Particle Swarm Optimization on Low Dimensional Pose Manifolds for Monocular Human Pose Estimation
Proceedings of SPIE, the International Society for Optical Engineering/Proceedings of SPIE(2013)
摘要
Automatic assessment of situations with modern security and surveillance systems requires sophisticated discrimination capabilities. Therefore, action recognition, e. g. in terms of person-person or person-object interactions, is an essential core component of any surveillance system. A subclass of recent action recognition approaches are based on space time volumes, which are generated from trajectories of multiple anatomical landmarks like hands or shoulders. A general prerequisite of these methods is the robust estimation of the body pose, i.e. a simplified body model consisting of several anatomical landmarks.In this paper we address the problem of estimating 3D poses from monocular person image sequences. The first stage of our algorithm is the localization of body parts in the 2D image. For this, a part based object detection method is used, which in previous work has been shown to provide a sufficient basis for person detection and landmark estimation in a single step. The output of this processing step is a probability distribution for each landmark and image indicating possible locations of this landmark in image coordinates. The second stage of our algorithm searches for 3D pose estimates that best fit to the 15 landmark probability distributions. For resolving ambiguities introduced by uncertainty in the locations of the landmarks, we perform an optimization within a Particle Swarm Optimization (PSO) framework, where each pose hypothesis is represented by a particle. Since the search in the high-dimensional 3D pose search space needs further guidance to deal with the inherently restricted 2D input information, we propose a new compact representation of motion sequences provided by motion capture databases. Poses of a motion sequence are embedded in a low-dimensional manifold. We represent each motion sequence by a compact representation - referred to as pose splines - using a small number of supporting point poses. The PSO algorithm can be extended to perform the optimization process directly on pose splines. Results of the proposed method are shown on the UMPM benchmark.
更多查看译文
关键词
Scene understanding,pose estimation,particle swarm optimization,pose manifold representation
AI 理解论文
溯源树
样例
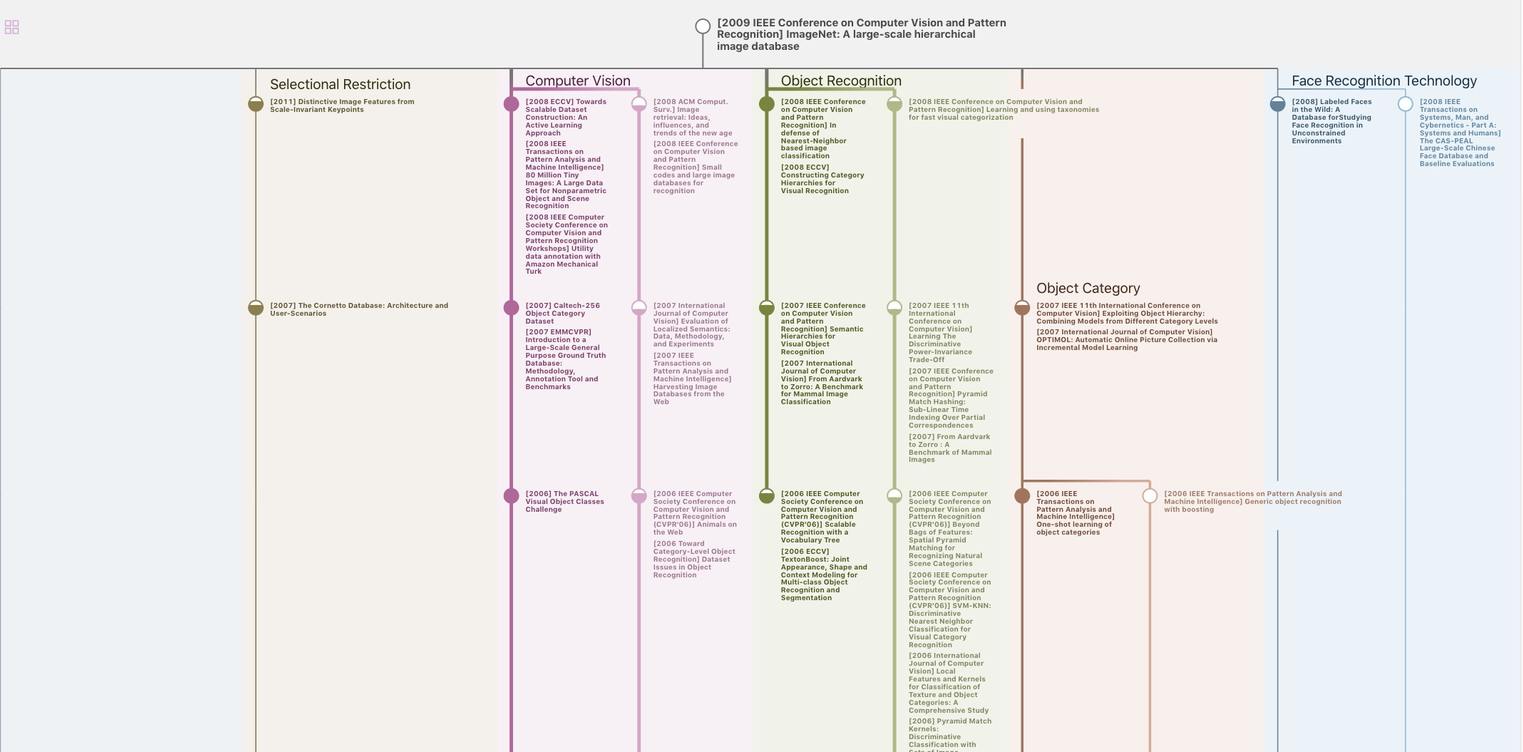
生成溯源树,研究论文发展脉络
Chat Paper
正在生成论文摘要