Improving The Accuracy Of Water And Bottom Properties Derived From Remote Sensing Reflectance Via Artificial Neural Network
COASTAL OCEAN REMOTE SENSING(2007)
摘要
Artificial neural network has been proven a useful technique for deriving water and bottom properties from remote sensing upwelling radiance. Conventionally, a neural network is trained to minimize the overall mean square error of desired products. The approach does not explicitly take into account the change of spectral shapes of upwelling radiance. In this study, we have created four groups of training sets, two groups with ratios of Rrs(lambda(i)) to Rrs(557), and the others without. Ratios of Rrs(lambda(i)) to Rrs(557) for lambda(i) of 409nm, 438nm, 488nm, 507nm, 616nm, 665nm, 683nm, 712nm, 750nm and 779nm have been used as additional inputs in the training of neural networks. Trained neural networks were then applied to an independent testing set which was created for optically different coastal waters. The inclusion of 10 spectral ratios in the training significantly improves the accuracy of derived water depth H, backscattering coefficient b(b)(438) and the absorption coefficient a(438). The accuracy of the derived coefficients is 86%, 94% and 92%. Our results clearly show the importance for including spectral ratios in the neural network training process. Remote sensing upwelling radiance over the identified 11 spectral channels provides adequate information for the retrieval of water optical property coefficients when an artificial neural network approach is used.
更多查看译文
关键词
water optical properties,artificial neural network
AI 理解论文
溯源树
样例
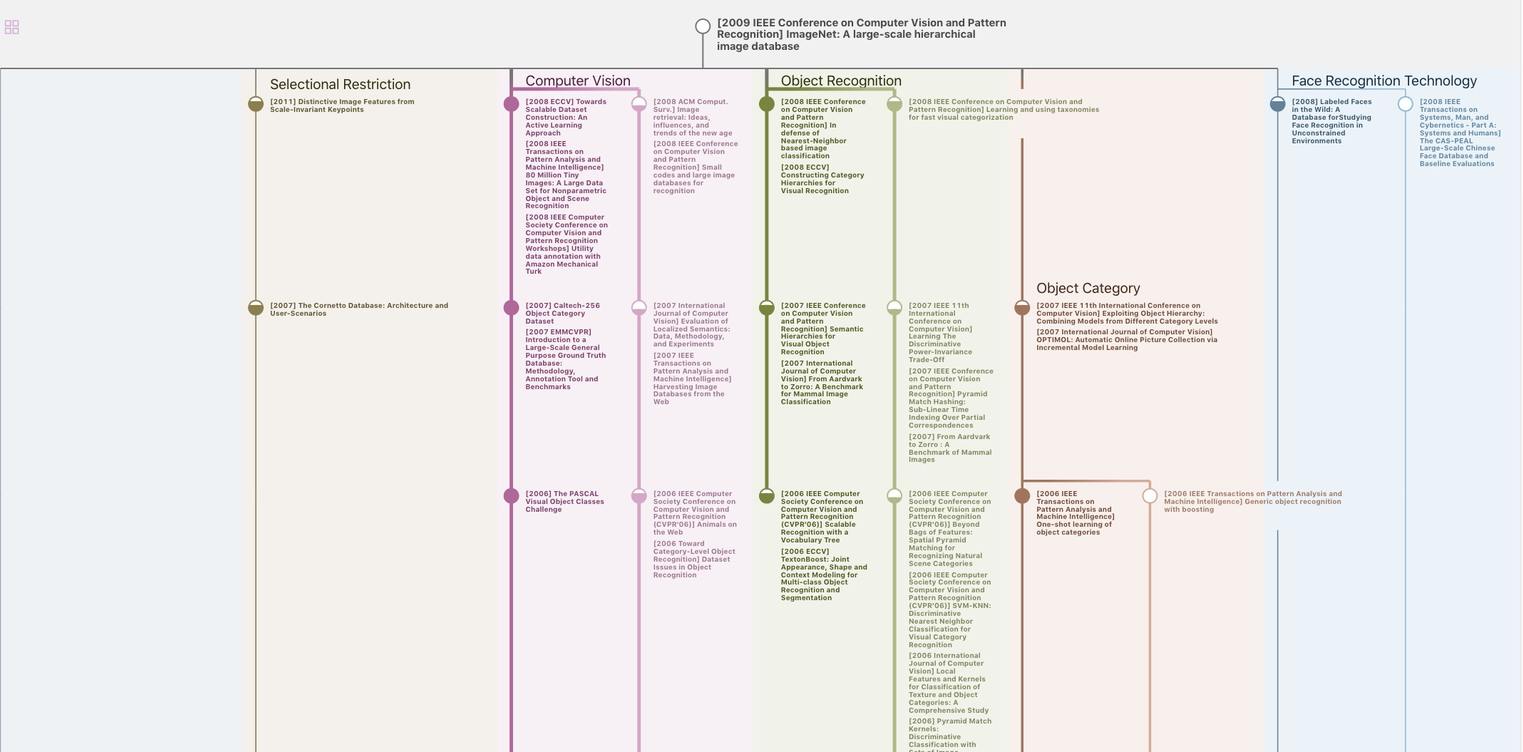
生成溯源树,研究论文发展脉络
Chat Paper
正在生成论文摘要