Particle filter-based Gaussian process optimisation for parameter inference
IFAC Proceedings Volumes(2014)
摘要
We propose a novel method for maximum likelihood-based parameter inference in nonlinear and/or non-Gaussian state space models. The method is an iterative procedure with three steps. At each iteration a particle filter is used to estimate the value of the log-likelihood function at the current parameter iterate. Using these log-likelihood estimates, a surrogate objective function is created by utilizing a Gaussian process model. Finally, we use a heuristic procedure to obtain a revised parameter iterate, providing an automatic trade-off between exploration and exploitation of the surrogate model. The method is profiled on two state space models with good performance both considering accuracy and computational cost.
更多查看译文
关键词
control engineering,signal processing
AI 理解论文
溯源树
样例
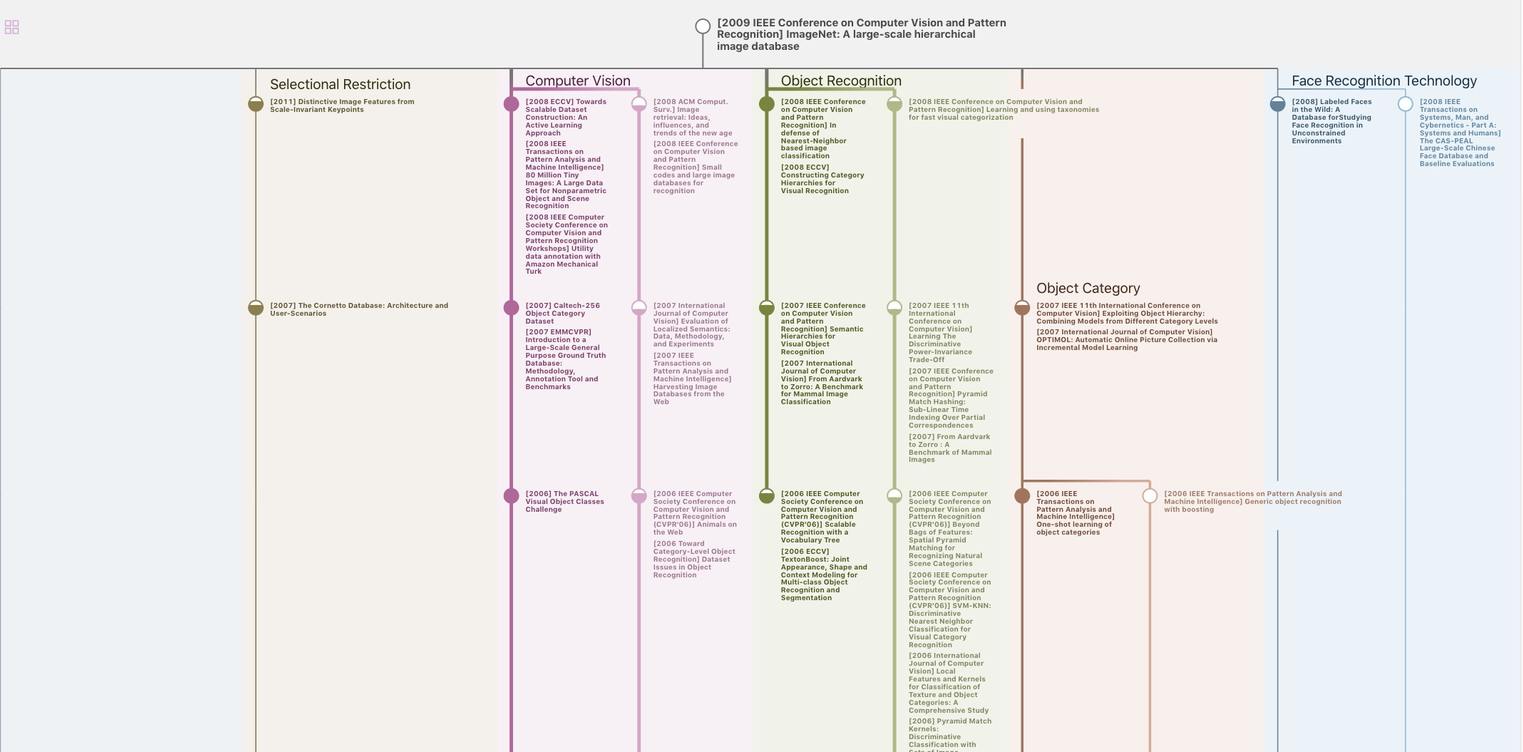
生成溯源树,研究论文发展脉络
Chat Paper
正在生成论文摘要