Automated segmentation of murine lung tumors in x-ray micro-CT images
Proceedings of SPIE(2014)
摘要
Recent years have seen micro-CT emerge as a means of providing imaging analysis in pre-clinical study, with in-vivo micro-CT having been shown to be particularly applicable to the examination of murine lung tumors. Despite this, existing studies have involved substantial human intervention during the image analysis process, with the use of fully-automated aids found to be almost non-existent. We present a new approach to automate the segmentation of murine lung tumors designed specifically for in-vivo micro-CT-based pre-clinical lung cancer studies that addresses the specific requirements of such study, as well as the limitations human-centric segmentation approaches experience when applied to such micro-CT data. Our approach consists of three distinct stages, and begins by utilizing edge enhancing and vessel enhancing non-linear anisotropic diffusion filters to extract anatomy masks (lung/vessel structure) in a pre-processing stage. Initial candidate detection is then performed through ROT reduction utilizing obtained masks and a two-step automated segmentation approach that aims to extract all disconnected objects within the ROT, and consists of Otsu thresholding, mathematical morphology and marker-driven watershed. False positive reduction is finally performed on initial candidates through random-forest-driven classification using the shape, intensity, and spatial features of candidates. We provide validation of our approach using data from an associated lung cancer study, showing favorable results both in terms of detection (sensitivity=86%, specificity=89%) and structural recovery (Dice Similarity=0.88) when compared against manual specialist annotation.
更多查看译文
关键词
biological image analysis,in-vivo micro-CT,micro-CT segmentation,murine lung tumours
AI 理解论文
溯源树
样例
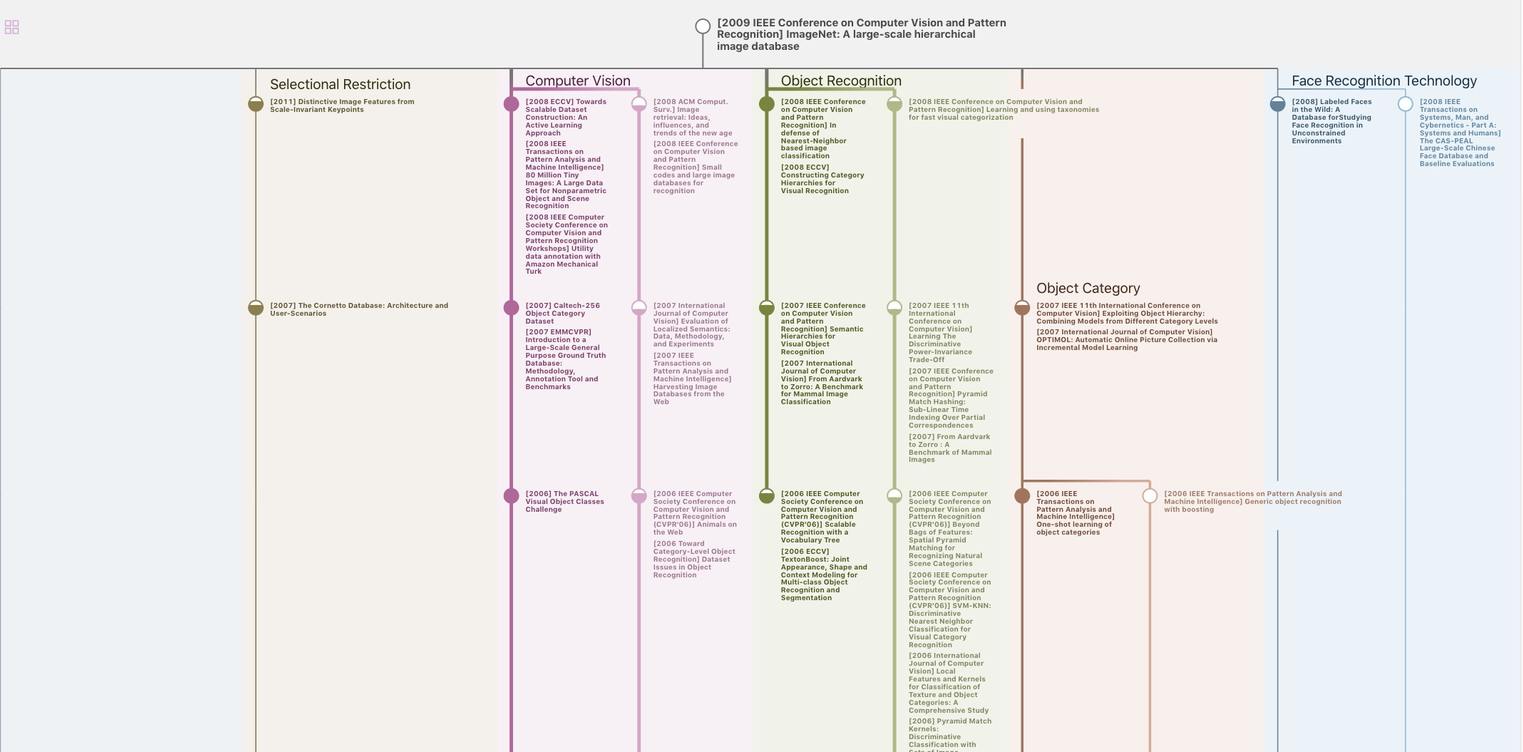
生成溯源树,研究论文发展脉络
Chat Paper
正在生成论文摘要