Classification of vegetation types in military region
Proceedings of SPIE(2015)
摘要
In decision-making process regarding planning and execution of military operations, the terrain is a determining factor. Aerial photographs are a source of vital information for the success of an operation in hostile region, namely when the cartographic information behind enemy lines is scarce or non-existent. The objective of present work is the development of a tool capable of processing aerial photos. The methodology implemented starts with feature extraction, followed by the application of an automatic selector of features. The next step, using the k-fold cross validation technique, estimates the input parameters for the following classifiers: Sparse Multinomial Logist Regression (SMLR), K Nearest Neighbor (KNN), Linear Classifier using Principal Component Expansion on the Joint Data (PCLDC) and Multi-Class Support Vector Machine (MSVM). These classifiers were used in two different studies with distinct objectives: discrimination of vegetation's density and identification of vegetation's main components. It was found that the best classifier on the first approach is the Sparse Logistic Multinomial Regression (SMLR). On the second approach, the implemented methodology applied to high resolution images showed that the better performance was achieved by KNN classifier and PCLDC. Comparing the two approaches there is a multiscale issue, in which for different resolutions, the best solution to the problem requires different classifiers and the extraction of different features.
更多查看译文
关键词
Classification,Feature Selector,Types of Vegetation
AI 理解论文
溯源树
样例
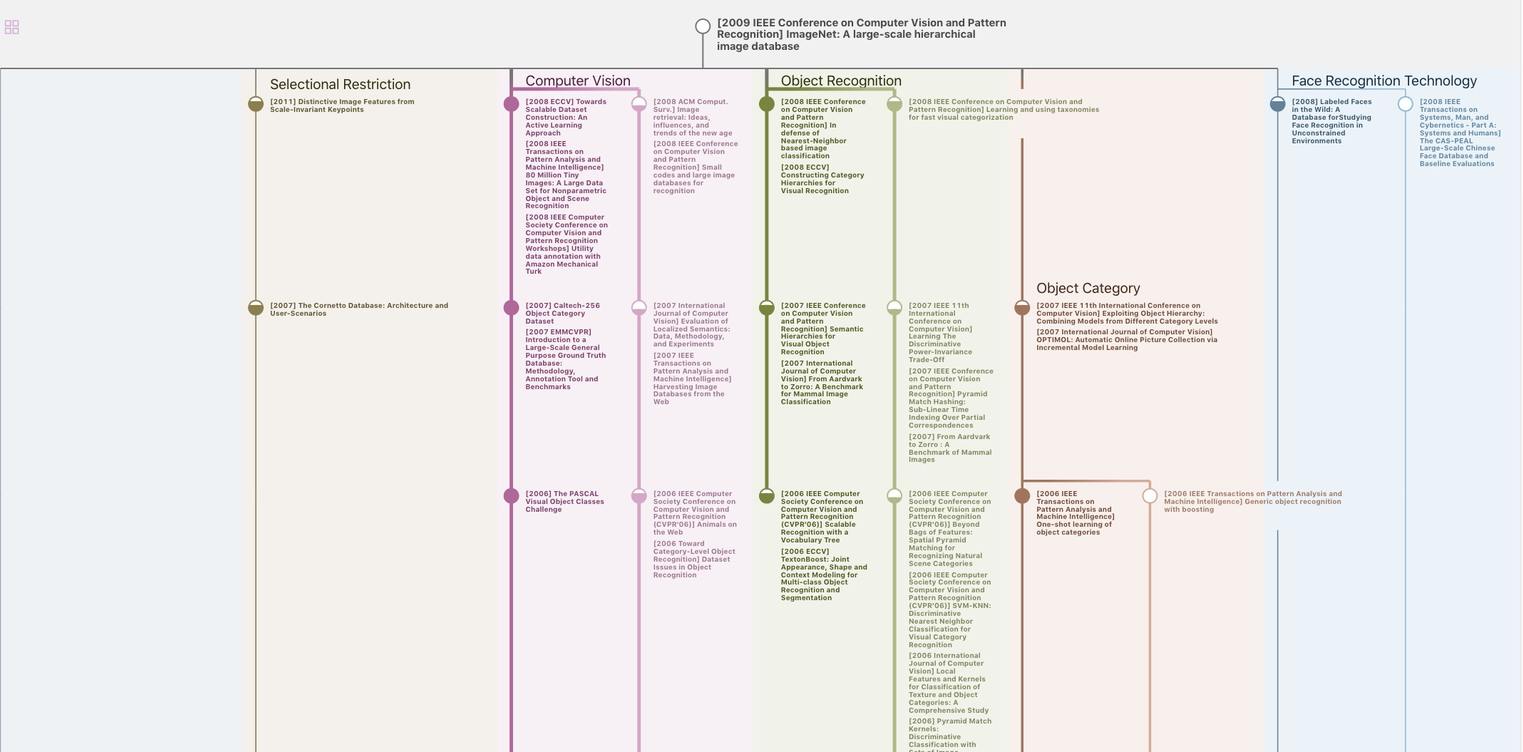
生成溯源树,研究论文发展脉络
Chat Paper
正在生成论文摘要