Data Dependent Priors In Pac-Bayes Bounds
COMPSTAT'2010: 19TH INTERNATIONAL CONFERENCE ON COMPUTATIONAL STATISTICS(2010)
摘要
One of the central aims of Statistical Learning Theory is the bounding of the test set performance of classifiers trained with i.i.d. data. For Support Vector Machines the tightest technique for assessing this so-called generalisation error is known as the PAC-Bayes theorem. The bound holds independently of the choice of prior, but better priors lead to sharper bounds. The priors leading to the tightest bounds to date are spherical Gaussian distributions whose means are determined from a separate subset of data. This paper gives another turn of the screw by introducing a further data dependence on the shape of the prior: the separate data set determines a direction along which the covariance matrix of the prior is stretched in order to sharpen the bound. In addition, we present a classification algorithm that aims at minimizing the bound as a design criterion and whose generalisation can be easily analysed in terms of the new bound.The experimental work includes a set of classification tasks preceded by a bound-driven model selection. These experiments illustrate how the new bound acting on the new classifier can be much tighter than the original PAC-Bayes Bound applied to an SVM, and lead to more accurate classifiers.
更多查看译文
关键词
PAC Bayes Bound, Support Vector Machines, generalization prediction, model selection
AI 理解论文
溯源树
样例
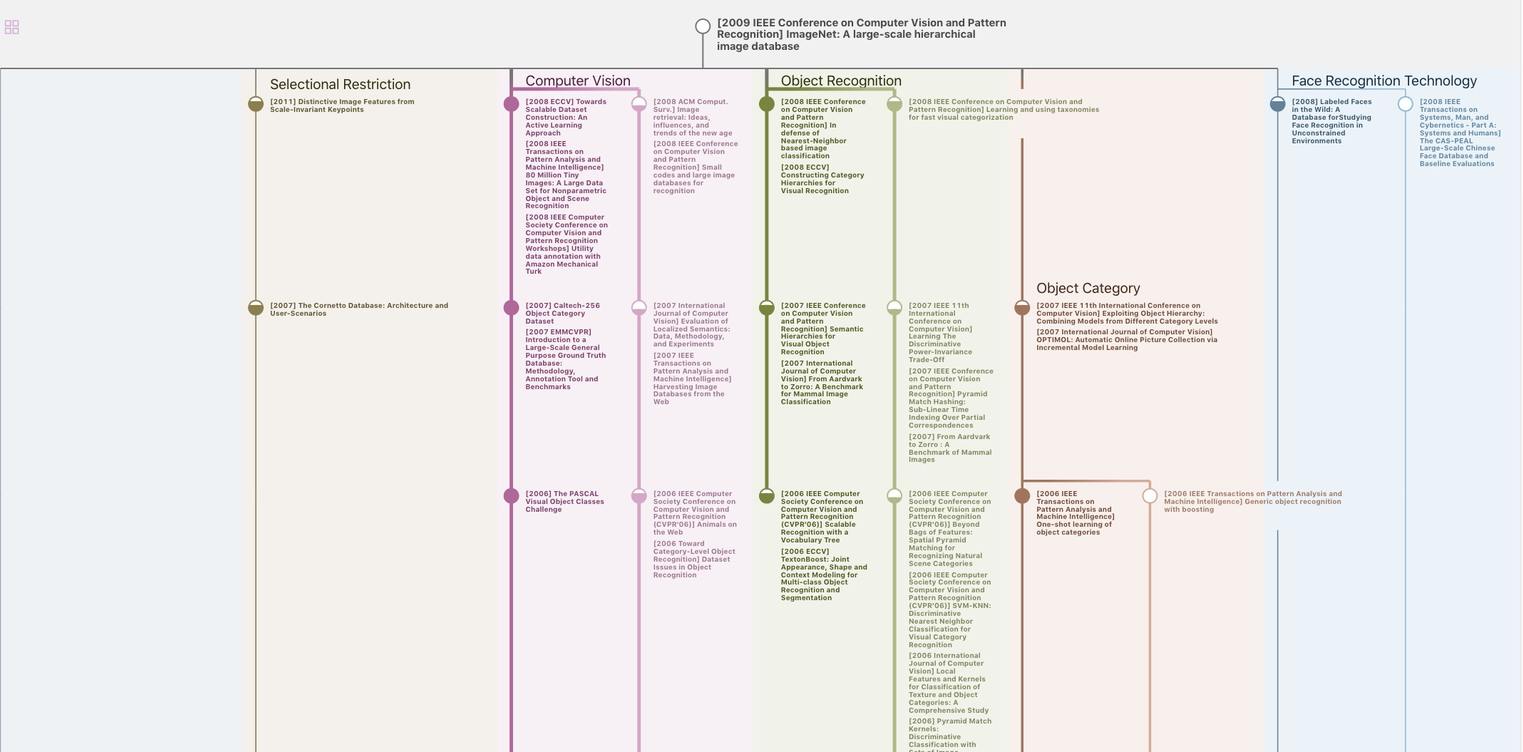
生成溯源树,研究论文发展脉络
Chat Paper
正在生成论文摘要