Non-destructive testing of cracks using eddy-currents and a generalized regression neural network (GRNN)
IEEE Antennas and Propagation Society International Symposium. Digest. Held in conjunction with: USNC/CNC/URSI North American Radio Sci. Meeting (Cat. No.03CH37450)(2003)
摘要
In this paper, we propose a new method for the robust estimation of crack dimensions. The method is based on the eddy current evaluation and a generalized regression neural network (GRNN) scheme. The network is trained by several known crack shapes based on the input impedance of a magnetic probe using a finite element solution for the eddy currents. The target value to be trained was the shape of the crack using a window based on the probe impedance. Noisy data, added to the probe measurements, is used to enhance the robustness of the method. We present a comparison of the results obtained using the proposed method with those obtained from a feed-forward neural network. It is shown that the GRNN is faster both in training as well as in identification of the cracks.
更多查看译文
关键词
radial basis neural networks,crack nondestructive testing,eddy current testing,generalized regression neural network,GRNN,crack dimensions estimation,network crack shape training,magnetic probe input impedance,finite element methods,feed-forward neural network,crack identification
AI 理解论文
溯源树
样例
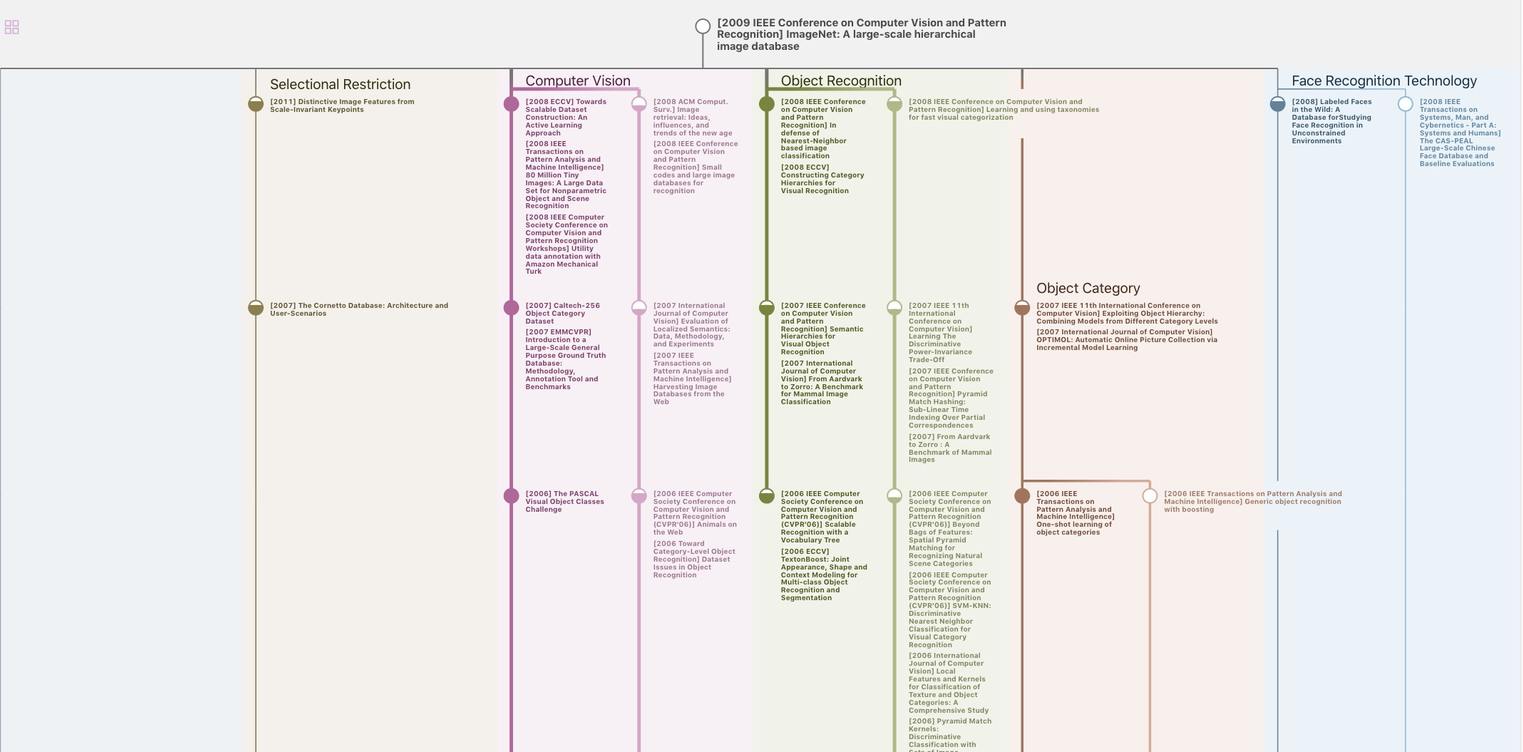
生成溯源树,研究论文发展脉络
Chat Paper
正在生成论文摘要