Lichen muqueux : à propos de 43 cas
Annales de Dermatologie et de Vénéréologie(2013)
摘要
The Soil and Water Assessment Tool (SWAT) and the Hydrologic Simulation Program-Fortran (HSPF) are two river basin simulation models with similar scheme of watershed delineation and functionalities. Both have been selected as modeling tools to support decision and policy making in management of the Illinois River Basin, the United States. This paper reports results calibrating and evaluating SWAT and HSPF model to hydrologic data in the Illinois River Basin, with relative performance of two models in hydrologic simulation and model behaviors under calibration being further compared. In this study, two different calibration approaches, the multi-criteria and the generalized likelihood uncertainty estimation (GLUE) method, were used to quantify uncertainties originated from the use of multi-site discharge observations and the presence of equifinal solutions. It is concluded that both models achieved satisfactory performance after the calibration, and the parameter identification of each model was subject to considerable uncertainties. Furthermore, there exist parameter sets that enable the HSPF model to generate more accurate predictions of the discharges in the main stem of the Illinois River than the SWAT model does, but when the two models were run in un-calibrated mode the distributions of the model fit summary statistics for HSPF observed in the Monte Carlo sampling during GLUE calibration are more varied than those for SWAT with heavier tails on the inferior side and SWAT would have comparable performance to HSPF on average. This finding implies that the accuracy that the HSPF model can achieve in a modeling exercise may have more reliance on the efficacy of the calibration procedure, and the application of SWAT may have some advantage when calibration data are lacking or scarce.
更多查看译文
关键词
muqueux
AI 理解论文
溯源树
样例
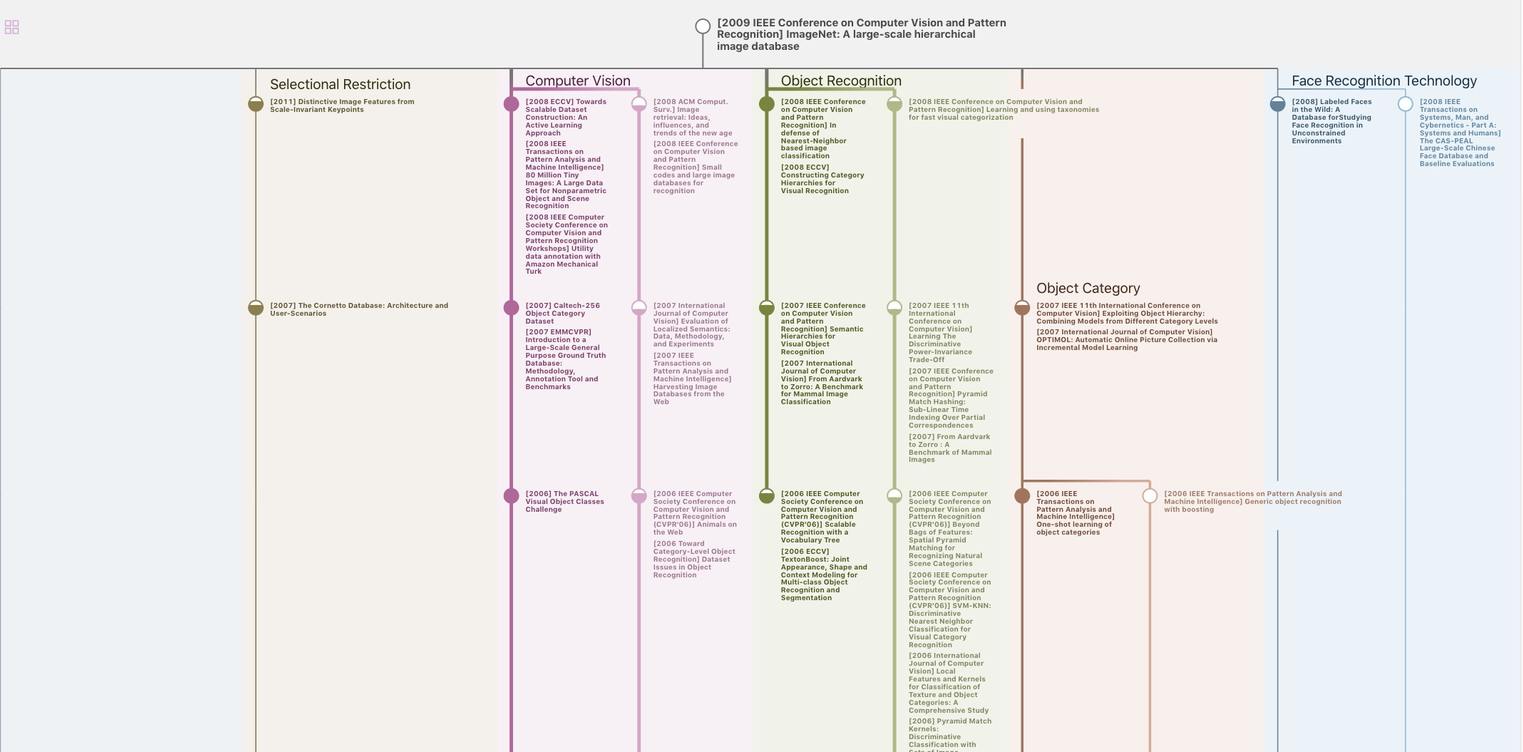
生成溯源树,研究论文发展脉络
Chat Paper
正在生成论文摘要