Identification of Outlying Observations with Quantile Regression for Censored Data
mag(2014)
摘要
Outlying observations, which significantly deviate from other measurements, may distort the conclusions of data analysis. Therefore, identifying outliers is one of the important problems that should be solved to obtain reliable results. While there are many statistical outlier detection algorithms and software programs for uncensored data, few are available for censored data. In this article, we propose three outlier detection algorithms based on censored quantile regression, two of which are modified versions of existing algorithms for uncensored or censored data, while the third is a newly developed algorithm to overcome the demerits of previous approaches. The performance of the three algorithms was investigated in simulation studies. In addition, real data from SEER database, which contains a variety of data sets related to various cancers, is illustrated to show the usefulness of our methodology. The algorithms are implemented into an R package OutlierDC which can be conveniently employed in the \proglang{R} environment and freely obtained from CRAN.
更多查看译文
AI 理解论文
溯源树
样例
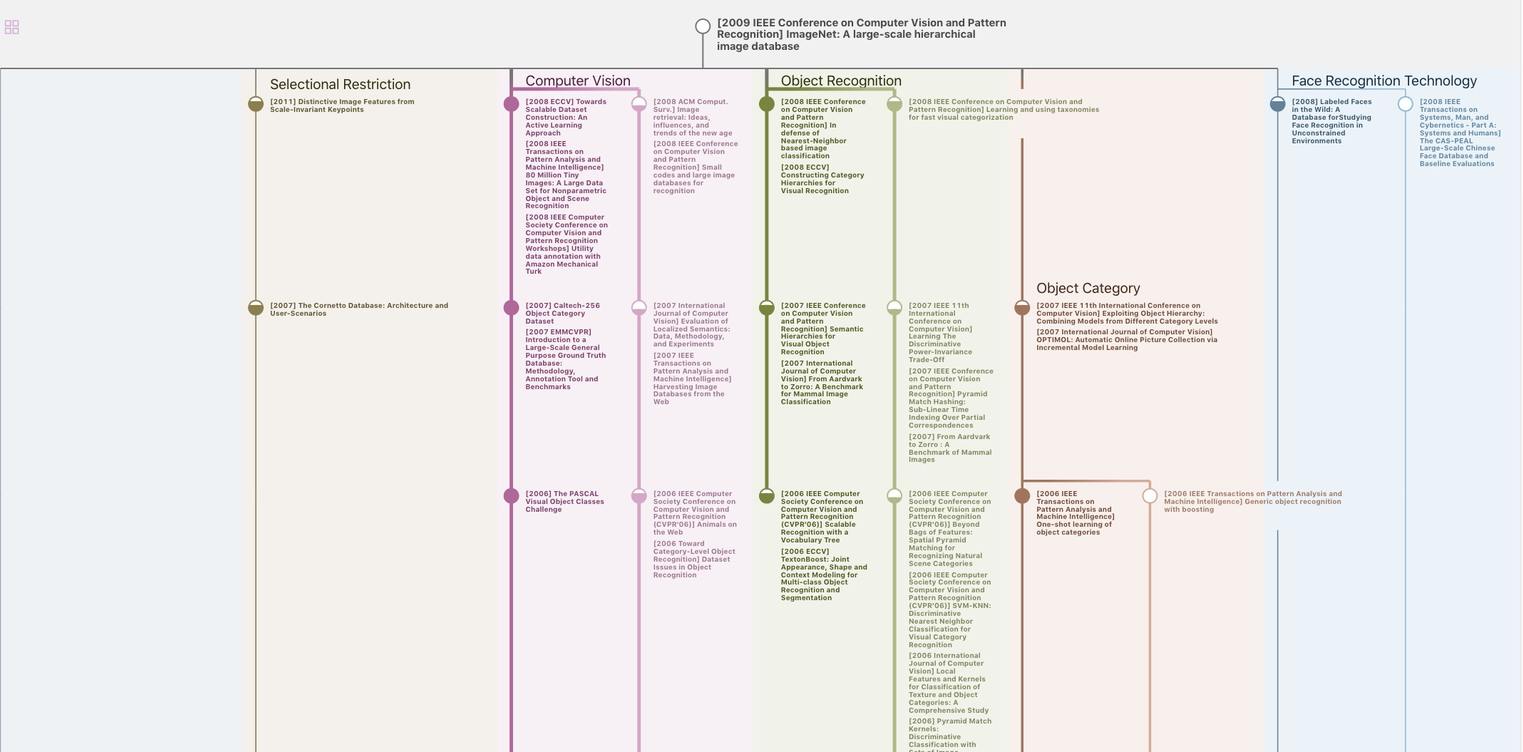
生成溯源树,研究论文发展脉络
Chat Paper
正在生成论文摘要