Error Rate Analysis of Labeling by Crowdsourcing
international conference on machine learning(2013)
摘要
Crowdsourcing label generation has been a crucial component for many real-world machine learning applications. In this paper, we provide finite-sample exponential bounds on the error rate (in probability and in expectation) of hyperplane binary labeling rules for the Dawid-Skene (and Symmetric DawidSkene ) crowdsourcing model. The bounds can be applied to analyze many commonly used prediction methods, including the majority voting, weighted majority voting and maximum a posteriori (MAP) rules. These bound results can be used to control the error rate and design better algorithms. In particular, under the Symmetric Dawid-Skene model we use simulation to demonstrate that the data-driven EM-MAP rule is a good approximation to the oracle MAP rule which approximately optimizes our upper bound on the mean error rate for any hyperplane binary labeling rule. Meanwhile, the average error rate of the EM-MAP rule is bounded well by the upper bound on the mean error rate of the oracle MAP rule in the simulation.
更多查看译文
AI 理解论文
溯源树
样例
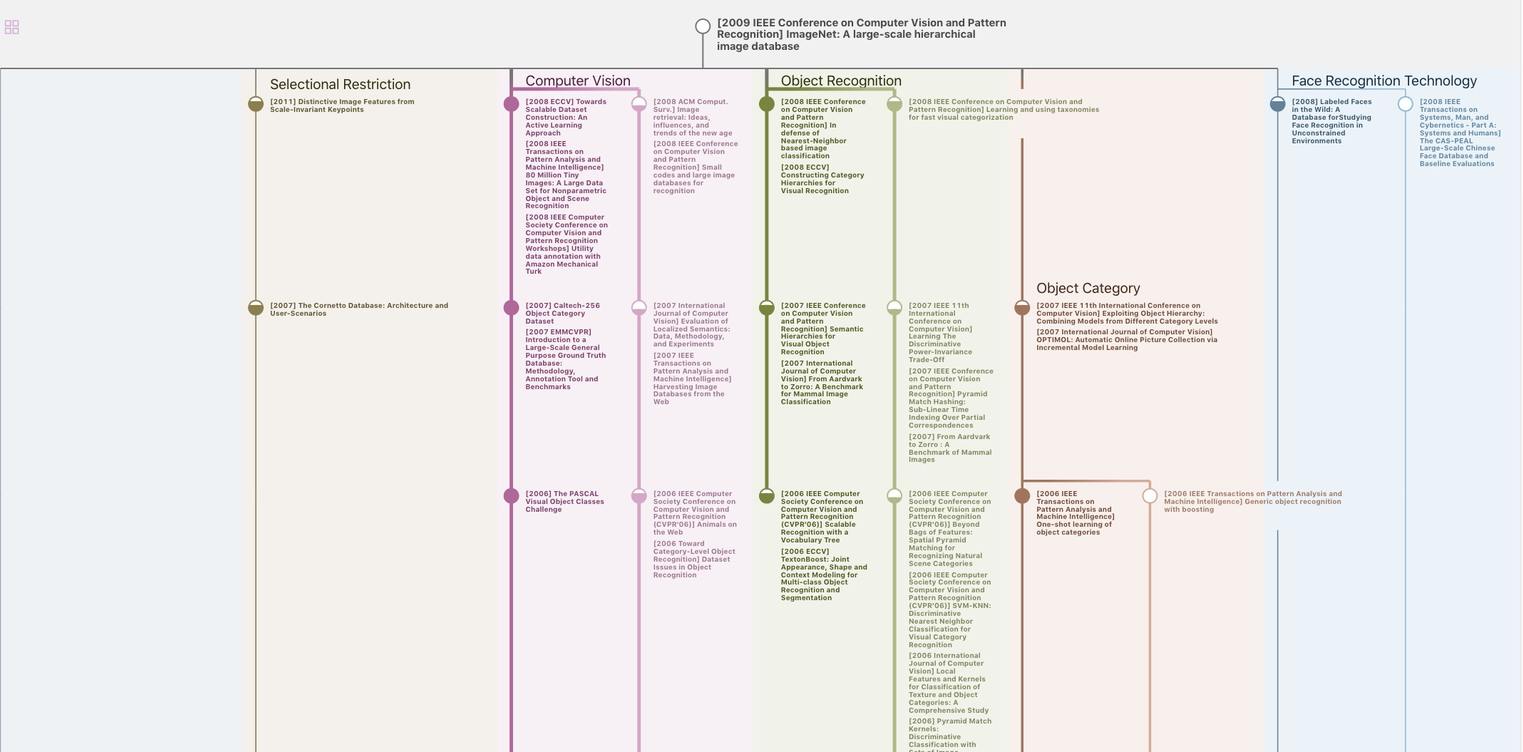
生成溯源树,研究论文发展脉络
Chat Paper
正在生成论文摘要