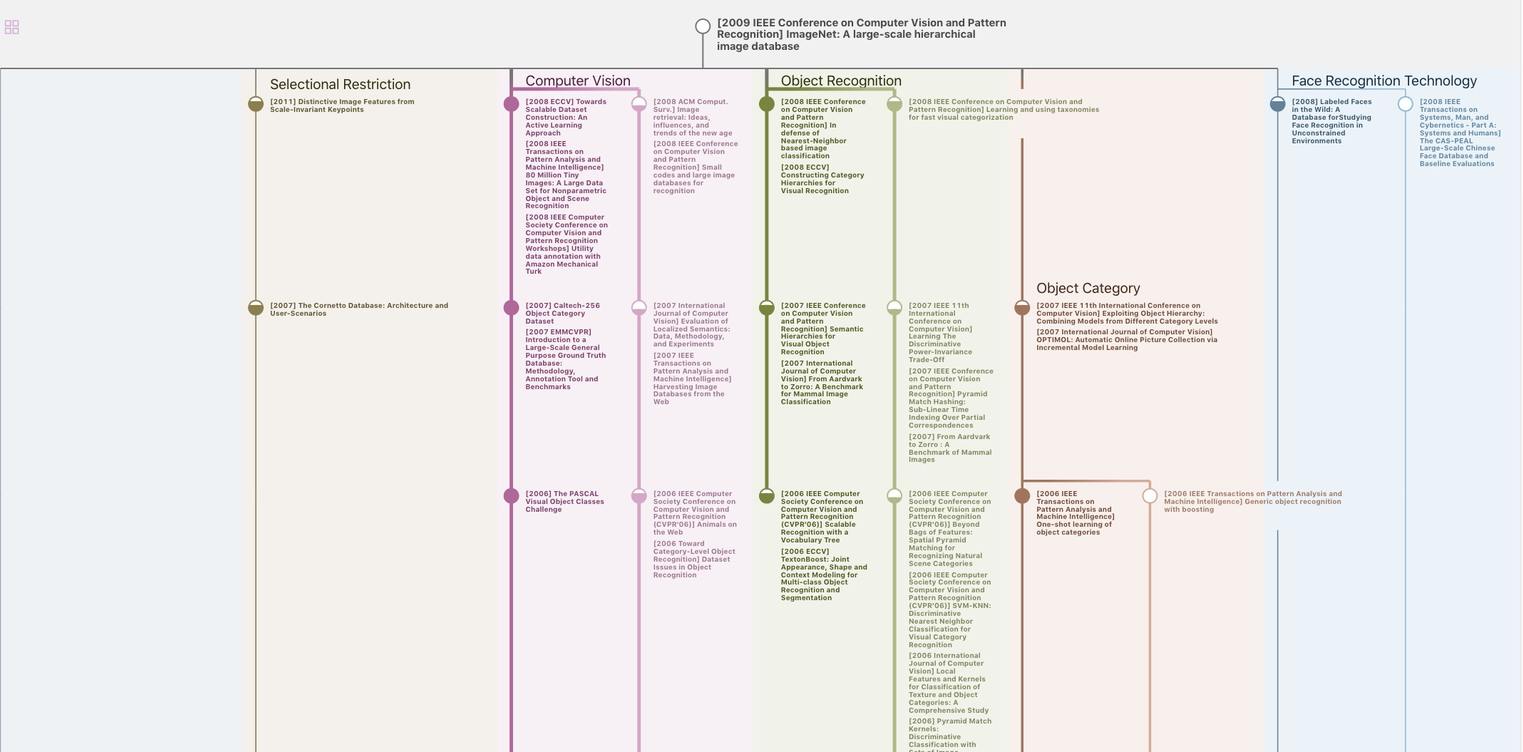
生成溯源树,研究论文发展脉络
Chat Paper
正在生成论文摘要