Local Entropy Estimation In Particle Velocity Images Using Histogram Deconvolution
IMAGE PROCESSING: ALGORITHMS AND SYSTEMS(2002)
摘要
Local entropy estimates can be useful in segmentation of Particle Image Velocimeter (PIV) images. Image intensity combined with local entropy estimates forms basis for bubble detection. The acquired images are corrupted by additive noise with fixed density function. Local entropy estimate of the original image can be extracted from the noisy image if noise distribution is known a priori. A new approach to the problem of local entropy estimation in noisy images is presented. We presume that our original image is corrupted by additive i.d.d. noise from an ergodic source. The noise thus comes from a source that has a fixed density function, which can be approximated by taking histogram over the noise image. Now the histogram of the observed image, can be approximated by convolving the histogram of the original with the noise density (or its approximation). Now, in principle, it is possible extract the histogram of the original image by a blind deconvolution and removing the effect of noise. In many cases, it is also possible to utilise a priori information of the noise process. Local histogram deconvolutions with different window sizes and histogram bin numbers are performed. It is found that with careful implementation the resulting entropy estimates improve the estimates based on noisy image. We expect that the proposed method will prove to be useful with higher dimensional input data. With multidimensional data the number samples grows rapidly with the window size which improves significantly the density estimates.
更多查看译文
关键词
local entropy, histogram deconvolution, particle image velocimeter
AI 理解论文
溯源树
样例
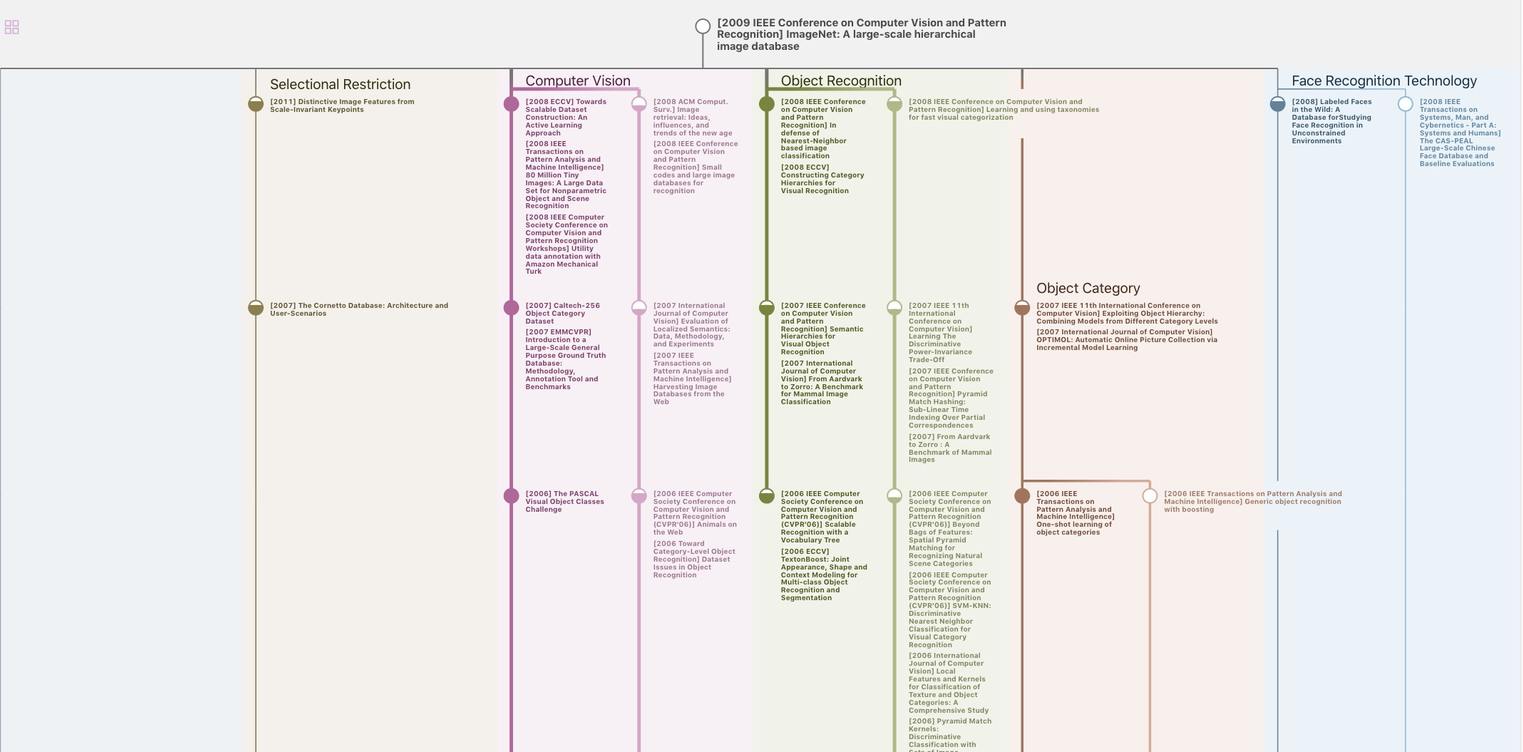
生成溯源树,研究论文发展脉络
Chat Paper
正在生成论文摘要