Analysis of Personal Life Patterns Using Accelerometer-Based Wearable Devices
International Journal of Automation and Smart Technology(2015)
摘要
Many commercially available wearable devices are equipped with sensors to measure motion and physiological signals from the wearer. G-sensors are commonly used in such wearable devices for counting steps, estimating energy expenditure and detecting sleep duration. In this study, two features derived from G-sensor motion signals, average cadence (step count divided by time) and ratio of high G value (outside the range of 0.5g~1.5g), were used to classify physical activities into four intensity levels (sedentary, light, moderate, hard). Eighty physical activity samples were collected and trained by the Weka machine learning software to form a classification model. G-sensor motion signals from four participants were collected over two weeks and classified into four activity intensity levels using the model. Physical activity levels (PAL) and personal life patterns of the participants were then derived. This data can then be used to tailor additional services for individual users of wearable devices. A BLE (Bluetooth Low Energy) based system for older adults with dementia, combining personal life pattern analysis with localization function, is also proposed as an example application.
更多查看译文
关键词
machine learning
AI 理解论文
溯源树
样例
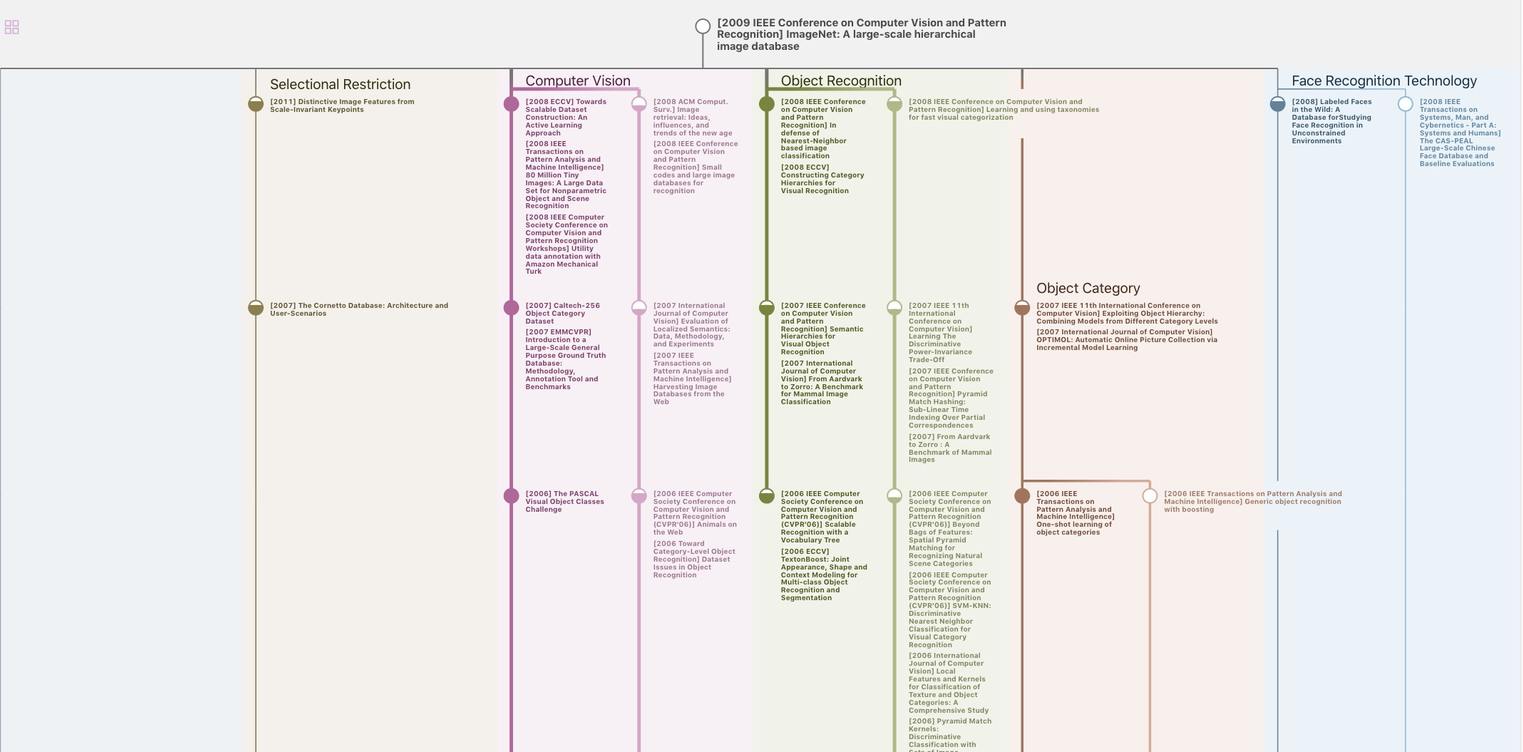
生成溯源树,研究论文发展脉络
Chat Paper
正在生成论文摘要